Informing Just Design with Place-Based Racial History
Publication: ASCE OPEN: Multidisciplinary Journal of Civil Engineering
Volume 1, Issue 1
Abstract
Enhanced designs of the built environment are particularly urgent for frontline communities, who experience the first and worst of climate change. A climate adaptation approach that prioritizes need-based distributive justice, the allocation of resources to meet the basic conditions necessary for continued community existence, may empower frontline communities in the resource allocation process. Need-based distributive justice is often viewed as a supplement to other universal principles of distributive justice, such as merit—the allocation of resources according to individual contributions. The overlooked need principle, however, is especially important because it can address the deficit of resources that frontline communities experience. Frontline communities disproportionately comprise members of racial minority groups, whose history of marginalization intertwines with past and present decisions that contribute to the position of communities on the frontlines of climate change. Yet, racial history is not commonplace in discussions about climate adaptation. Therefore, this research considers ways to introduce place-based racial history in climate adaptation planning. In an online experiment (n = 1,731), all participants were shown a video describing how updated infrastructure (e.g., cooling pavements) could address present and future heat vulnerability in a frontline community. The intervention, a 50-s addition to the video, described a highway in the community, which bisected and is associated with the decline of a historically Black neighborhood. Participants then shared their support for or opposition to a need-based distributive justice strategy for the project. The brief intervention tested did not significantly (P = 0.07) increase support for a need-based distributive justice strategy. However, additional analysis revealed that this relationship was mediated by the degree to which participants perceived the racial history presented to be relevant to the stated problem. These results suggest that more in-depth racial history interventions should be tested for this purpose.
Introduction: Equitable Climate Adaptation Efforts in the United States Must Recognize the Impact of Systemic Racism
The built environment is a mechanism by which historic injustices exacerbate modern threats for communities of color. Inequitable design, maintenance, and investment have led to problems relating to water, transportation, and energy infrastructure around the United States. Climate adaptation and resilience strategies that do not address equity will reinforce these legacies of prejudice (Matin et al. 2018; Meerow and Newell 2019). For example, the detrimental effects of redlining are now being exacerbated by climate change. These cities are up to 7 °C warmer in the summer months than cities with a less history of these practices (Hoffman et al. 2020). Approaching climate adaptation in a way that accounts for this context and its present and future effects is urgent for frontline communities, who experience the first and worst of climate change.
The decision-making process that goes into adaptation planning is a process of design, defined broadly as changing things from an existing state into a preferred state (Simon 1988). Professional designers of the built environment—engineers, architects, and urban planners—address climate-related problems as a function of their job and possess technical expertise that serves their role as a decision-maker within a larger system. However, there is also vital information that is unknowable to professional designers without the assistance of frontline communities (IPCC 2022). Other forms of research have shown that frontline community members who have cultivated their lived experience into reflective commentary possess information that can transform broad technical solutions into culturally relevant, socially sustainable initiatives (Ayers and Forsyth 2009; Dodman and Mitlin 2013; Kirkby et al. 2018).
We refer to these community members in a new way, as frontline designers. Compared with terms like stakeholders, users, and subjects, a frontline designer better acknowledges the value of the lived experience of advocates from frontline communities and serves as one step in leveling unequal power dynamics between frontline designers and professional designers (Costanza-Chock 2020).
Each frontline community is unique, and a solution that works for one community is not necessarily scalable to other frontline communities. However, there are behavioral dynamics that frontline designers in the United States are likely to encounter as they advocate for their communities. Common barriers include a denial of contemporary systemic racism as a factor in their ability to adapt to climate change, and concurrently, a belief in meritocratic ideology that can be blind to context and obstruct need-based requests for assistance. The first of these barriers has been addressed across the social sciences. Color-blind ideology for many years was popularly viewed as an antidote to racism (Lipsitz 2011). These ideas persist in decision-making for the built environment in the ideas that anything related to race—including the context of racial history—can be better represented by proxy variables such as zip code or socioeconomic status (Center on Society and Health 2016; Rothstein 2017). The second of these barriers, a meritocratic preference, is doubly problematic when paired with color-blind ideology that removes the context that illuminates the systemic nature of racial inequities. Meritocratic values are deeply embedded in US culture and have also been documented as a sizable force within professional design fields such as engineering (Cech 2013). This directly relates to how favorably universal distributive justice strategies may be viewed in the public eye and in the professional decision-making contexts.
