Abstract
Introduction
Vehicle Safety Inspection and Maintenance Programs
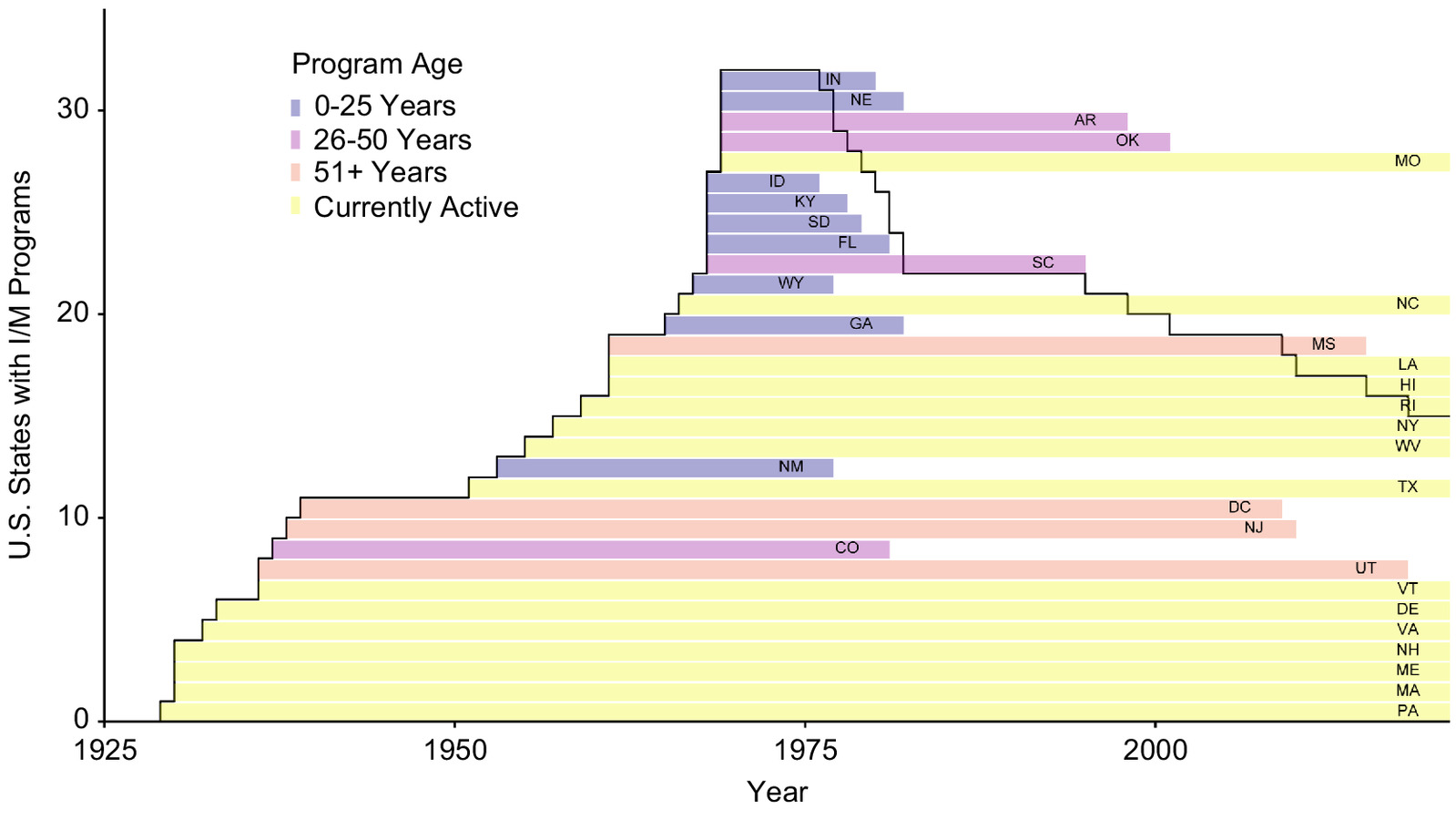
Literature Review
Early Quantitative Analyses
State- and Regional-Level Models
Studies Applying Highway Statistics Data
International Programs
Motivation
Contributions of This Study
Data
Fatality Analysis Reporting System
Vehicle Contributing Factors

Complementary Data
Methodology
Time-Series Regression Models
Accounting for I/M Program Jurisdiction
Dependent Variable
Exogenous Variables
Panel I
Variable name | Description |
---|---|
Program characteristics | |
Program | Does this state have a program in this year? (, ) |
Program_Repeal | Was this program repealed in this year? (, ) |
Program_Ever | Did this jurisdiction ever have a program? (, ) |
Demographics | |
Population | Statewide population (×100,000) |
VMT | Statewide total passenger VMT (billions) |
Prop_Rural_VMT | Fraction of VMT estimated to be on rural roads |
Registered_Vehicles | Number of registered passenger vehicles in state (×100,000) |
VMT_Per_Vehicle | Statewide mean annual VMT per vehicle (×1,000 mi) |
Veh_Per_Capita | Statewide mean number of registered passenger vehicles per capita |
Accident data | |
Fatal_Accidents | Total number of fatal accidents |
Driver_Age | Mean age of all drivers involved in fatal accidents |
Median_Income | Median statewide income |
Fatalities | Total number of road fatalities |
Vehicle_Age | Mean age of vehicles in fatal accidents |
Vehicles_Per_Accident | Mean number of vehicles in each fatal accident |
Lanes | Mean number of lanes at all accident sites |
Speed_Limit | Mean speed limit (mi/h) at all accident sites |
Prop_DUI | Fraction of accidents with at least one recorded DUI |
Prop_Speeding | Fraction of accidents where at least one vehicle was speeding |
Prop_VehCF | Fraction of accidents with at least one “vehicle contributing factor” |
Prop_Weather | Fraction of accidents in inclement weather |
Prop_Surface | Fraction of accidents with inclement surface conditions |
Note: VMT = vehicle miles traveled.
