Household Displacement and Return in Disasters: A Review
Abstract
Household displacement following disasters has become endemic in many areas worldwide, affecting at least 265 million people between 2008 and 2018. Although this figure includes short-term and potentially life-saving evacuations, there is ample evidence that not all households return after the emergency phase. Protracted displacement is associated with particularly negative consequences for the affected households and community. Yet, existing data on displacement duration are limited, and only a few disaster recovery models incorporate the multitude of factors beyond housing damage that are known to influence household return. This review synthesizes the current literature on disaster-induced displacement, including key terminology and context, the determinants of household return decisions, existing model-based approaches, and opportunities for future research. The identified key determinants of household return can be broadly organized into the following categories: physical damage to the built environment, psychological and social phenomena (e.g., attachment to place, social networks), household demographics (e.g., tenure, socioeconomic status), and pre- and postdisaster policies (e.g., housing reconstruction approach, design of aid programs).
Introduction
At least 265 million people were displaced due to sudden-onset disasters (e.g., earthquakes, tropical cyclones) between 2008 and 2018, which amounts to nearly one person per second (IDMC 2019). The number of people displaced by disasters is projected to increase, driven by poorly managed urban growth in hazard-prone areas (IDMC 2017) and the effects of climate change (IPCC 2012). This scale of human impact has prompted international attention, such as within the 2015–2030 Sendai Framework for Disaster Risk Reduction, which stresses the severe consequences of displacement, including disrupted family and community life, destroyed livelihoods, interrupted education, increase in poverty, health and safety concerns, and reduced access to basic services (UNDRR 2019).
Despite this massive scale of human impact, disaster risk assessments have historically focused on direct economic losses due to physical damage. This metric tends to highlight the wealthiest as the most at risk because they have the most economic value to lose. This outcome is discordant with observations from past disaster events, which consistently demonstrate that poor and marginalized groups are disproportionately affected (Hallegatte et al. 2020; Fothergill and Peek 2004). Mitigation strategies selected primarily based on economic losses may inadvertently deepen existing inequalities, highlighting a need for more people-centered and equitable risk metrics to inform disaster mitigation (Markhvida et al. 2020; Cremen et al. 2023). Displacement could serve as a more equitable risk metric that better captures the human toll of disasters, but consideration within disaster risk assessments has been limited.
Most attempts to incorporate population displacement within disaster risk and recovery models focus on the effects of physical damage to the built environment, which primarily inform initial displacement and emergency shelter needs. Factors beyond damage to the built environment, such as psychological and social phenomena (e.g., place attachment, social capital and networks, perceived risk), demographic factors (e.g., socioeconomic status, housing and land tenure, age, race/ethnicity/caste), and policy decisions (e.g., housing reconstruction approach, design of aid programs), are rarely considered within community recovery models despite widespread evidence of their significance on displacement duration and household return following past disaster events.
This review synthesizes existing research on disaster-induced displacement, including key definitions and contextual factors, determinants of return decisions, existing models of household displacement, and opportunities for future research. The findings from this review can aid an enhanced understanding of household displacement in disasters and guide future efforts to quantify the phenomenon within disaster recovery models.
Scope and Definitions
Disaster researchers have highlighted inconsistent use of terminology regarding population displacement following disasters (Esnard and Sapat 2014; Mitchell et al. 2012; Greer 2015). To address this, the definitions adopted herein are defined in this section based on a synthesis of the reviewed literature.
The Internal Displacement Monitoring Centre (IDMC) defines displacement as “involuntary or forced movements … of individuals or groups of people from their habitual places of residence” (IDMC 2020). These movements might be triggered by disasters, conflict or violence, or other factors such as development. This review focuses on displacement triggered by sudden-onset hazards (i.e., events that emerge quickly), as indicated in Fig. 1.
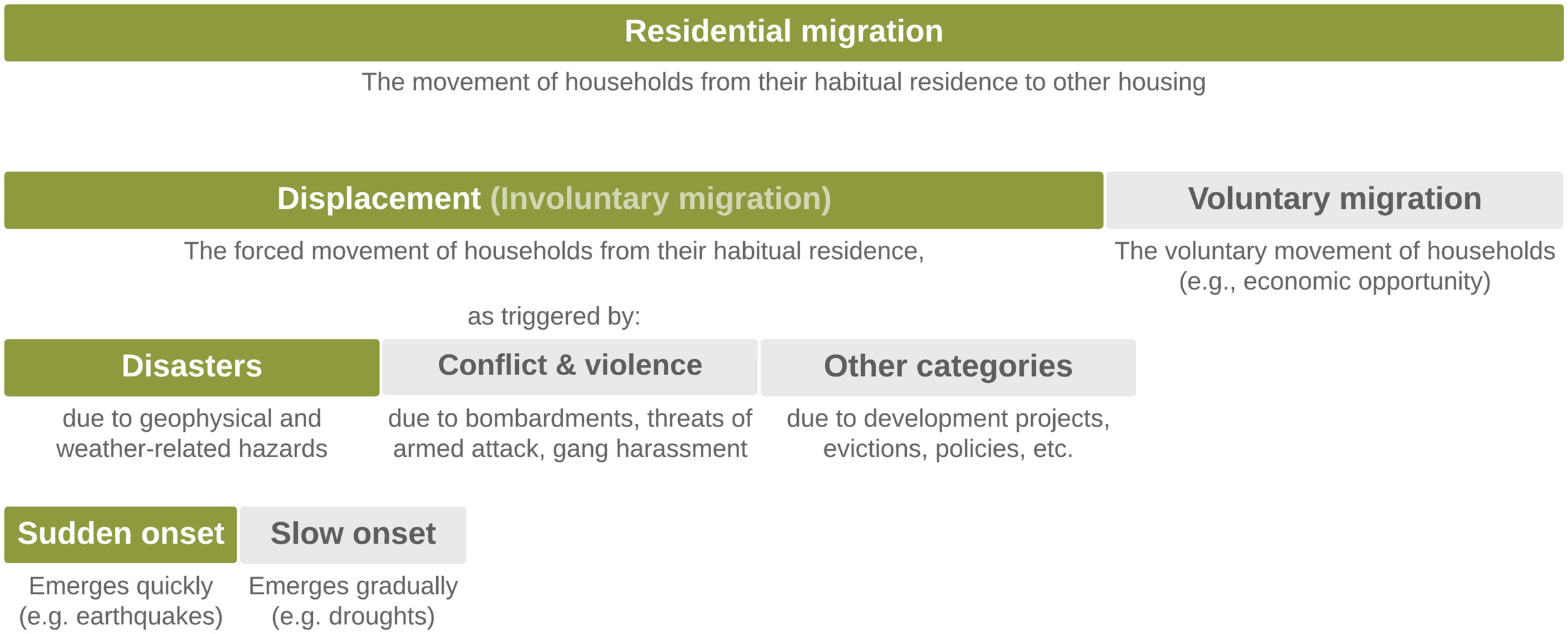
Importance of Duration
Notably, IDMC considers multiple movements under the umbrella of disaster-induced displacement, including evacuations and relocations (IDMC 2020; McAdam 2022). This means displacement can be both a risk with negative consequences for those affected and a successful life-saving risk reduction strategy (IOM and IDMC 2022). Therefore, the duration of displacement is essential to understand the human impact, as depicted in Fig. 2. Although large-scale evacuations can be followed by mass return shortly afterward, physical damage and disrupted livelihoods can lead to protracted displacement for a subset of the population. Protracted displacement is associated with particularly negative consequences such as job loss and income decline (e.g., Hori and Schafer 2010; Takahashi et al. 2016; Zissimopoulos and Karoly 2010), disruption of education and its long-term effects on children (Picou and Marshall 2007; Hamideh et al. 2022), increased post-traumatic stress (Blaze and Shwalb 2009; Hansel et al. 2013), and other psychosocial effects (e.g., strained family relationships, sleep disturbances, anxieties) (Bolin 1982).

Movements Associated with Displacement
This review adopts the following definitions of the key movements associated with disaster-induced displacement.
•
Evacuations: The movement of individuals or households away from the impacted area (Perry and Lindell 2003; Esnard and Sapat 2014), either preemptively in the early warning phase or during hazard onset and the emergency phase (Johnson et al. 2020). Although evacuations are usually intended to be short term and followed by a return (Xu et al. 2020), not all evacuees successfully return (Smith and McCarty 1996; Peacock et al. 2018). Evacuations may be encouraged by an evacuation order or can occur without such orders (i.e., shadow evacuations) (Gerber 2010; Dash and Gladwin 2007).
•
Dislocations: The movement of individuals or households after the hazard event occurs (Lin 2009), including in the emergency and recovery phases. In contrast to evacuations’ preventive or protective nature, dislocations are primarily driven by physical damage (e.g., housing and infrastructure damage) and potentially exacerbated by other factors (e.g., weather, job loss, socioeconomic characteristics) (Lin 2009).
•
Relocations: The movement of individuals or households from one residence to another. Individuals and households that relocate in the context of disasters often do so multiple times (Smith and McCarty 1996; Pardee 2012; Sutley and Hamideh 2020) and have been described as a “group in transit” (Morrow-Jones and Morrow-Jones 1991).
•
Returns: The movement of individuals or households back to their habitual residence or community of origin. Ideally, this movement would reflect a commitment and an aim to reestablish normal routines in that original location. However, such a movement does not guarantee a durable solution, because some households may return to uninhabitable housing due to a lack of viable alternatives (IDMC 2020).
•
Resettlements: The movement of an individual or household to a new location or community, reflecting a commitment to that new location and an aim to reestablish normal routines (Greer 2015). Individuals or households that have resettled have out-migrated from their community of origin. Although this review distinguishes “resettlement” from “relocation,” it is noted that many empirical and analytical studies of disaster recovery use both terms interchangeably and without clear distinction.
Population Metrics Associated with Displacement
This review adopts the following definitions of key population metrics associated with disaster-induced displacement.
•
Sheltered: People accommodated in public shelters, which may be provided by national authorities, nongovernmental organizations, or international organizations (IDMC 2020).
•
Rendered homeless: People without adequate shelter due to the effects of a disaster (IDMC 2020), such as uninhabitable or destroyed housing.
Stages of Sheltering and Housing
Immediately before or after a hazard event, individuals and households may evacuate their homes and seek shelter, such as with family and friends, in hotels or rentals, or at public shelters. As time passes, these households may continue to relocate and transition through various stages of sheltering and housing, as proposed by Quarantelli (1982, 1995). These stages are further reviewed by Peacock et al. (2018) and extended by Sutley and Hamideh (2020). The descriptions of each stage are illustrated in Fig. 3 and further described as follows:
•
Emergency sheltering: Sheltering in the immediate time before or after the hazard event for a short duration (i.e., a few hours to overnight) (Quarantelli 1982, 1995) and characterized by spontaneity, the immediacy of need, and locational convenience (Tierney et al. 2001; Alexander 2002; Bolin 1993).