Public opinion matters especially in a context like the United States where over $1 trillion has been allocated to support infrastructure design and maintenance (DeFazio 2021; Yarmuth 2022). These federal funds are distributed to states where local officials are able to make decisions about which projects the funds will be allocated to. Constituents are able to make their perspectives known through public opinion surveys or by directly contacting political offices (Warshaw 2019; Vedachalam et al. 2014).
This project builds upon findings from psychology showing that a knowledge of place-based critical racial history can influence contemporary perceptions of systemic racism. By incorporating place-based critical racial history into a climate adaptation project context, this study examines the relationship between this factor and preferences for a need-based distributive justice strategy that could facilitate the efforts of frontline designers.
Theoretical Background and Development of the Hypothesis
Knowledge of Place-Based Racial History Can Increase a Recognition of Systemic Racism
In How Racism Takes Place, Lipsitz (2011) writes that “by examining residential and school segregation, mortgage and insurance redlining, taxation and transportation politics, or the location of environmental amenities and toxic hazards, we learn that race is produced by space, that it takes places for racism to take place”. Race and the built environment in the United States are inextricably linked because of explicit decisions made in history that continue to affect the present (Lipsitz 2011; Rothstein 2017). This, in addition to the gap between racial groups in perceptions of contemporary racism (Norton and Sommers 2011; Strickhouser et al. 2019), were motivation for the study of place-based critical history in the field of psychology. Place-based critical history is a narrative of history presented with the perspective of the present in mind (Bonam et al. 2019). Empirical work has used place-based critical racial history as a tool to increase the recognition of contemporary racism in the United States (Bonam et al. 2019; Coleman et al. 2019). This work builds on the Marley hypothesis literature, which posits that the relevance of racial identity and a knowledge of racial history are two factors that help explain the gap in perceptions of contemporary racism (Nelson et al. 2013). There are boundary conditions to the Marley hypothesis: if perceptions are being assessed at a limited number of institutional or geographic regions, the effects noted previously have not always been successfully replicated (Bonam et al. 2019; Strickhouser et al. 2019). Specifically, findings produced at separate academic institutions in the Midwest did not replicate to a single more racially diverse academic institution in the southeast United States. This is a factor that specific institutions need to be aware of when looking to apply the findings of the Marley hypothesis.
This study seeks to expand the work using place-based critical racial history as an intervention by focusing on how it may affect participants’ reactions to a climate adaptation design decision context. By expanding this work to focus on design, the operationalization of this concept will need to change. Specifically, the intervention will need to be much shorter to feasibly fit into a presentation on a climate adaptation project, where it is one of many factors competing for attention.
Many Types of Justice Have Relevancy in Decision-Making for the Built Environment—Distributive Justice Is One of the More Tangible
There are many types of justice that may work synergistically with a knowledge of place-based critical racial history to improve equity in the built environment. For example, Bozeman et al. (2022) explore how the complexities of resource, policy, and cultural needs overlap to move toward or away from systemic equity, which incorporates different aspects of distributive, procedural, and recognition justice. There is also restorative and intergenerational justice, which are highly relevant to climate conversations (Siders et al. 2021). These are promising areas for future work. Inspired by Lipsitz’s observation of the relationship between race and space, this study focuses on distributive justice, the allocation of benefits and burdens.
Increased Support for Need-Based Distributive Justice May Benefit Frontline Communities in Design
There are at least four principles of distributive justice that describe common ways in which people describe allocations of resources as fair or just; an individual will likely prefer different principles depending on the context (Deutsch 1975; Konow 2001). The principle of equality suggests that resources should be allocated equally among a population, the principle of equity suggests that resources should be allocated with regard to individual contributions, the principle of need suggests that resources should be allocated to prioritize those in need, and the principle of entitlement suggests that resources should be allocated by status (Gollwitzer and van Prooijen 2016). Equality implies that frontline communities will get an equal portion of resources, presumably less than they need to be successful in adapting to climate change because of the historical and complex factors that have systemically disadvantaged them. Next, it should be noted that the term equity has many definitions that are very different from each other (Pereira et al. 2017). Equity has been defined to mean impartial (Sen 2009). It has also been defined as broadly as distribution based on individual differences (Rawls 1999). By some, it is understood as equivalence of the outcome/input ratios, which is the concept that this study refers to as merit (Cook and Hegtvedt 1983). Merit is likely to be a direct reinforcer funneling attention and resources away from frontline communities that do not have as much financial capital to invest or as much temporal wealth to invest. However, merit framed in other ways may work to the benefit of frontline communities. For example, if a metric considered in distributive decision-making was focused on carbon footprint, frontline communities may fare better than communities with individuals who have a lot of disposable income to spend on consumeristic priorities. Lastly, need-based allocation strategies emphasize attention to those who are working at a disadvantage and what they need to be successful. What success means if defined quite differently based on the various strategies. For example, sufficientarianism, which focuses on whether individuals have enough of a resource, might be a less effective position for supporting frontline communities because what is needed as a bare minimum will be just as unpredictable and rapidly changing as the effects of climate change.