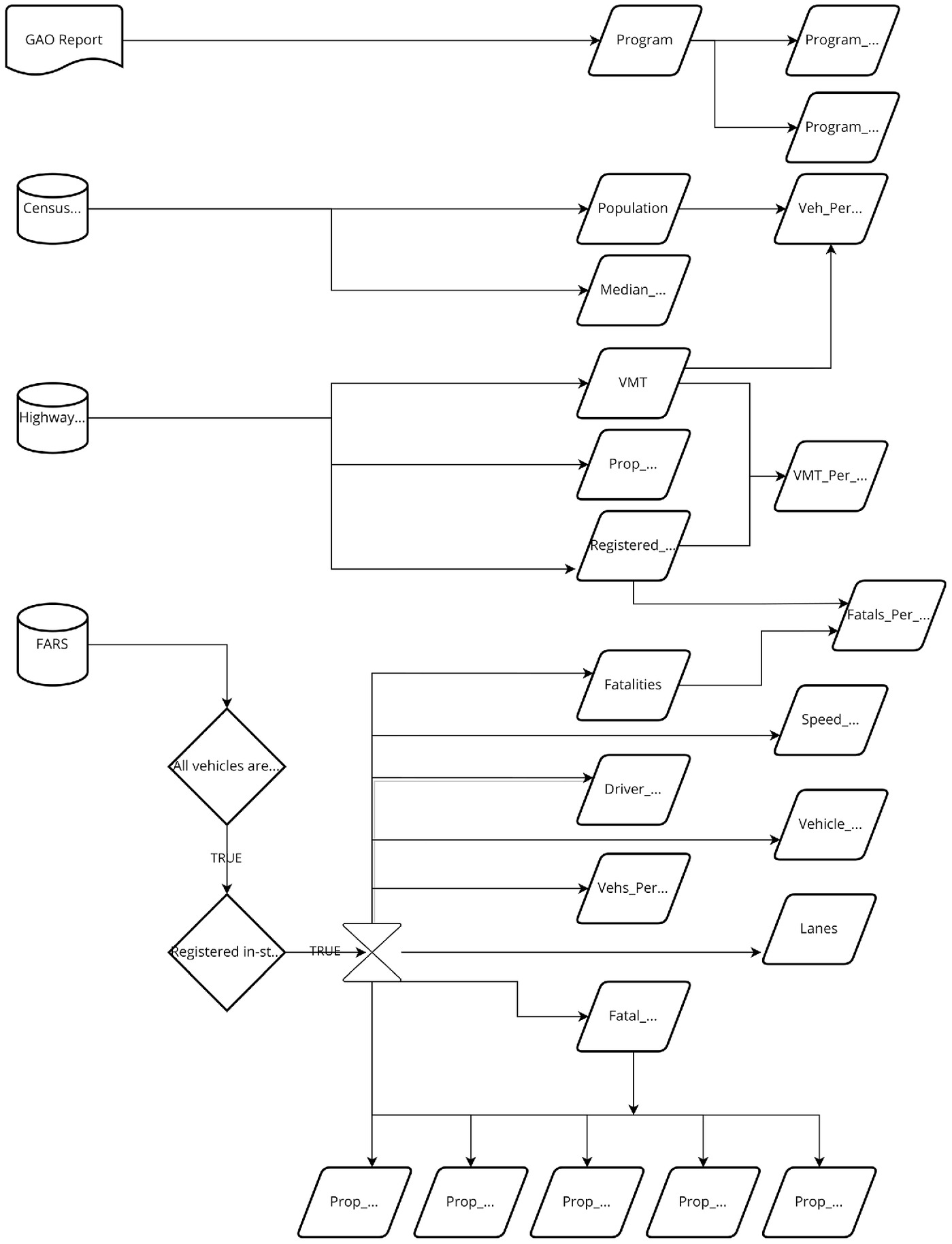
Panel II
Variable name | Description |
---|---|
Demographics | |
GDP | Statewide average GDP per capita ($2017) |
Pop.density | Statewide population density () |
Disposable.income | Median statewide disposable income ($2017) |
Driver_per_capita | Number of licensed drivers per capita |
Democrat | Legislative party in power (, ) |
Road characteristics | |
Area | State area () |
Road.length | Total length of road (mi) |
Road.density | State road density () |
Highway_expend_perCap | State highway expenditure ($2017) per capita |
Precipitation | Statewide average annual precipitation (in.) |
Note: GDP = gross domestic product.