•
Temporary sheltering: Sheltering in places where affected households can stay for longer periods before temporary or permanent housing becomes available. Daily necessities (e.g., food, water, beds, security) are provided here. However, these areas are not intended to replace housing, and those sheltered rarely attempt to reestablish their daily routines here (Quarantelli 1982, 1995).
•
Temporary housing: Housing is distinct from sheltering in that it allows daily routines to be reestablished. Households within temporary housing await permanent housing, whether that is a return to their previous homes or an alternative solution (Quarantelli 1982, 1995). The duration can vary from weeks to months or years. However, temporary housing may sometimes inadvertently become permanent housing (Quarantelli 1982, 1995; Barakat 2003) or be intentionally designed to transition into permanent housing (Barakat 2003; Sphere Project 2011).
•
Permanent housing: A return to the previous residence or resettlement (i.e., permanent relocation) to alternative stable housing where households can continue their daily routines (Quarantelli 1982, 1995; Sutley and Hamideh 2020).
•
Failure of the housing recovery system: An outcome of homelessness, languishing in uninhabitable housing, or death (Sutley and Hamideh 2020). This stage recognizes that some households may not achieve permanent housing (Fothergill and Peek 2015).

The proposed stages of sheltering and housing are idealized and not necessarily linear or continuous; some stages can overlap or be skipped, and some households can become stuck at or even regress between stages (Quarantelli 1982, 1995; Peacock et al. 2018; Fothergill and Peek 2015; Mukherji 2017). Moreover, the timelines between transitions are not uniform across all population subgroups (Quarantelli 1995; Sutley and Hamideh 2020; Lines et al. 2022; Taheri Tafti and Bashiri 2022).
A distinction is made between sheltering and housing, where the critical difference is that housing enables the resumption of household activities, domestic functions, and daily routines. This distinction is also evident in paired terminology used within disaster recovery literature, such as “shelter” and “livelihoods” (Corsellis and Vitale 2005; Nabong et al. 2021; Pu et al. 2021) or “habitability” (Chang et al. 2008) and either “usability” (Cavalieri et al. 2012) or “livability” (Scheele et al. 2020). A key takeaway is that household recovery is not fully defined by the inhabitance of a specific dwelling type (e.g., public shelter, prefabricated unit, trailer, owner-occupied home); instead, household recovery is a process that encompasses a broader set of needs and preferences as determined by each affected household separately (Sanderson et al. 2014).
Factors Influencing the Return of Displaced Households
Disasters are life events that can subject households to key migration decision points (Chang et al. 2008). The relative weight of influencing factors may vary by context (e.g., currently evacuated versus already returned). Although the initial evacuation or dislocation is often a forced movement, return decisions are more voluntary, albeit constrained by realities such as housing availability, moving costs, and proximity to livelihoods. Some common household decision points within the context of disasters are illustrated in Fig. 4.

This section focuses on the decisions to return. For a review of household evacuation decisions, see Thompson et al. (2017) and Dash and Gladwin (2007). For a review of household decisions to stay or resettle, see Greer et al. (2019). For a discussion of household decisions on shelter choice, see Lee and Chen (2018).
Prevalent determinants of household return decisions are described individually herein, although these factors are often highly linked (Henry 2013; Esnard and Sapat 2014) and intersecting (Rufat 2013; Morrow 1999). Moreover, the determinants of return decisions can differ by population subgroup (e.g., renters versus owners, city dwellers versus rural inhabitants) (Henry 2013; Burton et al. 2019) and by decision choice (i.e., return versus relocate) (Henry 2013; Kim and Soo Oh 2014). For example, Henry (2013) found that homeowners after Hurricane Katrina revolved their decisions to return or relocate around housing, but only as it related to factors such as family, work, insurance, and perceived or acceptable risk. In contrast, renters were less motivated by housing matters and more influenced by work, education, and family.
Available quantitative studies focused on household return decisions after disasters were reviewed, with a summary of the top influencing factors shown in Fig. 5 and the full Table S2 provided in the Supplemental Materials. Fig. 5 indicates the frequency of the top influencing factors in two ways: across all study instances (i.e., data sample or subset presented within a given study) and across only instances that considered that factor. The former may be biased toward factors that have been heavily considered within studies, whereas factors considered in fewer studies may be more prone to extreme values in the latter case. Different metrics are used to quantify similar phenomena across some studies, but these factors are presented separately. For example, attachment to place is often measured through proxies such as tenure time or hometown status. Similarly, housing damage can be measured using property damage losses as a proxy. To help organize the results, each factor was grouped into the following categories: housing matters, financial aspects, social and community aspects, and demographics. The factors that most consistently influenced return decisions in the reviewed quantitative studies include housing damage, tenure, neighborhood damage level, and family and relationships. The following subsections elaborate on these and some additional factors, organized into the following categories: physical damage to the built environment, psychological and social phenomena, household demographics, and pre- and postdisaster policies.
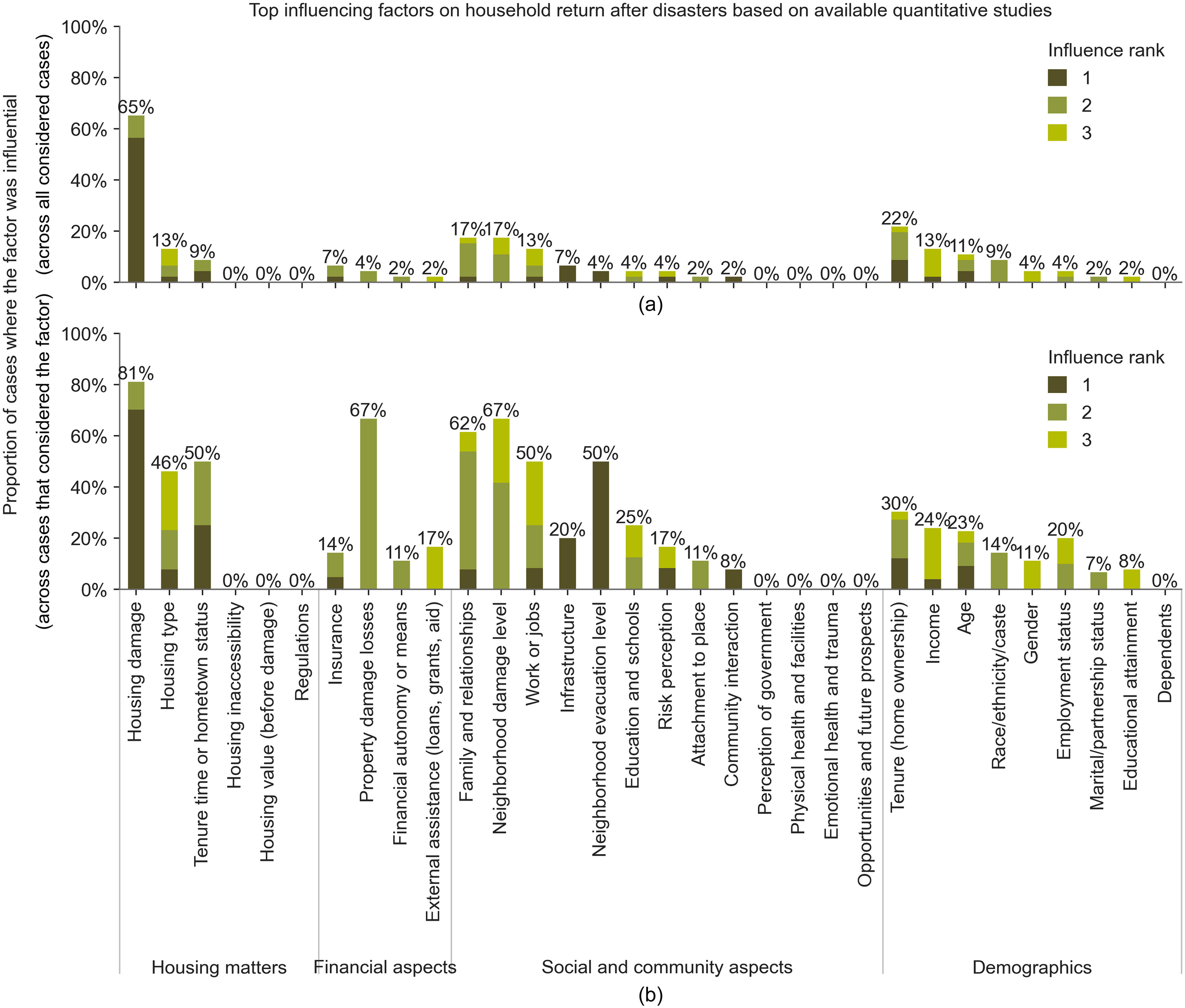
Physical Damage to the Built Environment
Physical damage to the built environment has consistently been a primary driver of displacement following disasters (Smith and McCarty 1996; Scheele et al. 2020; Burton et al. 2019; Abramson et al. 2015; Gray et al. 2009; Xu et al. 2020; Mayer et al. 2020; Cong et al. 2018; Abuhamdia 2018; Yabe et al. 2020; Girard and Peacock 1997; Groen and Polivka 2010). Building damage, utility loss, infrastructure damage, and weather can render homes uninhabitable, thereby displacing residents (Chang 2016; Khazai et al. 2012; Wright and Johnston 2010; Chien et al. 2002; Chang et al. 2008). This displacement may become protracted when housing remains uninhabitable or inaccessible during the recovery phase, as buildings and utilities undergo significant repairs within the larger community and safety cordons may be put in place. As displacement becomes protracted, households may become less likely to return, an effect that can snowball when simultaneously affecting many households in the community (Storr and Haeffele-Balch 2012; Sapat and Esnard 2016).
Despite evidence indicating that aggregate housing recovery targets can be met within 2 to 3 years (Hirayama 2000; Comerio 1998; SPUR 2012), differential trajectories exist by damage level and housing type (Comerio 1998; SPUR 2012; Peacock et al. 2014; Zhang and Peacock 2009; Hamideh et al. 2021; Comerio and Blecher 2010; Lu et al. 2007). Housing units damaged to a higher degree take longer to recover because repairing severe damage or demolition and replacement are complex and time intensive. Moreover, such repairs are more costly, and thus households may require more time to procure the necessary financing. Regarding housing type, single-family owner-occupied homes have consistently been observed to recover faster than multifamily or rental properties, even after controlling for the level of damage (Lu et al. 2007; Hamideh et al. 2021). Multifamily residences may have to contend with complex ownership structures and require time to build a consensus on recovery decisions (Wu and Lindell 2004; Johnson and Olshansky 2016), whereas decisions to maintain, repair, or rebuild rental housing rely upon sufficient economic incentive for landlords (Comerio 1998). Policy decisions and the availability of financing impact the recovery trajectories of both multifamily and rental housing, because past postdisaster policies have tended to be designed for and favor single-family owner-occupied homes, such as has been observed following past disaster events in the United States, India, and Iran (Comerio 1997; Mukherji 2017; Taheri Tafti and Tomlinson 2013). This phenomenon is described further in the section “Pre- and Postdisaster Policies.”