Equality and equity (i.e., merit) have received much more empirical attention than need and entitlement, both of which have been tied to the environmental justice movement (Gabriel and Bond 2019). It is possible to apply multiple principles in the same distributive justice effort. However, equality and need are often confounded, which makes them difficult to study together in an empirical manner (Hülle et al. 2018).
Need, particularly, has potential to be of help to frontline designers because it is “more fundamental than the other principles” in that it “refers to the basic conditions for human existence” (Traub and Kittel 2020). The question of what constitutes need has been a subject of debate. In a built environment context, clean air and water would almost certainly be included as needs, but is green space also included in this definition, given the empirical evidence on how it can impact well-being? (Clayton et al. 2016). There is no definitive answer to this question, and given the unique context and composition of each frontline community, it is perhaps more beneficial for these questions to be left to the discretion of frontline designers and professional designers in each context.
A popular theory associated with need-based distributive justice and climate adaptation is the Capabilities Approach, which emerged from a body of work by Sen and Nussbaum (Nussbaum 1988; Sen 2009; Traub and Kittel 2020). The Capabilities Approach is a framework that emphasizes the importance of well-being, which is defined by capabilities and functionings. Capabilities describe what is feasible and functions are capabilities that have been actualized. This work has been applied throughout the discourse on climate adaptation. For example, it was discussed as a method for articulating individual climate adaptation enablers and barriers to well-being (Kronlid 2014). It has also been lauded as offering “a particularly constructive way of understanding issues of vulnerability and impact” in a climate adaptation context (Schlosberg 2012). If methods like the Capabilities Approach are to be applied, there needs to be general buy-in that the need principle should be considered as a distributive justice strategy for climate adaptation and potentially prioritized over more popular principles of equality and equity to address the pressing challenges of frontline communities.
Research Question and Hypothesis
Research Question: How does the inclusion of place-based critical racial history affect support for a need-based distributive justice strategy in design for climate adaptation?
Hypothesis: People with more information about racial history will show stronger support for the need-based allocation of resources than people with less information about racial history.
Method
Study Design and Setting
This quantitative, two-cell experiment gathered data from the participants of Amazon Mechanical Turk (MTurk) living in the United States (Litman et al. 2017). As the use of MTurk for online studies has increased in popularity, so has the understanding of its benefits and concerns. For example, Aguinis et al. (2021) reviewed MTurk research across the field of management research and came up with best practices to avoid concerns affecting validity, such as participant inattention, self-misrepresentation, self-selection bias, vulnerability to web robots (bots), and social desirability bias. We followed the recommendations in this article to address as many of these concerns as possible.
In order to reduce variability in the interpretation of variables, the study context presented was urban heat vulnerability in Richmond, Virginia. RVAgreen 2050 is “Richmond’s equity-centered climate action and resilience planning initiative to reduce greenhouse gas emissions 45% by 2030, achieve net zero greenhouse gas emissions by 2050 and help the community adapt to Richmond’s climate impacts of extreme heat, precipitation, and flooding” (City of Richmond 2022). Because of these efforts, geographic information system maps of the city were already created with overlays that contain a variety of information relevant to climate equity (City of Richmond, Office of Sustainability 2021). Two overlays were used to develop the context for this study: Extreme Heat and Interstate Construction. Literature documenting the heat vulnerability challenges in the city and place-based racial history related to the construction of Interstate-95 (I-95) were used in the creation of a video vignette, which served as the independent variable (IV) (Evans 2015; Marquette 2020; Saverino et al. 2021).
Sample Size Determination
The sample size range for this study was determined a priori using G*Power 3.1 software (Faul et al. 2009). The pilot data indicated that a small effect size was likely (Cohen’s d = 0.1), so this study was designed to balance the anticipated small effect with the feasibility of collecting a large sample. In order to detect an effect of 0.1 with an alpha of 0.01, 95% power, and an allocation ratio of 1, the total sample size would need to be 7,130 (3,565 per group). A sample of 7,130 was beyond the constraints of this study. Therefore, the maximum effect size that we would expect to see based on the pilot data would be the upper range of a small effect (Cohen’s d = 0.2). With an effect size of 0.2, a sample size of N = 1,786 would have enough power (1 − β < 0.95) to detect an effect (α = 0.01). Therefore, we aimed for a participant enrollment threshold of N = 2,000.