Variable Transformation
Fixed-Effects Regressions
Supplementary Regressions
Two-Stage Least-Squares Regression
Vehicle Contributing Factors Data
Results
Fixed-Effects Regressions
Variable | Dependent variable | |
---|---|---|
Fatals_Per_100k_Veha | ||
Model I | Model II | |
Program | (0.017)* | (0.026)** |
Program_Repeal | (0.035) | (0.053) |
Populationa | (0.034)*** | — |
Driver_Agea | (0.080)*** | 0.055 (0.101) |
Median_Incomea | 0.038 (0.078) | 0.105 (0.104) |
Vehicle_Agea | 0.145 (0.048)*** | 0.344 (0.070)*** |
Vehicles_Per_Accidenta | 0.523 (0.116)*** | 0.175 (0.144) |
Lanesa | 0.032 (0.047) | (0.059)** |
Speed_Limita | 0.119 (0.095) | 0.290 (0.131)** |
Prop_DUIa | 0.213 (0.051)*** | 0.326 (0.076)*** |
Prop_Speedinga | (0.043)*** | (0.055) |
Prop_VehCFa | (0.156) | (0.242) |
Prop_Weathera | 0.121 (0.061)** | 0.076 (0.067) |
Prop_Surfacea | (0.097) | 0.084 (0.122) |
Veh_Per_Capitaa | (0.084)*** | (0.106)*** |
Prop_Rural_VMTa | — | 0.460 (0.144)*** |
GDPa | — | 0.470 (0.073)*** |
Highway_expend_perCapa | — | (0.020) |
Precipitationa | — | 0.021 (0.025) |
Democrat | — | (0.011)*** |
0.584 | 0.609 | |
F-statistic | 70.291 | 38.469 |
Observations | 2,244 | 1,224 |
Note: DUI = driving under the influence; GDP = gross domestic product; and VMT = vehicle miles traveled. *; **; and ***.
Supplementary Regressions
Two-Stage Least-Squares Regression
Variable | Dependent variable |
---|---|
Fatals_Per_100k_Veha | |
Program | (0.11)*** |
Program_Repeal | (0.06)*** |
Populationa | (0.04)*** |
Driver_Agea | (0.12) |
Median_Incomea | (0.09) |
Vehicle_Agea | 0.17 (0.05)*** |
Vehicles_Per_Accidenta | 0.58 (0.13)*** |
Lanesa | 0.02 (0.05) |
Speed_Limita | 0.25 (0.11)** |
Prop_VehCFa | (0.18) |
Prop_Weathera | 0.28 (0.08)*** |
Prop_Surfacea | (0.11)** |
Veh_Per_Capitaa | (0.10)*** |
0.88 | |
Number of observations | 2,244 |
Diagnostic test statistics | |
Weak instruments | 26.447*** |
Durbin–Wu–Hausman | 9.379** |
Sargan | 10.695** |
Note: **; and ***.
Vehicle Contributing Factors Data
Variable | Dependent variable | |
---|---|---|
Fatal accidents with contributing factor | ||
Proportiona | Counta | |
Program | (0.002)*** | (0.081)*** |
Program_Repeal | (0.005) | (0.171) |
Populationa | (0.005) | 0.969 (0.170)*** |
Driver_Agea | (0.011) | (0.385) |
Median_Incomea | 0.010 (0.011) | 3.059 (0.375)*** |
Vehicle_Agea | 0.036 (0.007)*** | 0.667 (0.230)*** |
Vehicles_Per_Accidenta | (0.016) | (0.559) |
Lanesa | (0.006)** | (0.225)*** |
Speed_Limita | (0.013) | 1.108 (0.456)** |
Prop_DUIa | 0.023 (0.007) | 0.256 (0.245)*** |
Prop_Speedinga | 0.013 (0.006)** | 1.724 (0.207)*** |
Prop_Weathera | 0.009 (0.009) | 0.188 (0.296) |
Prop_Surfacea | 0.022 (0.013)* | 0.802 (0.466)* |
Veh_Per_Capitaa | (0.014)** | 1.071 (0.483)** |
Fatals_Per_100k_Vehiclesa | (0.003) | 0.254 (0.104)** |
Observations | 2,244 | 2,244 |
0.038 | 0.096 | |
F-statistic | 5.667 | 15.172 |
Note: DUI = driving under the influence. *; **; and ***.