Aside from concerns with the habitability of residences, damage to businesses (Xiao and Van Zandt 2012; Miles and Chang 2011), workplaces (Comerio 1997), services (e.g., public transport, healthcare) (Comerio 1997), or other community assets (e.g., schools, churches) (Nejat et al. 2019; Airriess et al. 2008) may further discourage return. For example, the loss of jobs or destruction of livelihoods can limit the ability of displaced households to return and recover (Elliott and Pais 2006; Pu et al. 2021). In addition, community assets such as schools may encourage households to return in the interest of educational continuity, but closed schools can inhibit return and contribute to a sense of “nothing to go back to” (Peek et al. 2011).
Psychological and Social Phenomena
Beyond physical damage, various psychological and social phenomena have been found to influence household decisions to return after disasters. These factors include conditions in the origin and host communities, place attachment, social capital, and perceived risk.
Disasters have been known to accelerate ongoing trends (Kates et al. 2006; Haas et al. 1977). Similarly, the predisaster community conditions or postdisaster functioning can prompt displaced households to act on previous desires or plans, thereby quickening existing migration trends (Belcher and Bates 1983; Nawrotzki et al. 2014). However, separating disaster-specific patterns from long-term patterns is a measurement challenge (Chang 2010). In addition, there have been limited attempts to separate disaster-specific migration from general migration in case studies of previous events (Gray et al. 2009; Nawrotzki et al. 2014).
Place attachment, the strong affective bond people have to their environments (Hidalgo and Hernández 2001; Scannell and Gifford 2010), has been identified as a determinant of return decisions (Bonaiuto et al. 2016; Morrice 2013; Chamlee-Wright and Storr 2009). However, destruction from disaster events can also cause emotional distress after return that modulates the level of place attachment and can affect decisions to stay (Morrice 2013; Miller and Rivera 2007). Place identity (an individual’s perception of their own identity relative to their environment) and place dependence (an individual’s perception of their community’s ability to address their needs) have been identified as two dimensions of place attachment that are correlated but distinct (Williams and Vaske 2003). However, studies on the influence of place attachment are limited by the difficulty of quantifying the phenomena; although surveys can be designed to assess the level of place attachment and its dimensions directly (Xu et al. 2020), other approaches have used tenure duration, hometown status, or neighborhood satisfaction as a proxy (Nejat and Ghosh 2016; Costa et al. 2022b; Lee et al. 2017).
Social capital has also been identified as an influential factor in the return decisions of displaced households (Lee et al. 2017; Henry 2013). Social capital has been described as “resources embedded in one’s social networks … that can be accessed or mobilized through ties in the networks” (Lin 2008, p. 51; Aldrich 2011). Different types of social capital include bonding (within networks), bridging (between networks), and linking (formal collaboration with institutions) (Aldrich and Meyer 2015; Nakagawa and Shaw 2004; Woolcock 2002). As an example of bonding social capital, Nejat et al. (2016) found that those who lived with family members before Hurricane Sandy in 2012 in Staten Island, New York were less likely to relocate after the disaster than those who lived alone (although the effect was not observed in those with alienated family relationships). Following the 1995 Kobe earthquake in Japan, Aldrich (2011) found that wards with higher social capital (measured as the number of new nonprofit and community-based organizations per capita) had higher population growth rates. Additionally, collective action has been observed, whereby individual reconstruction and return decisions were influenced by neighbors’ decisions (Nejat and Damnjanovic 2012; Financial Services Roundtable 2006).
Although the perceived risk of future disasters has been consistently identified as a driver of evacuation decisions (Thompson et al. 2017; Dash and Gladwin 2007), few studies investigate the role of perceived risk on return decisions (Henry 2013; Xu et al. 2020; Nawrotzki et al. 2014). In one such example, Xu et al. (2020) investigated households’ willingness to relocate following the Wenchuan and Lushan earthquakes in Sichuan, China, considering the perception of disaster severity and disaster possibility. Based on the survey data, Xu et al. (2020) found that disaster severity was significantly positively associated with households’ willingness to relocate.
Household Demographics
Beyond physical damage, outcomes and recovery trajectories appear to be stratified across demographic lines (Elliott and Pais 2006; Peacock et al. 2014; Wyczalkowski et al. 2019; Zhang and Peacock 2009), with poor and marginalized groups facing fewer options, more protracted or circuitous recovery pathways, and worse sheltering and housing outcomes (Spoon et al. 2021; Gray et al. 2009; Hirayama 2000; Cole 2003). As such, a social vulnerability lens is often applied to disaster research to better capture how historical injustices, social inequalities, and cultural biases shape the susceptibility of various groups to harm and limit their ability to respond or cope with disasters (Cutter et al. 2003; Blaikie et al. 2014). Demographic factors influencing housing recovery and population return include socioeconomic status, ownership status, age, and race/ethnicity/caste.
Populations of lower socioeconomic status tend to inhabit hazard-prone areas, reside in lower-quality homes, and are less likely to have access to risk reduction measures such as structural strengthening or insurance (Winsemius et al. 2018; Hallegatte et al. 2020; Tierney et al. 2001; Fothergill and Peek 2004; Bolin and Stanford 1991). These factors can lead poor and marginalized groups to experience more significant damage in the event of a disaster, increasing the likelihood of displacement and delaying return. Beyond the direct consequences of damage, those with lower socioeconomic status are also more likely to face obstacles in the recovery phase, including difficulties accessing assistance (Comerio 2014; Dash et al. 1997; Greene 1992; Peacock et al. 2018) and fewer resources to abate the effects of displacement (e.g., to afford temporary accommodation or absorb rent increases).
Homeownership status is a consistent determinant of household return (Kim and Soo Oh 2014; Mayer et al. 2020; Cong et al. 2018; Lee et al. 2017; Elliott and Pais 2006). Explanations for the faster return of single-family homeowners include place attachment (Henry 2013; Binder et al. 2015), lack of options due to mortgage obligations (Elliott and Pais 2006), and the bias of governmental aid toward homeowners (Comerio 1997; Mukherji 2017; Taheri Tafti and Tomlinson 2013). Moreover, renters may encounter more obstacles to finding permanent housing because they have limited control over recovery decisions on the property they inhabit, have to grapple with limited availability of affordable rental units and possible postdisaster rental price increases if unable to enter their buildings (Comerio 1998; Mukherji 2014; Pardee 2012), and may be evicted by opportunistic landlords seeking to raise rents (Pardee 2012). In parallel, landlords may lack economic incentive to repair the buildings and keep their original tenants (Hirayama 2000; Taheri Tafti and Tomlinson 2013; Mukherji 2010). Aside from home and land ownership, a lack of the associated ownership documentation can limit access to public assistance, described further in the following section. For a more detailed review of the role of housing tenure, see Lee and Van Zandt (2019).
Concerning age, older individuals have been observed to be more likely to return following disasters (Groen and Polivka 2010; Hu et al. 2019; Cong et al. 2018). They may be more motivated to return to recoup their social supports (e.g., family, community resources, friends, and physicians) (Sanders et al. 2004). At the same time, the elderly are more likely to live in low-quality housing and experience higher relative loss due to limited financial means (Ngo 2001; Bolin and Klenow 1988), which may inhibit their ability to return.
Findings concerning race, ethnicity, and caste have been mixed concerning return and housing recovery following disasters. Studies investigating the delayed return of Black residents to New Orleans following Hurricane Katrina in 2005 found that the racial discrepancies were primarily driven by differential housing damage and not necessarily by race (Fussell et al. 2010; Groen and Polivka 2010). On the other hand, discriminatory practices can limit options for alternative accommodation (Girard and Peacock 1997; Taheri Tafti and Bashiri 2022), and access to assistance may be limited by distrust in the government, poor language skills, or lack of political and social capital (Aldrich 2010; Bolin and Stanford 1998; Phillips 1993; Prater and Lindell 2000; Mukherji 2010; Taheri Tafti and Tomlinson 2013).
Pre- and Postdisaster Policies
Postdisaster recovery policies shape the nature of both housing recovery and population return. Financing can be disbursed to homeowners for the repair or replacement of their buildings, cash assistance or rental subsidies can provide relief for renters, targets can be set for the provision or construction of temporary and permanent housing, and new standards can be established for where and how to build (e.g., no-build zones, building code enforcement). However, disasters do not occur in a vacuum—preexisting policies have already influenced housing supply and vacancy rates, the affordability of housing and rent prices, the quality and maintenance of the housing stock, and the extent of homelessness and informality.
Comerio (1998) proposed four key models for housing reconstruction, as given in Table 1. Two principal axes of housing reconstruction have also been proposed: the level of government involvement (Comerio 2014; Johnson and Olshansky 2016; Mukherji 2017) and the level of community participation in housing recovery (Comerio 2014; Johnson and Olshansky 2016).
Model | Description | Examples |
---|---|---|
Redevelopment | The national government takes a strong role, featuring large-scale housing reconstruction programs | Turkey following the 1999 Marmara earthquake |
China after the 1976 Tangshan earthquake | ||
The Soviet Union after the 1988 Spitak (Armenia) earthquake | ||
Capital infusion | An injection of public or external funds or resources filters through governmental and nongovernmental agencies that implement housing reconstruction programs | Haiti following the 2010 earthquake |
Indonesia after the 2004 Indian Ocean earthquake and tsunami | ||
Iran after the 2003 Bam earthquake | ||
India after the 2001 Gujarat earthquake | ||
Limited intervention | Similar to the market model, but includes insurance and limited governmental assistance in the form of grants and loans to households and businesses | The United States after hurricanes in the late 20th and 21st centuries |
Japan after the 1995 Kobe earthquake | ||
Market | Primarily relies on the real estate market and nongovernmental organizations to address housing recovery issues | — |
Although housing reconstruction is critical, it does not guarantee that the original residents return nor that displaced households achieve similar quality, affordability, and proximity of housing conditions to their predisaster state (Taheri Tafti and Tomlinson 2013). Public assistance has typically been skewed toward owner-driven housing reconstruction rather than affordable housing options for renters, who comprise a substantial portion of the urban population (Mukherji 2010, 2017; Freeman 2004; Comerio 1998). As such, disaster researchers have advocated for needs-based assistance and a broad-based housing recovery approach (Bolin and Stanford 1998; Kamel and Loukaitou-Sideris 2004; Howell and Elliott 2019; Mukherji 2017; Taheri Tafti and Bashiri 2022). Examples of broader assistance include rental subsidies, temporary or public housing provisions, economic incentives for rental property owners, and homeownership programs (Mukherji 2015).