Participants
A sample of 2,197 participants was enrolled from a general pollution subject pool in the United States via Amazon Mechanical Turk. A total of 336 (228 intervention, 108 control) participants were screened out for failing to pay attention to the independent variable video in the first few minutes of the study. A total of 46 (22 intervention, 24 control) additional participants dropped out before the end of the study and 84 (43 intervention, 41 control) participants were excluded based on preregistered criteria (Gordon and Klotz 2021). The independent variable attention check filtered out approximately twice as many participants from the intervention group than the control group. A supplementary analysis that compared the demographic proportions between the intervention and the control group did not detect that this had a notable effect on the data. However, it is possible that there are unmeasured explanations for the differential attrition, so the potential contributions of this are examined in the discussion. The final sample (N = 1,731) included 900 intervention group participants and 831 control group participants. The groups were proportional on all demographics including gender identity (50.7% women), age (M = 42.7, SD = 13.4), race (78.1% white), and formal education (39.5% had a bachelor’s degree).
When designing this study, there were two types of bias that were of particular concern: desirability bias and self-selection bias. Desirability bias is a type of bias that comes about as a result of participants wanting to answer in a way that is socially appropriate. With highly political issues like race and climate change, the risk of desirability bias will always be higher than that with less-politicized topics. In order to combat this bias, the anonymity of responses was emphasized and valid reasons to support all of the strategies were presented in the video. The possibility of self-selection bias was high due to differential attrition. To limit self-selection bias, there was no indication that racial topics were involved in the study prior to the intervention. However, the recruitment materials did indicate that the study involved decision-making for climate infrastructure adaptation, which could bias the results in favor of people who are interested in that topic.
Procedure
After consenting to participate, participants were randomly assigned to either the intervention or the control condition. The participants then viewed a video about a fictional climate adaptation project addressing heat vulnerability in the city of Richmond, Virginia. In the middle of the intervention group’s video, there was a 50-s clip detailing place-based racial history about the historically Black neighborhood of Jackson Ward. On the next page, all participants responded to an attention check on the video they viewed. The participants had the opportunity to go back and watch the video before submitting the question and were notified that if they did not answer the question correctly, they would be screened out of the survey. All participants who answered the attention check correctly proceeded to a question inquiring about their support for (or opposition to) a need-based distributive justice strategy. The need-based distributive justice strategy was represented visually and the participants were guided through understanding the elements on the map prior to answering the question. The participants also reported their support for (or opposition to) an equality-based strategy and a merit-based strategy, and then, they were asked to choose one of the strategies, asked a series of follow-up exploratory questions, and then asked to do a comprehension check. Finally, the participants reported their demographic information and reported any discrepancies that would make their data less usable before receiving compensation.
Primary Measure: A Need-Based Distributive Justice Strategy
The dependent variable (DV) was measured on a scale from 1 (Strongly oppose) to 6 (Strongly agree). Participants were asked the question “How much do you support or oppose this distribution of resources?” (Fig. 1). The distribution of resources for the need-based distributive justice strategy was represented by monetary allocations ranging from $100,000 to $300,000 based on current heat vulnerability estimates. The full visual for this measure can be found in the Supplemental Materials.