Discussion and Conclusions
Influence of Vehicle, Infrastructure, and Traffic Characteristics
Vehicle Contributing Factors
Policy Implications and Scope for Future Work
Appendix I. List of Abbreviations
Abbreviation | Definition |
---|---|
2SLS | Two-stage least-squares (regression) |
ADAS | Advanced driver-assistance systems |
ATE | Average treatment effect |
AV | Autonomous vehicle |
BTS | (US) Bureau of Transportation Statistics |
CDC | US Centers for Disease Control |
DOT | (State) Department of Transportation |
DUI | Driving under the influence (of alcohol, drugs, for example) |
EPA | (US) Environmental Protection Agency |
FARS | Fatality Analysis Reporting System |
FE | Fixed effects |
FHWA | (US) Federal Highway Administration |
FMVSS | Federal Motor Vehicle Safety Standards |
GAO | (US) Government Accountability Office |
I/M program | (Vehicle) inspection and maintenance program |
NASS-GES | National Automotive Sampling System–General Estimation System |
NCEI | National Centers for Environmental Information |
NHTSA | National Highway Traffic Safety Administration |
NOAA | National Oceanic and Atmospheric Administration |
PennDOT | Pennsylvania Department of Transportation |
USDOT | US Department of Transportation |
VIF | Variance inflation factor |
VIU | Vehicle-in-use (standard) |
VMT | Vehicle miles traveled (usually annual) |
Appendix II. FARS Data Summaries
Year | Total | Passenger, registered in state | ||||
---|---|---|---|---|---|---|
Accidents | Vehicles | Fatalities | Accidents | Vehicles | Fatalities | |
1975 | 39,161 | 55,534 | 44,525 | 32,346 | 41,926 | 36,998 |
1976 | 39,747 | 56,084 | 45,523 | 32,664 | 42,132 | 37,677 |
1977 | 42,211 | 60,516 | 47,878 | 34,252 | 44,509 | 39,110 |
1978 | 44,433 | 64,144 | 50,331 | 36,077 | 47,036 | 41,163 |
1979 | 45,223 | 64,762 | 51,093 | 36,429 | 47,129 | 41,529 |
1980 | 45,284 | 63,485 | 51,091 | 36,266 | 46,322 | 41,307 |
1981 | 44,000 | 62,699 | 49,301 | 35,253 | 45,708 | 39,795 |
1982 | 39,092 | 56,455 | 43,945 | 31,201 | 40,138 | 35,356 |
1983 | 37,976 | 55,106 | 42,589 | 30,220 | 38,992 | 34,181 |
1984 | 39,631 | 57,972 | 44,257 | 31,373 | 40,687 | 35,326 |
1985 | 39,196 | 58,271 | 43,825 | 31,008 | 40,830 | 34,884 |
1986 | 41,090 | 60,792 | 46,087 | 33,019 | 43,446 | 37,339 |
1987 | 41,438 | 61,836 | 46,390 | 33,527 | 44,508 | 37,789 |
1988 | 42,130 | 62,703 | 47,087 | 34,410 | 45,640 | 38,780 |
1989 | 40,741 | 60,870 | 45,582 | 33,498 | 44,913 | 37,715 |
1990 | 39,836 | 59,292 | 44,599 | 32,667 | 43,541 | 36,748 |
1991 | 36,937 | 54,795 | 41,508 | 30,312 | 40,292 | 34,278 |
1992 | 34,942 | 52,227 | 39,250 | 28,916 | 38,888 | 32,689 |
1993 | 35,780 | 53,777 | 40,150 | 29,648 | 39,636 | 33,401 |
1994 | 36,254 | 54,911 | 40,716 | 29,848 | 40,399 | 33,706 |
1995 | 37,241 | 56,524 | 41,817 | 30,795 | 41,817 | 34,745 |
1996 | 37,494 | 57,347 | 42,065 | 31,153 | 42,361 | 35,113 |
1997 | 37,324 | 57,060 | 42,013 | 31,061 | 42,284 | 35,154 |
1998 | 37,107 | 56,922 | 41,501 | 30,722 | 42,124 | 34,481 |
1999 | 37,140 | 56,820 | 41,717 | 30,813 | 42,086 | 34,687 |
2000 | 37,526 | 57,594 | 41,945 | 31,076 | 42,441 | 34,920 |
2001 | 37,862 | 57,918 | 42,196 | 31,290 | 42,663 | 35,083 |
2002 | 38,491 | 58,426 | 43,005 | 31,690 | 43,033 | 35,610 |
2003 | 38,477 | 58,877 | 42,884 | 31,494 | 42,836 | 35,259 |
2004 | 38,444 | 58,729 | 42,836 | 31,288 | 42,384 | 35,027 |
2005 | 39,252 | 59,495 | 43,510 | 31,489 | 42,316 | 35,151 |
2006 | 38,648 | 58,094 | 42,708 | 31,040 | 41,179 | 34,525 |
2007 | 37,435 | 56,253 | 41,259 | 29,900 | 39,715 | 33,160 |
2008 | 34,172 | 50,660 | 37,423 | 26,961 | 35,296 | 29,693 |