The design of aid eligibility requirements, disbursement time, and funding amounts also influence households’ recovery trajectories and can hinder housing options for subsets of displaced households. Concerning eligibility requirements, aid disbursement often requires the provision of documents (e.g., tenure title, utility bills) that can be lost in the disaster or nonexistent in the first place (Mitchell et al. 2017; Spoon et al. 2021; Taheri Tafti and Tomlinson 2013), low-income residents may struggle to qualify for loans due to poor credit or low repayment potential (Kamel and Loukaitou-Sideris 2004; Comerio 1997), and stipulations that cash or rental assistance must be spent on housing reconstruction can prevent households with more immediate needs from receiving future installments to which they would otherwise be entitled (Mukherji 2018; Taheri Tafti and Tomlinson 2015; Sutley and Hamideh 2020). In addition, lengthy disbursement times create uncertainty for affected households and can delay decision-making or the transition to permanent housing (Finch et al. 2010; Emrich et al. 2022; Alisjahbana et al. 2022; Sou and Howarth 2023), particularly for those households that cannot rely on their personal resources to accommodate their recovery needs.
Household Displacement and Return in Disaster Recovery Models
This section focuses on displacement models that extend into the recovery phase rather than evacuation and shelter choice. An evaluation of models for evacuation behavior in hurricanes is available in Anyidoho et al. (2022), and an evaluation of models for shelter needs following earthquakes is available in Vecere et al. (2017). Displacement duration following disasters depends critically on housing recovery and the community context, such as socioeconomic characteristics, infrastructure and services, and resource constraints (Comerio 2006; Costa et al. 2021; Sutley et al. 2019). For a comprehensive overview of various community and housing recovery methods, see Miles et al. (2019). This review focuses specifically on the integration of population displacement estimates within these models. A summary of the key models discussed herein is shown in Fig. 6.

FEMA proposed a population displacement model in HAZUS-MH (FEMA 2003), primarily based on research following Hurricane Hugo, the Loma Prieta earthquake, and the Northridge earthquake (Lin 2009). The number of uninhabitable buildings is estimated by building type (i.e., single family or multifamily) and damage level (i.e., complete for single family and moderate for multifamily). Lin (2009) proposed two alternate approaches: the first a modified version of the HAZUS methodology (i.e., using different damage state probabilities and aggregation units) and the second an algorithmic approach incorporating social vulnerability factors (i.e., race/ethnicity) based on empirical evidence following Hurricane Andrew in 1992 in Miami-Dade County, Florida. The algorithmic approach uses a logistic regression model that estimates the probability that residents of a given structure will dislocate based on the percent loss of the structure (due to damage), housing type, and the percent of both Black and Hispanic subpopulations in the respective block group. The output of these models represents a dislocated population, but does not explicitly include a duration component nor does it capture successive population movements such as returns, relocations, and resettlements.
Regression models based on survey data have commonly been applied to identify determinants of household decisions to return or relocate (Nejat and Ghosh 2016; Kim and Soo Oh 2014; Burton et al. 2019; Cong et al. 2018; Lee et al. 2017; Gray et al. 2009; Elliott and Pais 2006; Abuhamdia 2018; Nawrotzki et al. 2014; Girard and Peacock 1997; Groen and Polivka 2010). Burton et al. (2019) used such a model to directly link community recovery model variables (i.e., housing damage, utility disruption, neighborhood evacuation level, duration of building inaccessibility) and household characteristics (income, tenure, insurance access) to household decisions to return or resettle (with or without repairs). The integration of the proposed household decision-making module within the disaster recovery model was shown to have a significant impact on the population recovery trajectories for their case study of a hypothetical earthquake in Los Angeles, emphasizing the importance of such decisions on community and household recovery. This model captures displacement duration by tracking population return (versus resettlement), but does not explicitly capture the transition of individual households through various stages of sheltering and temporary housing.
Costa et al. (2022a) proposed a comprehensive model of population displacement following earthquakes, differentiating between temporary displacement (based on structural damage, availability of water and power, and socioeconomic demographics) and permanent relocation (based on housing recovery speed, neighborhood recovery status, the recovery decisions of neighbors, a predisposition to move out, and psychological trauma). This model adopts an agent-based approach, which has previously been advocated to capture the heterogeneity and interactions across individual agents in household migration studies (Husby and Koks 2017). This initial model was later extended to integrate place attachment (Costa et al. 2022b) and to link the repair of rental units with landlord decisions (Mongold et al. 2022). Model outputs include population recovery trajectories, typically aggregated at the neighborhood level, although the approach could theoretically be applied at the household level if such detailed data were available. This framework captures the duration of displacement by tracking population return, but also explicitly differentiates dislocation (temporary displacement) versus resettlements (permanent displacement). Similar to the Burton et al. (2019) model, the Costa et al. (2022a) approach does not explicitly track household movements between stages of sheltering and temporary housing.
Similarly, Bhattacharya and Kato (2021) adopted an agent-based model approach to capture household decisions to return or relocate after a disaster, accounting for place attachment level, option level (e.g., financial standing and insurance access), and infrastructure requirements as primary household characteristics. Secondary household characteristics (i.e., elderly with or without pension; young with or without children) allow different household archetypes to prioritize different place attributes (e.g., employment, schools, hospital), influencing their perception of the disaster area’s attractiveness. Each household agent has a tolerance threshold considering the cost, waiting time, attractiveness, and (in the case of a high attachment) distance from home. Their framework allows the simulation of various recovery policy options, such as the provision of temporary housing, target infrastructure service levels over time, and the spatial location of facilities. In contrast to the other models described herein, this approach explicitly tracks relocations into temporary housing.
Grinberger and Felsenstein (2016) also employed agent-based models to capture urban dynamics and disaster recovery, incorporating household relocation decisions. Households decide to leave their residence based on utility-maximizing behavior (income relative to residential prices) and constraints (i.e., lack of space and affordability). Residential prices vary depending on the floor area and the average housing price in the neighborhood, which dynamically varies with demand, supply, and the level of community services available. Additionally, interaction with commercial buildings is considered as household agents decide to visit nonresidential buildings; without sufficient traffic, commercial buildings become unoccupied. The framework allows evaluating population recovery over time, depending on policy choices such as land-use regulations, provision of shelters, and restoration of damaged public services. Similar to most of the other models described herein, the focus is on returns and resettlements (i.e., the permanent housing stage).
Research Gaps and Opportunities
Although household displacement and return is a growing research area, several vital gaps and research opportunities remain. Despite the significance of displacement duration on the affected households and the community, few studies explicitly capture this temporal component (Sutley and Hamideh 2020; Smith and McCarty 1996; Fussell et al. 2010). Household displacement duration can be integrated within existing community recovery models that explicitly account for a temporal component (Costa et al. 2021; Miles and Chang 2011; Burton et al. 2016) but require validation that is limited by the lack of authoritative time-series data from past disaster events. Moreover, existing estimates of displaced persons after disasters are typically derived using housing damage estimates (IDMC 2020), posing a validation challenge because most household displacement models rely on similar assumptions. Mobile location data (e.g., call detail records, smartphone GPS data) could serve as a valuable data proxy for displacement that explicitly captures the time and space component (Lu et al. 2012; Bengtsson et al. 2011; Yabe et al. 2019, 2020; Wilson et al. 2016), but additional research is required to benchmark past events across geographies and hazard types to understand the data’s representativeness and applicability. The suitability of housing and community recovery models also requires review across different contexts because most applications have been to high-income communities that may have unique policy contexts (e.g., market-driven, limited public assistance) and determinants of household decision-making.
Furthermore, observations from past events indicate challenges with the recovery of affordable rental housing in urban areas (Hirayama 2000; Taheri et al. 2013; Mukherji 2010), whereas explicit consideration of multifamily and rental housing is largely missing from community and housing recovery models (Miles et al. 2019; Mongold et al. 2022). Surveys and regression models have been applied to homeowner and renter decisions to return or relocate/resettle following a disaster. Yet, similar work may be required to capture landlord decisions regarding repair or abandonment. Until rental and multifamily housing are incorporated, community and housing recovery models may have a limited capacity to evaluate policy decisions that encourage broad-based recovery (e.g., rental assistance and cash vouchers, incentives for rental property owners). Moreover, these models often use housing as the sole vehicle for displacement and return. Still, livelihood factors (e.g., job loss) and related policies (e.g., postdisaster employment insurance, livelihood assistance) may also have a significant impact.
Another challenge within community recovery models is the correlation of factors that influence both social and physical vulnerability. Synthetic population distribution is often employed in disaster risk models to populate buildings with people and their relevant characteristics, typically using aggregate demographic or census data. Microdata (e.g., individual or household-level responses to statistical surveys) may help realistically capture the evident correlation of some of these characteristics (e.g., income level and housing quality) that have been observed in past disaster events (Rubinyi et al. 2022).
Last, given the conflation and inconsistency of common terminology under the scope of disaster-induced displacement, future research should aim to define the adopted definitions clearly and indicate the setting of displacement within the disaster timeline (e.g., in the emergency phase, in the recovery phase).
Conclusions
Despite the scale and prevalence of household displacement following disasters and the toll of protracted displacement on affected households and communities, quantification of displacement primarily remains limited to the emergency phase in existing disaster risk models. This review aimed to synthesize the knowledge from existing studies and proposed models to focus future research and improve model-based approaches to quantify disaster-induced displacement.
From a review of the existing literature, it is clear that different factors influence household evacuation in the preparedness or emergency phase versus return in the recovery phase. There is consensus that physical factors (e.g., housing damage, utility disruption, neighborhood damage) are critical during the emergency phase. However, social and psychological factors such as place attachment and social capital become more important in the recovery phase as displaced households decide whether or not to return. Furthermore, return outcomes and recovery trajectories frequently appear stratified across demographic lines such as income level and ownership status. These differential patterns of household recovery are contributed to by predisaster conditions (e.g., concentrations of socially vulnerable populations in hazard-prone areas) and postdisaster policy decisions (e.g., the design of government assistance programs). As such, aggregate metrics cannot represent all households and often obscure significant differentials across specific population subgroups (Bolin 1985; Hirayama 2000; Hamideh et al. 2021; Peacock et al. 2018).
Various approaches have been taken to integrate household displacement and return into disaster recovery models. While physical damage to the built environment has long been considered a driver of household displacement, more recent models incorporate additional factors such as financial autonomy, household demographics, and attachment to place. Although significant improvements have been made, there are ongoing challenges in modeling household displacement and return in disasters. Some of these research opportunities include model validation and review across different contexts, robust incorporation of multifamily and rental housing, and consideration of the correlations between physical and social vulnerability factors.
Supplemental Materials
File (supplemental_materials_nhrefo.nheng-1930_paul.zip)
- Download
- 834.37 KB
Data Availability Statement
All data generated or analyzed during the study are included in the published paper.
Acknowledgments
This work was partly funded by the University College London Overseas Research Scholarship (ORS) and the Willis Towers Watson Research Network.
References
Abramson, D. M., D. Van Alst, A. Merdjanoff, R. Piltch-Loeb, J. Beedasy, P. Findley, and L. Ann Peek. 2015. The Hurricane Sandy place report: Evacuation decisions, housing issues and sense of community. New Brunswick, NJ: Rutgers Univ. School of Social Work. https://doi.org/10.7916/D82806TN.
Abuhamdia, R. Z. A. 2018. “The role of infrastructure in household relocation decision after disasters: A case study of relocation in Nepal after the 2015 earthquake.” Doctoral dissertation, Dept. of Civil and Environmental Engineering, Texas A&M Univ.
Airriess, C. A., W. Li, K. J. Leong, A. Chia-Chen Chen, and V. M. Keith. 2008. “Church-based social capital, networks and geographical scale: Katrina evacuation, relocation, and recovery in a New Orleans Vietnamese American community.” Geoforum Rethinking Econ. 39 (3): 1333–1346. https://doi.org/10.1016/j.geoforum.2007.11.003.
Aldrich, D. P. 2010. “Separate and unequal: Post-tsunami aid distribution in southern India.” Soc. Sci. Q. 91 (5): 1369–1389. https://doi.org/10.1111/j.1540-6237.2010.00736.x.
Aldrich, D. P. 2011. “The power of people: Social capital’s role in recovery from the 1995 Kobe earthquake.” Nat. Hazards 56 (3): 595–611. https://doi.org/10.1007/s11069-010-9577-7.
Aldrich, D. P., and M. A. Meyer. 2015. “Social capital and community resilience.” Am. Behav. Sci. 59 (2): 254–269. https://doi.org/10.1177/0002764214550299.
Alexander, D. E. 2002. Principles of emergency planning and management. Harpenden, UK: Terra.
Alisjahbana, I., A. Moura-Cook, R. Costa, and A. Kiremidjian. 2022. “An agent-based financing model for post-earthquake housing recovery: Quantifying recovery inequalities across income groups.” Earthquake Spectra 38 (2): 1254–1282. https://doi.org/10.1177/87552930211064319.
Anyidoho, P., R. Davidson, T. Rambha, and L. Nozick. 2022. “Prediction of population behavior in hurricane evacuations.” Transp. Res. Part A Policy Pract. 159 (May): 200–221. https://doi.org/10.1016/j.tra.2022.03.001.
Barakat, S. 2003. Housing reconstruction after conflict and disaster. London: Overseas Development Institute.
Belcher, J. C., and F. L. Bates. 1983. “Aftermath of natural disasters: Coping through residential mobility.” Disasters 7 (2): 118–128. https://doi.org/10.1111/j.1467-7717.1983.tb00805.x.
Bengtsson, L., X. Lu, A. Thorson, R. Garfield, and J. von Schreeb. 2011. “Improved response to disasters and outbreaks by tracking population movements with mobile phone network data: A post-earthquake geospatial study in Haiti.” PLoS Med. 8 (8): e1001083. https://doi.org/10.1371/journal.pmed.1001083.
Bhattacharya, Y., and T. Kato. 2021. “Development of an agent-based model on the decision-making of dislocated people after disasters.” In Urban informatics and future cities, edited by S. C. M. Geertman, C. Pettit, R. Goodspeed, and A. Staffans, 387–406. Berlin: Springer. https://doi.org/10.1007/978-3-030-76059-5_20.
Binder, S. B., C. K. Baker, and J. P. Barile. 2015. “Rebuild or relocate? Resilience and postdisaster decision-making after hurricane sandy.” Am. J. Commun. Psychol. 56 (1): 180–196. https://doi.org/10.1007/s10464-015-9727-x.
Blaikie, P., T. Cannon, I. Davis, and B. Wisner. 2014. At risk: Natural hazards, people’s vulnerability and disasters. 2nd ed. London: Routledge. https://doi.org/10.4324/9780203714775.
Blaze, J. T., and D. W. Shwalb. 2009. “Resource loss and relocation: A follow-up study of adolescents two years after Hurricane Katrina.” Psychol. Trauma: Theory Res. Pract. Policy 1 (4): 312. https://doi.org/10.1037/a0017834.
Bolin, R. C. 1982. “Long-term family recovery from disaster.” In Long-term family recovery from disaster, 278. Boulder, CO: Univ. of Colorado.
Bolin, R. C. 1985. “Disasters and long-term recovery policy: A focus on housing and families.” Rev. Policy Res. 4 (4): 709–715. https://doi.org/10.1111/j.1541-1338.1985.tb00319.x.
Bolin, R. C. 1993. “Post-earthquake shelter and housing: Research findings and policy implications.” In Monograph 5: Socioeconomic Impacts, 107–131. Memphis, TN: Central United States Earthquake Consortium.
Bolin, R. C., and D. J. Klenow. 1988. “Older people in disaster: A comparison of black and white victims.” Int. J. Aging Human Dev. 26 (1): 29–43. https://doi.org/10.2190/RHK2-416V-MGXH-HYKL.
Bolin, R. C., and L. Stanford. 1991. “Shelter, housing and recovery: A comparison of US disasters.” Disasters 15 (1): 24–34. https://doi.org/10.1111/j.1467-7717.1991.tb00424.x.
Bolin, R. C., and L. Stanford. 1998. “The Northridge earthquake: Community-based approaches to unmet recovery needs.” Disasters 22 (1): 21–38. https://doi.org/10.1111/1467-7717.00073.
Bonaiuto, M., S. Alves, S. De Dominicis, and I. Petruccelli. 2016. “Place attachment and natural hazard risk: Research review and agenda.” J. Environ. Psychol. 48 (Dec): 33–53. https://doi.org/10.1016/j.jenvp.2016.07.007.
Burton, H., H. Kang, S. Miles, A. Nejat, and Z. Yi. 2019. “A framework and case study for integrating household decision-making into post-earthquake recovery models.” Int. J. Disaster Risk Reduct. 37 (Jun): 101167. https://doi.org/10.1016/j.ijdrr.2019.101167.
Burton, H. V., G. Deierlein, D. Lallemant, and T. Lin. 2016. “Framework for incorporating probabilistic building performance in the assessment of community seismic resilience.” J. Struct. Eng. 142 (8): C4015007. https://doi.org/10.1061/(ASCE)ST.1943-541X.0001321.
Cavalieri, F., P. Franchin, P. Gehl, and B. Khazai. 2012. “Quantitative assessment of social losses based on physical damage and interaction with infrastructural systems.” Earthquake Eng. Struct. Dyn. 41 (11): 1569–1589. https://doi.org/10.1002/eqe.2220.
Chamlee-Wright, E., and V. H. Storr. 2009. “‘There’s no place like New Orleans’: Sense of place and community recovery in the Ninth Ward after Hurricane Katrina.” J. Urban Affairs 31 (5): 615–634. https://doi.org/10.1111/j.1467-9906.2009.00479.x.
Chang, S. E. 2010. “Urban disaster recovery: A measurement framework and its application to the 1995 Kobe earthquake.” Disasters 34 (2): 303–327. https://doi.org/10.1111/j.1467-7717.2009.01130.x.
Chang, S. E. 2016. “Socioeconomic impacts of infrastructure disruptions.” In Oxford research encyclopedia of natural hazard science. Oxford, UK: Oxford University Press. https://doi.org/10.1093/acrefore/9780199389407.013.66.
Chang, S. E., C. Pasion, K. Tatebe, and R. Ahmad. 2008. Linking lifeline infrastructure performance and community disaster resilience: Models and multi-stakeholder processes. Washington, DC: National Science Foundation.
Chien, S. W., L. C. Chen, S. Y. Chang, G. H. Chiu, and C. L. Chu. 2002. “Development of an after earthquake disaster shelter evaluation model.” J. Chin. Inst. Eng. 25 (5): 591–596. https://doi.org/10.1080/02533839.2002.9670733.
Cole, P. M. S. 2003. “An empirical examination of the housing recovery process following disaster.” Ph.D. thesis, Dept. of Architecture, Texas A&M Univ.
Comerio, M. C. 1997. “Housing issues after disasters.” J. Contingencies Crisis Manage. 5 (3): 166–178. https://doi.org/10.1111/1468-5973.00052.
Comerio, M. C. 1998. Disaster hits home: New policy for urban housing recovery. Berkeley, CA: University of California Press.
Comerio, M. C. 2006. “Estimating downtime in loss modeling.” Earthquake Spectra 22 (2): 349–365. https://doi.org/10.1193/1.2191017.
Comerio, M. C. 2014. “Disaster recovery and community renewal: Housing approaches.” Cityscape 16 (2): 51–68.
Comerio, M. C., and H. E. Blecher. 2010. “Estimating downtime from data on residential buildings after the Northridge and Loma Prieta earthquakes.” Earthquake Spectra 26 (4): 951–965. https://doi.org/10.1193/1.3477993.
Cong, Z., A. Nejat, D. Liang, Y. Pei, and R. J. Javid. 2018. “Individual relocation decisions after tornadoes: A multi-level analysis.” Disasters 42 (2): 233–250. https://doi.org/10.1111/disa.12241.
Corsellis, T., and A. Vitale. 2005. “Transitional settlement.” In Displaced population. Cambridge, UK: Univ. of Cambridge.
Costa, R., T. Haukaas, and S. E. Chang. 2021. “Agent-based model for post-earthquake housing recovery.” Earthquake Spectra 37 (1): 46–72. https://doi.org/10.1177/8755293020944175.
Costa, R., T. Haukaas, and S. E. Chang. 2022a. “Predicting population displacements after earthquakes.” Sustainable Resilient Infrastruct. 7 (4): 253–271. https://doi.org/10.1080/23789689.2020.1746047.
Costa, R., C. Wang, and J. W. Baker. 2022b. “Integrating place attachment into housing recovery simulations to estimate population losses.” Nat. Hazard. Rev. 23 (4): 04022021. https://doi.org/10.1061/(ASCE)NH.1527-6996.0000571.