2009 | 30,862 | 45,540 | 33,883 | 24,725 | 32,487 | 27,330 |
2010 | 30,296 | 44,862 | 32,999 | 24,188 | 31,715 | 26,544 |
2011 | 29,867 | 44,119 | 32,479 | 23,738 | 31,032 | 25,973 |
2012 | 31,006 | 45,960 | 33,782 | 24,765 | 32,271 | 27,151 |
2013 | 30,202 | 45,101 | 32,893 | 24,111 | 31,590 | 26,426 |
2014 | 30,056 | 44,950 | 32,744 | 24,089 | 31,651 | 26,391 |
2015 | 32,538 | 49,478 | 35,484 | 26,135 | 34,778 | 28,703 |
2016 | 34,748 | 52,714 | 37,806 | 27,768 | 37,178 | 30,408 |
2017 | 34,247 | 52,645 | 37,133 | 27,317 | 36,732 | 29,804 |
2018 | 33,654 | 51,872 | 36,560 | 26,721 | 35,929 | 29,230 |
State (FIPS) | Total | Passenger, registered in state | ||||
---|---|---|---|---|---|---|
Accidents | Vehicles | Fatalities | Accidents | Vehicles | Fatalities | |
Alabama (01) | 876 | 1,321 | 953 | 714 | 942 | 785 |
Alaska (02) | 69 | 104 | 80 | 57 | 74 | 67 |
Arizona (04) | 916 | 1,404 | 1,010 | 573 | 795 | 643 |
Arkansas (05) | 472 | 735 | 516 | 356 | 478 | 389 |
California (06) | 3,259 | 4,986 | 3,563 | 2,701 | 3,708 | 2,989 |
Colorado (08) | 588 | 897 | 632 | 471 | 608 | 507 |
Connecticut (09) | 276 | 417 | 294 | 209 | 279 | 224 |
Delaware (10) | 104 | 168 | 111 | 76 | 99 | 78 |
District of Columbia (11) | 30 | 44 | 31 | 12 | 13 | 12 |
Florida (12) | 2,915 | 4,564 | 3,133 | 2,395 | 3,246 | 2,595 |
Georgia (13) | 1,407 | 2,165 | 1,504 | 1,129 | 1,495 | 1,214 |
Hawaii (15) | 110 | 157 | 117 | 90 | 110 | 97 |
Idaho (16) | 212 | 316 | 231 | 148 | 186 | 160 |
Illinois (17) | 948 | 1,481 | 1,031 | 751 | 1,040 | 819 |
Indiana (18) | 774 | 1,218 | 858 | 625 | 841 | 694 |
Iowa (19) | 291 | 469 | 318 | 221 | 290 | 244 |
Kansas (20) | 366 | 568 | 404 | 266 | 343 | 296 |
Kentucky (21) | 664 | 1,033 | 724 | 542 | 713 | 597 |
Louisiana (22) | 716 | 1,070 | 768 | 599 | 777 | 645 |
Maine (23) | 128 | 179 | 137 | 99 | 121 | 105 |
Maryland (24) | 474 | 741 | 501 | 368 | 490 | 391 |
Massachusetts (25) | 343 | 491 | 360 | 277 | 347 | 291 |
Michigan (26) | 905 | 1,480 | 974 | 778 | 1,115 | 842 |
Minnesota (27) | 349 | 538 | 381 | 286 | 385 | 316 |
Mississippi (28) | 597 | 898 | 664 | 497 | 629 | 554 |
Missouri (29) | 848 | 1,344 | 921 | 644 | 888 | 709 |
Montana (30) | 168 | 214 | 182 | 123 | 146 | 134 |
Nebraska (31) | 201 | 353 | 230 | 154 | 235 | 177 |
Nevada (32) | 300 | 452 | 330 | 223 | 302 | 245 |
New Hampshire (33) | 134 | 193 | 147 | 92 | 116 | 104 |
New Jersey (34) | 525 | 782 | 564 | 412 | 539 | 446 |
New Mexico (35) | 350 | 520 | 391 | 240 | 302 | 267 |
New York (36) | 889 | 1,291 | 943 | 701 | 891 | 753 |
North Carolina (37) | 1,321 | 2,065 | 1,437 | 1,100 | 1,514 | 1,207 |
North Dakota (38) | 95 | 144 | 105 | 63 | 77 | 72 |
Ohio (39) | 996 | 1,576 | 1,068 | 822 | 1,124 | 887 |
Oklahoma (40) | 603 | 975 | 655 | 466 | 658 | 513 |
Oregon (41) | 450 | 665 | 506 | 298 | 373 | 333 |
Pennsylvania (42) | 1,103 | 1,686 | 1,190 | 873 | 1,170 | 946 |
Rhode Island (44) | 56 | 82 | 59 | 35 | 40 | 35 |
South Carolina (45) | 970 | 1,475 | 1,037 | 799 | 1,052 | 852 |
South Dakota (46) | 110 | 148 | 130 | 82 | 97 | 102 |
Tennessee (47) | 974 | 1,518 | 1,041 | 757 | 1,041 | 812 |
Texas (48) | 3,305 | 5,222 | 3,642 | 2,690 | 3,713 | 2,994 |
Utah (49) | 237 | 376 | 260 | 175 | 242 | 194 |
Vermont (50) | 60 | 86 | 68 | 47 | 58 | 52 |
Virginia (51) | 778 | 1,154 | 820 | 627 | 813 | 661 |
Washington (53) | 497 | 762 | 546 | 403 | 553 | 449 |
West Virginia (54) | 265 | 409 | 294 | 192 | 249 | 214 |
Wisconsin (55) | 530 | 799 | 588 | 408 | 548 | 457 |
Wyoming (56) | 100 | 137 | 111 | 55 | 64 | 61 |
Note: FIPS = Federal Information Processing Standards.