Cremen, G., et al. 2023. “A state-of-the-art decision-support environment for risk-sensitive and pro-poor urban planning and design in tomorrow’s cities.” Int. J. Disaster Risk Reduct. 85 (Feb): 103400. https://doi.org/10.1016/j.ijdrr.2022.103400.
Cutter, S. L., B. J. Boruff, and W. L. Shirley. 2003. “Social vulnerability to environmental hazards.” Soc. Sci. Q. 84 (2): 242–261. https://doi.org/10.1111/1540-6237.8402002.
Dash, N., and H. Gladwin. 2007. “Evacuation decision making and behavioral responses: Individual and household.” Nat. Hazards Rev. 8 (3): 69–77. https://doi.org/10.1061/(ASCE)1527-6988(2007)8:3(69).
Dash, N., W. Peacock, and B. Morrow. 1997. And the poor get poorer: A neglected black community, 206–225. London: Routledge.
Elliott, J. R., and J. Pais. 2006. “Race, class, and Hurricane Katrina: Social differences in human responses to disaster.” Soc. Sci. Res. 35 (2): 295–321. https://doi.org/10.1016/j.ssresearch.2006.02.003.
Emrich, C. T., S. K. Aksha, and Y. Zhou. 2022. “Assessing distributive inequities in FEMA’s disaster recovery assistance fund allocation.” Int. J. Disaster Risk Reduct. 74 (May): 102855. https://doi.org/10.1016/j.ijdrr.2022.102855.
Esnard, A. M., and A. Sapat. 2014. Displaced by disaster: Recovery and resilience in a globalizing world. New York: Routledge. https://doi.org/10.4324/9780203728291.
FEMA. 2003. HAZUS-MH technical manual. Washington, DC: FEMA.
Financial Services Roundtable. 2006. “Accelerating the Katrina recovery: An interim report by the Blue Ribbon Commission on Mega-Catastrophes of the Financial Services Roundtable.” Accessed April 7, 2023. https://web.archive.org/web/20120217135538/http://www.fsround.org/publications/pdfs/KATRINAFinalDocument.pdf.
Finch, C., C. T. Emrich, and S. L. Cutter. 2010. “Disaster disparities and differential recovery in New Orleans.” Popul. Environ. 31 (4): 179–202. https://doi.org/10.1007/s11111-009-0099-8.
Fothergill, A., and L. A. Peek. 2004. “Poverty and disasters in the United States: A review of recent sociological findings.” Nat. Hazards 32 (1): 89–110. https://doi.org/10.1023/B:NHAZ.0000026792.76181.d9.
Fothergill, A., and L. A. Peek. 2015. Children of Katrina. Austin, TX: University of Texas Press.
Freeman, P. K. 2004. “Allocation of post-disaster reconstruction financing to housing.” Build. Res. Inf. 32 (5): 427–437. https://doi.org/10.1080/0961321042000221016.
Fussell, E., N. Sastry, and M. VanLandingham. 2010. “Race, socioeconomic status, and return migration to New Orleans after Hurricane Katrina.” Popul. Environ. 31 (1): 20–42. https://doi.org/10.1007/s11111-009-0092-2.
Gerber, B. J. 2010. “Management of evacuee ingress during disasters: Identifying the determinants of local government capacity and preparedness.” Risk Hazards Crisis Public Policy 1 (3): 115–142. https://doi.org/10.2202/1944-4079.1063.
Girard, C., and W. G. Peacock. 1997. “Ethnicity and segregation: Post-hurricane relocation.” In Hurricane Andrew: Ethnicity, gender sociology disasters, 191–205. London: Routledge.
Gray, C., E. Frankenberg, T. Gillespie, C. Sumantri, and D. Thomas. 2009. “Population displacement and mobility in Sumatra after the tsunami.” In Proc., 26th Int. Population Conf. of the IUSSP. Princeton, NJ: Princeton Univ.
Greene, M. 1992. “Housing recovery and reconstruction: Lessons from recent urban earthquakes.” In Proc., 3rd US/Japan Workshop on Urban Earthquakes. Oakland, CA: Earthquake Engineering Research Institute.
Greer, A. 2015. Household residential decision-making in the wake of disaster: Cases from Hurricane Sandy. Newark, DE: Univ. of Delaware.
Greer, A., J. Trainor, and S. McNeil. 2019. “Voluntary household relocation decision making in the wake of disaster: Re-interpreting the empirical record.” Int. J. Mass Emerg. Disasters 37 (2): 197–226. https://doi.org/10.1177/028072701903700206.
Grinberger, A. Y., and D. Felsenstein. 2016. “Dynamic agent based simulation of welfare effects of urban disasters.” Comput. Environ. Urban Syst. 59 (Sep): 129–141. https://doi.org/10.1016/j.compenvurbsys.2016.06.005.
Groen, J. A., and A. E. Polivka. 2010. “Going home after Hurricane Katrina: Determinants of return migration and changes in affected areas.” Demography 47 (4): 821–844. https://doi.org/10.1007/BF03214587.
Haas, J. E., R. W. Kates, and M. J. Bowden. 1977. “Reconstruction following disaster.” In Reconstruction following disaster. Cambridge, MA: Massachusetts Institute of Technology.
Hallegatte, S., A. Vogt-Schilb, J. Rozenberg, M. Bangalore, and C. Beaudet. 2020. “From poverty to disaster and back: A review of the literature.” Econ. Disasters Clim. Change 4 (1): 223–247. https://doi.org/10.1007/s41885-020-00060-5.
Hamideh, S., W. Gills Peacock, and S. Van Zandt. 2021. “Housing type matters for pace of recovery: Evidence from Hurricane Ike.” Int. J. Disaster Risk Reduct. 57 (Apr): 102149. https://doi.org/10.1016/j.ijdrr.2021.102149.
Hamideh, S., P. Sen, and E. Fischer. 2022. “Wildfire impacts on education and healthcare: Paradise, California, after the Camp Fire.” Nat. Hazards 111 (1): 353–387. https://doi.org/10.1007/s11069-021-05057-1.
Hansel Tonya, C., D. Osofsky Joy, J. Osofsky Howard, and F. Patricia. 2013. “The effect of long-term relocation on child and adolescent survivors of Hurricane Katrina.” J. Traumatic Stress 26 (5): 613. https://doi.org/10.1002/jts.21837.
Henry, J. 2013. “Return or relocate? An inductive analysis of decision-making in a disaster.” Disasters 37 (2): 293–316. https://doi.org/10.1111/j.1467-7717.2012.01303.x.
Hidalgo, M. C., and B. Hernández. 2001. “Place attachment: Conceptual and empirical questions.” J. Environ. Psychol. 21 (3): 273–281. https://doi.org/10.1006/jevp.2001.0221.
Hirayama, Y. 2000. “Collapse and reconstruction: Housing recovery policy in Kobe after the Hanshin Great earthquake.” Housing Stud. 15 (1): 111–128. https://doi.org/10.1080/02673030082504.
Hori, M., and M. J. Schafer. 2010. “Social costs of displacement in louisiana after Hurricanes Katrina and Rita.” Popul. Environ. 31 (Apr): 64–86. https://doi.org/10.1007/s11111-009-0094-0.
Howell, J., and J. R. Elliott. 2019. “Damages done: The longitudinal impacts of natural hazards on wealth inequality in the United States.” Soc. Probl. 66 (3): 448–467. https://doi.org/10.1093/socpro/spy016.
Hu, D., W. Yu, J. Zhao, W. Liu, F. Han, and X. Yi. 2019. “A hierarchical mixed logit model of individuals’ return decisions after Hurricane Katrina.” Int. J. Disaster Risk Reduct. 34 (Mar): 443–447. https://doi.org/10.1016/j.ijdrr.2018.12.015.
Husby, T. G., and E. E. Koks. 2017. “Household migration in disaster impact analysis: Incorporating behavioural responses to risk.” Nat. Hazards 87 (1): 287–305. https://doi.org/10.1007/s11069-017-2763-0.
IDMC (Internal Displacement Monitoring Centre). 2017. “Global disaster displacement risk—A baseline for future work.” Accessed March 29, 2023. https://www.internal-displacement.org/publications/global-disaster-displacement-risk-a-baseline-for-future-work.
IDMC (Internal Displacement Monitoring Centre). 2019. “Disaster displacement—A global review, 2008–2018.” Accessed March 29, 2023. https://www.internal-displacement.org/publications/disaster-displacement-a-global-review.
IDMC (Internal Displacement Monitoring Centre). 2020. “Global report on internal displacement: Methodological annex.” Accessed March 29, 2023. https://www.internal-displacement.org/global-report/grid2020/downloads/2020-IDMC-GRID-methodology.pdf.
IOM (International Organization for Migration) and IDMC (Internal Displacement Monitoring Centre). 2022. “Displacement indicators for DRR: Analysis of existing methodologies, research and datasets.” Accessed March 29, 2023. https://environmentalmigration.iom.int/sites/g/files/tmzbdl1411/files/documents/Displacement%20indicators%20for%20DRR%20State%20of%20the%20art%20report.pdf.
IPCC (Intergovernmental Panel on Climate Change). 2012. “Summary for policymakers.” In Managing the risks of extreme events and disasters to advance climate change adaptation. 1st ed. Cambridge, UK: Cambridge University Press. https://doi.org/10.1017/CBO9781139177245.
Johnson, L. A., J. L. Jones, A. M. Wein, and J. A. Peters. 2020. “Analysis of communities at risk in the haywired scenario.” In The haywired earthquake scenario—Societal consequences. Reston, VA: USGS.
Johnson, L. A., and R. B. Olshansky. 2016. After great disasters: How six countries managed community recovery. Phoenix: Lincoln Institute of Land Policy Cambridge.
Kamel, N. M. O., and A. Loukaitou-Sideris. 2004. “Residential assistance and recovery following the Northridge earthquake.” Urban Studies 41 (3): 533–562. https://doi.org/10.1080/0042098042000178672.
Kates, R. W., C. E. Colten, S. Laska, and S. P. Leatherman. 2006. “Reconstruction of New Orleans after Hurricane Katrina: A research perspective.” Proc. Natl. Acad. Sci. U.S.A. 103 (40): 14653–14660. https://doi.org/10.1073/pnas.0605726103.
Khazai, B., J. Daniell, P. Franchin, F. Cavalieri, B. Vangelsten, I. Iervolino, and S. Esposito. 2012. “A new approach to modeling post-earthquake shelter demand: Integrating social vulnerability in systemic seismic vulnerability analysis.” In Proc., 15WCEE – 15th World Conf. on Earthquake Engineering. Red Hook, NY: Curran Associates. https://doi.org/10.13140/2.1.3023.7129.