Appendix III. Vehicle Contributing Factors
Contributing factor | FARS data code | ||
---|---|---|---|
1975–1981 | 1982–2009 | 2010–2017 | |
Tires | 1 | 1 | 1 |
Brake system | 2 | 2 | 2 |
Steering system | 3 | 3 | 3 |
Suspension | 4 | 4 | 4 |
Power train | 5 | 5 | 5 |
Exhaust | 6 | 6 | 6 |
Headlights | 7 | 7 | 7 |
Signal lights | 8 | 8 | 8 |
Other lights | 9 | 9 | 9 |
Mirrors | 11 | 11 | 12 |
Wipers | 12 | 12 | 10 |
Body | 14 | 14 | 14 |
Trailer hitch, safety chains | 15 | 15 | 15 |
Wheels | 1 | 16 | 11 |
Safety systems | — | 17, 19 | 16 |
Other vehicle defects | — | 10, 13,18 | 17, 97 |

Appendix IV. Panel Development
Regressors
Mean Vehicle and Driver Ages
Number of Roadway Lanes at Accident Site
Median Income
Vehicle Miles Traveled and Roadway Types
Vehicle Contributing Factors
DUI, Speeding, and Inclement Weather
I/M Program Presence and Repeal
Road Length
Area and Density
State Highway Expenditure
State Legislature Political Party
Licensed Drivers
Precipitation
Data from Commercial and Out-of-State Vehicles

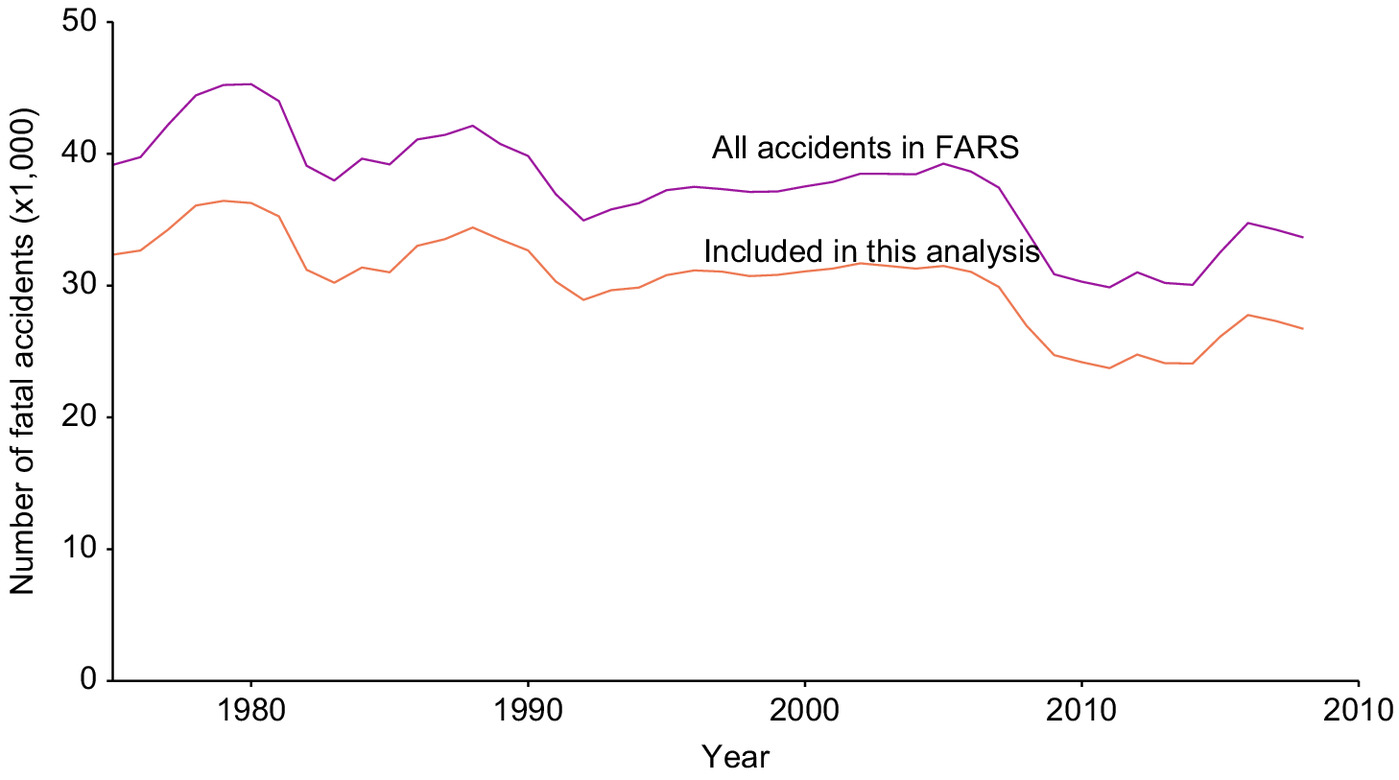
Appendix V. Summary Statistics, Data Panels
Variable | Minimum | Maximum | Mean | Standard deviation | Median |
---|---|---|---|---|---|
Program | 0 | 1 | 0.412 | 0.492 | 0 |
Program_Repeal | 0 | 1 | 0.008 | 0.087 | 0 |
Program_Ever | 0 | 1 | 0.627 | 0.484 | 1 |
Population () | 376.170 | 39,557.045 | 5,313.190 | 5,948.093 | 3,591.057 |
VMT (million) | 2,527 | 348,432 | 47,161.525 | 51,583.483 | 32,178 |
Prop_Rural_VMT | 0 | 0.858 | 0.457 | 0.188 | 0.470 |
Registered_Vehicles () | 142.717 | 20,037.727 | 2,470.443 | 2,745.115 | 1,752.886 |
Fatal_Accidents | 3 | 4,104 | 595.928 | 625.827 | 444 |
Driver_Age | 26.500 | 54.674 | 38.501 | 3.548 | 38.685 |
Median_Income | 0.603 | 1.487 | 0.995 | 0.159 | 0.979 |
Fatalities | 4 | 4,631 | 668.600 | 704.045 | 498.500 |
Vehicle_Age | 3.024 | 15.603 | 9.184 | 1.956 | 8.905 |
Vehicles_Per_Accident | 1 | 1.605 | 1.299 | 0.071 | 1.303 |
Lanes | 1.939 | 4.857 | 2.364 | 0.315 | 2.299 |
Speed_Limit | 25 | 62.567 | 47.283 | 5.202 | 48.091 |
Prop_DUI | 0 | 0.712 | 0.370 | 0.107 | 0.367 |
Prop_Speeding | 0 | 0.685 | 0.303 | 0.102 | 0.301 |
Prop_VehCF | 0 | 0.224 | 0.025 | 0.028 | 0.018 |
Prop_Weather | 0 | 1 | 0.163 | 0.092 | 0.148 |
Prop_Surface | 0 | 0.578 | 0.195 | 0.076 | 0.190 |
VMT_Per_Vehicle | 9,414.072 | 72,133.592 | 20,571.154 | 7,393.444 | 18,903.095 |
Veh_Per_Capita | 0.129 | 0.740 | 0.469 | 0.089 | 0.479 |
Note: DUI = driving under the influence; and VMT = vehicle miles traveled.
Variable | Minimum | Maximum | Mean | Standard deviation | Median |
---|---|---|---|---|---|
Program | 0 | 1 | 0.382 | 0.486 | 0 |
Program_Repeal | 0 | 1 | 0.006 | 0.075 | 0 |
Program_Ever | 0 | 1 | 0.627 | 0.484 | 1 |
Population () | 401.851 | 39,399.349 | 5,558.172 | 6,243.947 | 3,767.109 |
VMT (millions) | 2,665 | 343,701.646 | 51,027.006 | 54,783.206 | 35,025 |
Prop_Rural_VMT | 0 | 0.812 | 0.440 | 0.190 | 0.446 |
Registered_Vehicles () | 160.090 | 19,972.837 | 2,477.761 | 2,777.318 | 1,751.166 |
Fatal_Accidents | 3 | 4,054 | 570.642 | 605.963 | 420 |
Driver_Age | 26.500 | 54.674 | 39.525 | 3.402 | 39.833 |
Median_Income | 0.603 | 1.487 | 1 | 0.158 | 0.982 |
Fatalities | 4 | 4,569 | 636.724 | 678.814 | 468.500 |
Vehicle_Age | 5.011 | 15.603 | 9.843 | 1.933 | 9.570 |
Vehicles_Per_Accident | 1 | 1.605 | 1.300 | 0.071 | 1.305 |
Lanes | 1.939 | 4.857 | 2.378 | 0.318 | 2.317 |
Speed_Limit | 25 | 62.567 | 47.577 | 5.295 | 48.368 |
Prop_DUI | 0 | 0.712 | 0.365 | 0.097 | 0.356 |
Prop_Speeding | 0 | 0.685 | 0.303 | 0.105 | 0.302 |
Prop_VehCF | 0 | 0.194 | 0.018 | 0.024 | 0.010 |
Prop_Weather | 0 | 1 | 0.179 | 0.108 | 0.153 |
Prop_Surface | 0 | 0.561 | 0.190 | 0.076 | 0.185 |
VMT_Per_Vehicle | 9,548.243 | 72,133.592 | 22,323.416 | 7,572.760 | 21,360.633 |
Veh_Per_Capita | 0.129 | 0.740 | 0.451 | 0.092 | 0.453 |
Area () | 0.018 | 172.334 | 19.301 | 24.854 | 14.575 |
Road.length () | 0.110 | 31.432 | 7.831 | 5.272 | 7.712 |
Road.density | 0.010 | 8.670 | 0.744 | 1.050 | 0.610 |
GDP | 14,116 | 177,559 | 38,504.507 | 18,048.217 | 38,001 |
Pop.density | 0.235 | 3,926.023 | 122.806 | 480.470 | 33.237 |
Disposable.income | 6,458 | 67,202 | 27,940.438 | 12,440.479 | 28,419.500 |
Highway_expend_perCap | 95 | 2,273 | 402.823 | 250.977 | 341 |
Driver per capita | 0.511 | 0.878 | 0.677 | 0.053 | 0.681 |
Precipitation | 4.970 | 77.290 | 37.221 | 14.682 | 39.545 |
Democrat | 0 | 1 | 0.435 | 0.496 | 0 |
Note: DUI = driving under the influence; GDP = gross domestic product; and VMT = vehicle miles traveled.