Kim, J., and S. Soo Oh. 2014. “The virtuous circle in disaster recovery: Who returns and stays in town after disaster evacuation?” J. Risk Res. 17 (5): 665–682. https://doi.org/10.1080/13669877.2013.822917.
Lee, H. C., and H. Chen. 2018. “Social determinants in choice of shelter: An evidence-based analysis.” Nat. Hazards 93 (3): 1277–1294. https://doi.org/10.1007/s11069-018-3352-6.
Lee, J. Y., and S. Van Zandt. 2019. “Housing tenure and social vulnerability to disasters: A review of the evidence.” J. Plann. Lit. 34 (2): 156–170. https://doi.org/10.1177/0885412218812080.
Lee, Y. J., H. Sugiura, and I. Gečienė. 2017. “Stay or relocate: The roles of networks after the Great East Japan earthquake.” Chap. 15 in Social network analysis of disaster response, recovery, and adaptation, edited by E. C. Jones and A. J. Faas, 223–238. Oxford, UK: Butterworth-Heinemann. https://doi.org/10.1016/B978-0-12-805196-2.00015-7.
Lin, N. 2008. “A network theory of social capital.” In The handbook of social capital, 50–69. Oxford, UK: Oxford University Press.
Lin, Y. S. 2009. Development of algorithms to estimate post-disaster population dislocation—A research-based approach. Austin, TX: Texas A&M Univ.
Lines, R., J. P. Faure Walker, and R. Yore. 2022. “Progression through emergency and temporary shelter, transitional housing and permanent housing: A longitudinal case study from the 2018 Lombok earthquake, Indonesia.” Int. J. Disaster Risk Reduct. 75 (Jun): 102959. https://doi.org/10.1016/j.ijdrr.2022.102959.
Lu, J. C., W. G. Peacock, Y. Zhang, and N. Dash. 2007. “Long-term housing recovery: Does housing type really make a difference.” In Proc., of 2nd Int. Conf. on Urban Disaster Reduction. New Taipei City, Taiwan: National Science and Technology Center for Disaster Reduction.
Lu, X., L. Bengtsson, and P. Holme. 2012. “Predictability of population displacement after the 2010 Haiti earthquake.” Proc. Natl. Acad. Sci. U.S.A. 109 (29): 11576. https://doi.org/10.1073/pnas.1203882109.
Markhvida, M., B. Walsh, S. Hallegatte, and J. Baker. 2020. “Quantification of disaster impacts through household well-being losses.” Nat. Sustainability 3 (7): 538–547. https://doi.org/10.1038/s41893-020-0508-7.
Mayer, J., S. Moradi, A. Nejat, S. Ghosh, Z. Cong, and D. Liang. 2020. “Drivers of post-disaster relocations: The case of Moore and Hattiesburg tornados.” Int. J. Disaster Risk Reduct. 49 (Oct): 101643. https://doi.org/10.1016/j.ijdrr.2020.101643.
McAdam, J. 2022. “Evacuations: A form of disaster displacement?” Forced Migr. Rev. 69 (Mar): 56–57.
Miles, S. B., H. V. Burton, and H. Kang. 2019. “Community of practice for modeling disaster recovery.” Nat. Hazards Rev. 20 (1): 04018023. https://doi.org/10.1061/(ASCE)NH.1527-6996.0000313.
Miles, S. B., and S. E. Chang. 2011. “ResilUS: A community based disaster resilience model.” Cartogr. Geogr. Inf. Sci. 38 (1): 36–51. https://doi.org/10.1559/1523040638136.
Miller, D. S., and J. D. Rivera. 2007. “Landscapes of disaster and place orientation in the aftermath of Hurricane Katrina.” In The sociology of Katrina: Perspectives on a modern catastrophe, 141–154. Lanham, MD: Rowman & Littlefield Publishers.
Mitchell, C. M., A. M. Esnard, and A. Sapat. 2012. “Hurricane events, population displacement, and sheltering provision in the United States.” Nat. Hazards Rev. 13 (2): 150–161. https://doi.org/10.1061/(ASCE)NH.1527-6996.0000064.
Mitchell, D., D. Grant, D. Roberge, G. Prasad Bhatta, and C. Caceres. 2017. “An evaluation framework for earthquake-responsive land administration.” Land Use Policy 67 (Sep): 239–252. https://doi.org/10.1016/j.landusepol.2017.05.020.
Mongold, E., R. Costa, Á. Zsarnóczay, and J. W. Baker. 2022. “Simulating post-disaster landlord residence and rental unit recovery.” In Proc., 12th National Conf. on Earthquake Engineering. Oakland, CA: Earthquake Engineering Research Institute.
Morrice, S. 2013. “Heartache and Hurricane Katrina: Recognising the influence of emotion in post-disaster return decisions.” Area 45 (1): 33–39. https://doi.org/10.1111/j.1475-4762.2012.01121.x.
Morrow, B. H. 1999. “Identifying and mapping community vulnerability.” Disasters 23 (1): 1–18. https://doi.org/10.1111/1467-7717.00102.
Morrow-Jones, H. A., and C. R. Morrow-Jones. 1991. “Mobility due to natural disaster: Theoretical considerations and preliminary analyses.” Disasters 15 (2): 126–132. https://doi.org/10.1111/j.1467-7717.1991.tb00441.x.
Mukherji, A. 2010. “Post-earthquake housing recovery in Bachhau, India: The homeowner, the renter, and the squatter.” Earthquake Spectra 26 (4): 1085–1100. https://doi.org/10.1193/1.3474646.
Mukherji, A. 2014. “Post-disaster housing recovery: The promise and peril of social capital.” J. Civ. Soc. 10 (2): 119–143. https://doi.org/10.1080/17448689.2014.885787.
Mukherji, A. 2015. “From tenants to homeowners: Housing renters after disaster in Bhuj, India.” Housing Stud. 30 (7): 1135–1157. https://doi.org/10.1080/02673037.2015.1008423.
Mukherji, A. 2017. “Post-disaster housing recovery.” Oxford Res. Encycl. Nat. Hazard Sci. 10 (2): 119–143. https://doi.org/10.1093/acrefore/9780199389407.013.82.
Mukherji, A. 2018. “Resilience at the margins: Informal housing recovery in Bachhau, India, after the 2001 Gujarat quake.” Int. J. Housing Policy 18 (2): 266–289. https://doi.org/10.1080/14616718.2016.1219648.
Nabong, E., A. Opdyke, M. K. Alinsunurin, S. Merrill, G. Mcdonald, and P. Jensen. 2021. A collaborative research framework for humanitarian shelter and settlements assistance, 97–106. Washington, DC: InterAction.
Nakagawa, Y., and R. Shaw. 2004. “Social capital: A missing link to disaster recovery.” Int. J. Mass Emerg. Disasters 22 (1): 5–34. https://doi.org/10.1177/028072700402200101.
Nawrotzki, R. J., H. Brenkert-Smith, L. M. Hunter, and P. A. Champ. 2014. “Wildfire-migration dynamics: Lessons from Colorado’s fourmile canyon fire.” Soc. Nat. Resour. 27 (2): 215–225. https://doi.org/10.1080/08941920.2013.842275.
Nejat, A., Z. Cong, and D. Liang. 2016. “Family structures, relationships, and housing recovery decisions after hurricane sandy.” Buildings 6 (2): 14. https://doi.org/10.3390/buildings6020014.
Nejat, A., and I. Damnjanovic. 2012. “Agent-based modeling of behavioral housing recovery following disasters.” Comput.-Aided Civ. Infrastruct. Eng. 27 (10): 748–763. https://doi.org/10.1111/j.1467-8667.2012.00787.x.
Nejat, A., and S. Ghosh. 2016. “LASSO model of postdisaster housing recovery: Case study of hurricane sandy.” Nat. Hazards Rev. 17 (3): 04016007. https://doi.org/10.1061/(ASCE)NH.1527-6996.0000223.
Nejat, A., S. Moradi, and S. Ghosh. 2019. “Anchors of social network awareness index: A key to modeling postdisaster housing recovery.” J. Infrastruct. Syst. 25 (2): 04019004. https://doi.org/10.1061/(ASCE)IS.1943-555X.0000471.
Ngo, E. B. 2001. “When disasters and age collide: Reviewing vulnerability of the elderly.” Nat. Hazards Rev. 2 (2): 80–89. https://doi.org/10.1061/(ASCE)1527-6988(2001)2:2(80).
Pardee, J. W. 2012. Living through displacement: Housing insecurity among low-income evacuees, 63–78. Austin, TX: University of Texas Press. https://doi.org/10.7560/735774-008.
Peacock, W. G., N. Dash, Y. Zhang, and S. Van Zandt. 2018. “Post-disaster sheltering, temporary housing and permanent housing recovery.” In Handbook of disaster research. 2nd ed., 569–594. New York: Springer.
Peacock, W. G., S. Van Zandt, Y. Zhang, and W. E. Highfield. 2014. “Inequities in long-term housing recovery after disasters.” J. Am. Plann. Assoc. 80 (4): 356–371. https://doi.org/10.1080/01944363.2014.980440.
Peek, L., B. Morrissey, and H. Marlatt. 2011. “Disaster hits home: A model of displaced family adjustment after Hurricane Katrina.” J. Family Issues 32 (10): 1371–1396. https://doi.org/10.1177/0192513X11412496.
Perry, R. W., and M. K. Lindell. 2003. “Preparedness for emergency response: Guidelines for the emergency planning process.” Disasters 27 (4): 336–350. https://doi.org/10.1111/j.0361-3666.2003.00237.x.
Phillips, B. D. 1993. “Cultural diversity in disasters: Sheltering, housing, and long term recovery.” Int. J. Mass Emerg. Disasters 11 (1): 99–110. https://doi.org/10.1177/028072709301100108.
Picou, J. S., and B. K. Marshall. 2007. “Social impacts of Hurricane Katrina on displaced K–12 students and educational institutions in coastal Alabama counties: Some preliminary observations.” Sociol. Spectrum 27 (6): 767–780. https://doi.org/10.1080/02732170701534267.
Prater, C. S., and M. K. Lindell. 2000. “Politics of hazard mitigation.” Nat. Hazards Rev. 1 (2): 73–82. https://doi.org/10.1061/(ASCE)1527-6988(2000)1:2(73).
Pu, G., A. Chang-Richards, S. Wilkinson, and R. Potangaroa. 2021. “What makes a successful livelihood recovery? A study of China’s Lushan earthquake.” Nat. Hazards 105 (3): 2543–2567. https://doi.org/10.1007/s11069-020-04412-y.
Quarantelli, E. L. 1982. “General and particular observations on sheltering and housing in american disasters.” Disasters 6 (4): 277–281. https://doi.org/10.1111/j.1467-7717.1982.tb00550.x.