Appendix VI. VIF Tables: FE Models I and II
Variable | VIF | |
---|---|---|
Program_Repeal | 1.019 | 0.019 |
Prop_Speedinga | 1.234 | 0.190 |
Program | 1.363 | 0.266 |
Prop_VehCFa | 1.458 | 0.314 |
Prop_DUIa | 1.543 | 0.352 |
Median_Incomea | 1.667 | 0.400 |
Vehicles_Per_Accidenta | 1.712 | 0.416 |
Lanesa | 1.788 | 0.441 |
Prop_Weathera | 1.917 | 0.478 |
Prop_Surfacea | 2.031 | 0.508 |
Populationa | 2.671 | 0.626 |
Driver_Agea | 3.150 | 0.683 |
Vehicle_Agea | 3.647 | 0.726 |
Veh_Per_Capitaa | 3.690 | 0.729 |
Speed_Limita | 4.006 | 0.750 |
Prop_Rural_VMTa | 5.702 | 0.825 |
VMT_Per_Vehiclea | 6.503 | 0.846 |
Note: VIF = variance inflation factors; and VMT = vehicle miles traveled.
Variable | Iteration | |||
---|---|---|---|---|
(1)a | (2)a | |||
VIF | VIF | |||
Program_Repeal | 1.047 | 0.044 | 1.040 | 0.038 |
Democrat | 1.346 | 0.257 | 1.245 | 0.197 |
Prop_Speedinga | 1.434 | 0.303 | 1.402 | 0.287 |
Prop_VehCFa | 1.699 | 0.411 | 1.651 | 0.394 |
Vehicles_Per_Accidenta | 1.916 | 0.478 | 1.593 | 0.372 |
Prop_Weathera | 2.039 | 0.509 | 2.000 | 0.500 |
Lanesa | 2.046 | 0.511 | 1.873 | 0.466 |
Prop_DUIa | 2.088 | 0.521 | 1.965 | 0.491 |
Program_Ever | 2.157 | 0.536 | 2.119 | 0.528 |
Precipitationa | 2.415 | 0.586 | 1.770 | 0.435 |
Median_Incomea | 2.464 | 0.594 | 2.039 | 0.510 |
Prop_Surfacea | 2.507 | 0.601 | 2.232 | 0.552 |
Program | 2.592 | 0.614 | 2.237 | 0.553 |
Highway_expend_perCapa | 3.011 | 0.668 | 2.062 | 0.515 |
Driver_Agea | 4.247 | 0.765 | 2.990 | 0.666 |
Vehicle_Agea | 4.474 | 0.776 | 3.568 | 0.720 |
Veh_Per_Capitaa | 5.652 | 0.823 | 4.654 | 0.785 |
Prop_Rural_VMTa | 7.580 | 0.868 | 4.961 | 0.798 |
Speed_Limita | 7.611 | 0.869 | 3.464 | 0.711 |
GDPa | 8.919 | 0.888 | 3.865 | 0.741 |
VMT_Per_Vehiclea | 9.235 | 0.892 | 7.434 | 0.865 |
Populationa | 11.909 | 0.916 | NA | |
Road.densitya | 14.383 | 0.930 | NA | |
Disposable.incomea | 16.843 | 0.941 | NA | |
Road.lengtha | 24.688 | 0.959 | NA | |
Areaa | 26.078 | 0.962 | NA |
Note: GDP = gross domestic product; VIF = variance inflation factor; and VMT = vehicle miles traveled.
Appendix VII. Dependent Variable Normalization
Variable | Dependent variable: roadway fatalities per | ||
---|---|---|---|
100k vehiclesa | Billion VMTa | 100k populationa | |
Program | (0.017)* | (0.016)** | (0.016) |
Program_Repeal | (0.035) | (0.033) | (0.034) |
Populationa | (0.034)*** | (0.032)*** | (0.033)*** |
Driver_Agea | (0.080)*** | (0.074)*** | (0.077)*** |
Median_Incomea | 0.038 (0.078) | (0.072) | 0.090 (0.076) |
Vehicle_Agea | 0.145 (0.048)*** | 0.086 (0.045)* | 0.148 (0.047)*** |
Vehicles_Per_Accidenta | 0.523 (0.116)*** | 0.315 (0.107)*** | 0.458 (0.112)*** |
Lanesa | 0.032 (0.047) | (0.044) | 0.027 (0.045) |
Speed_Limita | 0.119 (0.095) | 0.069 (0.088) | 0.082 (0.092) |
Prop_DUIa | 0.213 (0.051)*** | 0.069 (0.047) | 0.182 (0.049)*** |
Prop_Speedinga | (0.043)*** | (0.040) | (0.042)*** |
Prop_VehCFa | (0.156) | (0.145)* | (0.151) |
Prop_Weathera | 0.121 (0.061)** | 0.071 (0.057) | 0.086 (0.060) |
Prop_Surfacea | (0.097) | (0.090) | (0.094) |
Veh_Per_Capitaa | (0.084)*** | (0.078) | 0.043 (0.081) |
0.584 | 0.896 | 0.901 | |
Observations | 2,244 | 2,244 | 2,244 |
Note: *; **; and ***.
Data Availability Statement
Acknowledgments
References
Information & Authors
Information
Published In
Copyright
History
ASCE Technical Topics:
- Analysis (by type)
- Business management
- Construction engineering
- Construction management
- Engineering fundamentals
- Highway and road management
- Highway transportation
- Highways and roads
- Infrastructure
- Inspection
- Passengers
- Practice and Profession
- Public administration
- Public health and safety
- Public transportation
- Regression analysis
- Safety
- Statistical analysis (by type)
- Traffic accidents
- Traffic engineering
- Traffic management
- Traffic safety
- Transportation engineering
- Vehicle impacts