Quarantelli, E. L. 1995. “Patterns of sheltering and housing in US disasters.” Disaster Prev. Manage. Int. J. 4 (3): 43–53. https://doi.org/10.1108/09653569510088069.
Rubinyi, S., J. Verschuur, R. Goldblatt, J. Gussenbauer, A. Kowarik, J. Mannix, B. Bottoms, and J. Hall. 2022. “High-resolution synthetic population mapping for quantifying disparities in disaster impacts: An application in the Bangladesh coastal zone.” Front. Environ. Sci. 10 (Nov): 2363. https://doi.org/10.3389/fenvs.2022.1033579.
Rufat, S. 2013. “Spectroscopy of urban vulnerability.” Ann. Assoc. Am. Geogr. 103 (3): 505–525. https://doi.org/10.1080/00045608.2012.702485.
Sanders, S., S. Bowie, and Y. Bowie. 2004. “Chapter 2 lessons learned on forced relocation of older adults.” J. Gerontol. Soc. Work 40 (4): 23–35. https://doi.org/10.1300/J083v40n04_03.
Sanderson, D., A. Sharma, J. Kennedy, and J. Burnell. 2014. “Principles, practice and lessons from Haiti for urban post-disaster shelter recovery programs.” Asian J. Environ. Disaster Manage. 6 (2): 131–151. https://doi.org/10.3850/S1793924014000362.
Sapat, A., and A. M. Esnard. 2016. Coming home after disaster: Multiple dimensions of housing recovery. New York: Routledge. https://doi.org/10.4324/9781315404264.
Scannell, L., and R. Gifford. 2010. “Defining place attachment: A tripartite organizing framework.” J. Environ. Psychol. 30 (1): 1–10. https://doi.org/10.1016/j.jenvp.2009.09.006.
Scheele, F., T. Wilson, E. M. Lane, K. Crowley, M. W. Hughes, T. Davies, and N. Horspool. 2020. “Modelling residential habitability and human displacement for tsunami scenarios in Christchurch, New Zealand.” Int. J. Disaster Risk Reduct. 43 (Feb): 101403. https://doi.org/10.1016/j.ijdrr.2019.101403.
Smith, S. K., and C. McCarty. 1996. “Demographic effects of natural disasters: A case study of Hurricane Andrew.” Demography 33 (2): 265–275. https://doi.org/10.2307/2061876.
Sou, G., and K. Howarth. 2023. “Waiting during disasters: Negotiating the spatio-temporalities of resilience and recovery.” Geogr. Res. 61 (2): 273–284. https://doi.org/10.1111/1745-5871.12583.
Sphere Project. 2011. Humanitarian charter and minimum standards in humanitarian response—Russian. Rugby, UK: Practical Action.
Spoon, J., D. Gerkey, R. B. Chhetri, A. Rai, U. Basnet, and C. E. Hunter. 2021. “Understanding short-term household recoveries from the 2015 Nepal earthquakes: Lessons learned and recommendations.” Progress Disaster Sci. 10 (Apr): 100169. https://doi.org/10.1016/j.pdisas.2021.100169.
SPUR. 2012. “Safe enough to stay.” Accessed January 26, 2023. https://www.spur.org/publications/spur-report/2012-02-01/safe-enough-stay.
Storr, V. H., and S. H. Balch. 2012. “Post-disaster community recovery in heterogeneous, loosely connected communities.” Rev. Soc. Econ. 70 (3): 295–314. https://doi.org/10.1080/00346764.2012.662786.
Sutley, E. J., and S. Hamideh. 2020. “Postdisaster housing stages: A Markov chain approach to model sequences and duration based on social vulnerability.” Risk Anal. 40 (12): 2675–2695. https://doi.org/10.1111/risa.13576.
Sutley, E. J., S. Hamideh, M. Dillard, D. Gu, K. Seong, and J. Van De Lindt. 2019. “Integrative modeling of housing recovery as a physical, economic, and social process.” In Proc., 13th Int. Conf. on Applications of Statistics and Probability in Civil Engineering. Seoul: International Civil Engineering Risk and Reliability Association.
Taheri Tafti, M., and M. Bashiri. 2022. “Navigating transition: Post-disaster housing pathways of households.” J. Hous. Built Environ. 37 (4): 1745–1767. https://doi.org/10.1007/s10901-021-09918-w.
Taheri Tafti, M., and R. Tomlinson. 2013. “The role of post-disaster public policy responses in housing recovery of tenants.” Habitat Int. 40 (Oct): 218–224. https://doi.org/10.1016/j.habitatint.2013.05.004.
Taheri Tafti, M., and R. Tomlinson. 2015. “Best practice post-disaster housing and livelihood recovery interventions: Winners and losers.” Int. Dev. Plann. Rev. 37 (2): 165–185. https://doi.org/10.3828/idpr.2015.14.
Takahashi, S., M. Nakamura, Y. Yonekura, K. Tanno, K. Sakata, A. Ogawa, and S. Kobayashi. 2016. “Association between relocation and changes in cardiometabolic risk factors: A longitudinal study in tsunami survivors of the 2011 Great East Japan earthquake.” BMJ Open 6 (5): e011291. https://doi.org/10.1136/bmjopen-2016-011291.
Thompson, R. R., D. R. Garfin, and R. C. Silver. 2017. “Evacuation from natural disasters: A systematic review of the literature.” Risk Anal. 37 (4): 812–839. https://doi.org/10.1111/risa.12654.
Tierney, K. J., M. K. Lindell, and R. W. Perry. 2001. Facing the unexpected: Disaster preparedness and response in the United States. Washington, DC: Joseph Henry. https://doi.org/10.17226/9834.
UNDRR (United Nations Office for Disaster Risk Reduction). 2019. “Disaster displacement: How to reduce risk, address impacts, and strengthen resilience.” Accessed April 12, 2023. https://www.undrr.org/words-into-action/disaster-displacement-how-reduce-risk-address-impacts-and-strengthen-resilience.
Vecere, A., R. Monteiro, W. J. Ammann, S. Giovinazzi, and R. H. Melo Santos. 2017. “Predictive models for post disaster shelter needs assessment.” Int. J. Disaster Risk Reduct. 21 (Jun): 44–62. https://doi.org/10.1016/j.ijdrr.2016.11.010.
Williams, D. R., and J. J. Vaske. 2003. “The measurement of place attachment: Validity and generalizability of a psychometric approach.” For. Sci. 49 (6): 830–840.
Wilson, R., et al. 2016. “Rapid and near real-time assessments of population displacement using mobile phone data following disasters: The 2015 Nepal earthquake.” PLoS Currents 8. https://doi.org/10.1371/currents.dis.d073fbece328e4c39087bc086d694b5c.
Winsemius, H. C., B. Jongman, T. I. E. Veldkamp, S. Hallegatte, M. Bangalore, and P. J. Ward. 2018. “Disaster risk, climate change, and poverty: Assessing the global exposure of poor people to floods and droughts.” Environ. Dev. Econ. 23 (3): 328–348. https://doi.org/10.1017/S1355770X17000444.
Woolcock, M. 2002. Social capital in theory and practice: Where do we stand? Social capital and economic development. Cheltenham, UK: Edward Elgar.
Wright, K. C., and D. M. Johnston. 2010. “Post-earthquake sheltering needs: How loss of structures and services affects decision making for evacuation.” In Proc., 2010 New Zealand Society for Earthquake Engineering Conf., 23. Christchurch, New Zealand: New Zealand Society for Earthquake Engineering.
Wu, J. Y., and M. K. Lindell. 2004. “Housing reconstruction after two major earthquakes: The 1994 Northridge earthquake in the United States and the 1999 Chi-Chi earthquake in Taiwan.” Disasters 28 (1): 63–81. https://doi.org/10.1111/j.0361-3666.2004.00243.x.
Wyczalkowski, C. K., E. J. Van Holm, A. M. Esnard, and B. S. Lai. 2019. “Uneven neighborhood recovery: Hurricane damage and neighborhood change in the Houston–Galveston region since 1970.” City Commun. 18 (2): 689–709. https://doi.org/10.1111/cico.12390.
Xiao, Y., and S. Van Zandt. 2012. “Building community resiliency: Spatial links between household and business post-disaster return.” Urban Studies 49 (11): 2523–2542. https://doi.org/10.1177/0042098011428178.
Xu, D., C. Qing, X. Deng, Z. Yong, W. Zhou, and Z. Ma. 2020. “Disaster risk perception, sense of pace, evacuation willingness, and relocation willingness of rural households in earthquake-stricken areas: Evidence from Sichuan Province, China.” Int. J. Environ. Res. Public Health 17 (2): 602. https://doi.org/10.3390/ijerph17020602.
Yabe, T., Y. Sekimoto, K. Tsubouchi, and S. Ikemoto. 2019. “Cross-comparative analysis of evacuation behavior after earthquakes using mobile phone data.” PLoS One 14 (2): e0211375. https://doi.org/10.1371/journal.pone.0211375.
Yabe, T., K. Tsubouchi, N. Fujiwara, Y. Sekimoto, and S. V. Ukkusuri. 2020. “Understanding post-disaster population recovery patterns.” J. R. Soc. Interface 17 (163): 20190532. https://doi.org/10.1098/rsif.2019.0532.
Zhang, Y., and W. G. Peacock. 2009. “Planning for housing recovery? Lessons learned from Hurricane Andrew.” J. Am. Plann. Assoc. 76 (1): 5–24. https://doi.org/10.1080/01944360903294556.
Zissimopoulos, J., and L. A. Karoly. 2010. “Employment and self-employment in the wake of Hurricane Katrina.” Demography 47 (2): 345–367. https://doi.org/10.1353/dem.0.0099.
Information & Authors
Information
Published In
Copyright
This work is made available under the terms of the Creative Commons Attribution 4.0 International license, https://creativecommons.org/licenses/by/4.0/.
History
Published online: Dec 13, 2023
Published in print: Feb 1, 2024
Discussion open until: May 13, 2024
ASCE Technical Topics:
- Buildings
- Business management
- Continuum mechanics
- Disaster preparedness
- Disaster recovery
- Disaster risk management
- Disasters and hazards
- Displacement (mechanics)
- Emergency management
- Engineering mechanics
- Evacuation
- Existing buildings
- Housing
- Infrastructure
- Practice and Profession
- Social factors
- Solid mechanics
- Structural engineering
- Structural mechanics
- Structures (by type)
- Urban and regional development
Authors
Metrics & Citations
Metrics
Citations
Download citation
If you have the appropriate software installed, you can download article citation data to the citation manager of your choice. Simply select your manager software from the list below and click Download.