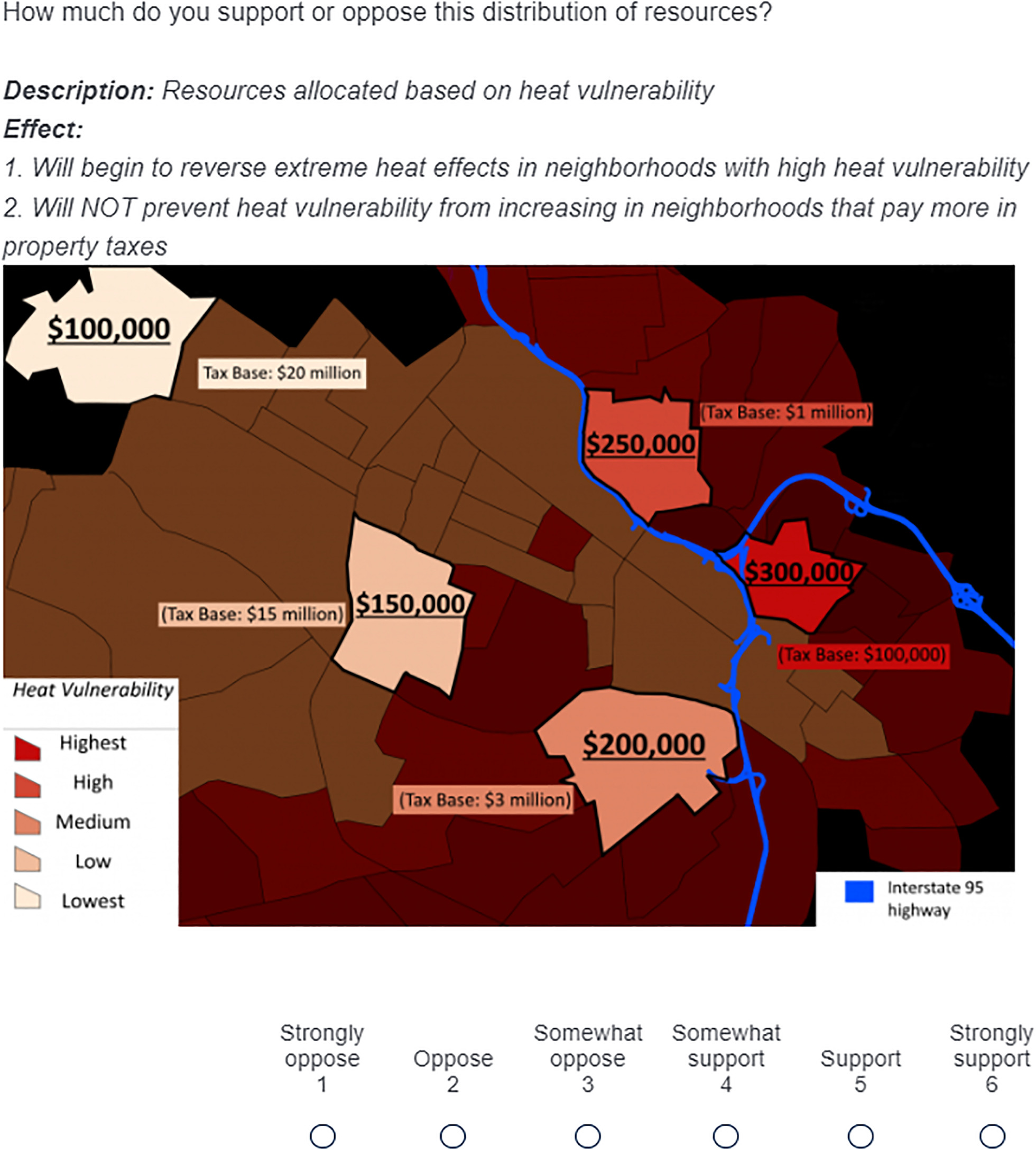
While single-item dependent variables are quite common for the support or oppose type of question that we asked in this study, there can be drawbacks to not using a multi-item measure. Most notably, there can be a reduced sensitivity, which makes it more difficult to detect whether the hypothesized differences are truly absent or simply not detectable with a single-item measure. However, this single question best represents how this design choice may be presented in practice. Additionally, there are several other constructs, including racial preferences, which are best measured with a single item (Axt 2018).
Supplementary Measures
Equality and Merit Strategies
In addition to the need-based strategy, equality and merit were also presented to establish clear comparison points for all participants. In the equality-based strategy, all neighborhoods were allocated $200,000. In the merit-based strategy, the allocations ranged from $100,000 to $300,000 based on the average property taxes collected from each neighborhood in the year prior. The full visuals for these measures can be found in the Supplemental Materials.
Vote
The participants were asked to select which of the three strategies they would vote for if they were to choose from these options. Because it is possible to support or oppose all three strategies equally, this question clarifies participants’ overall preferences.
Relevance Factors
Participants were asked to rate how important a series of factors were in determining which options to support or oppose (0 = completely irrelevant, 3 = completely relevant). The randomized list of factors included the following: racial history, general history, socioeconomic status, climate change, current heat vulnerability levels, how much you typically pay in property taxes, and where you imagined yourself living in this scenario. Additionally, among these questions, there was an attention check with the instructions: “Please select ‘Somewhat relevant,’” to encourage any distracted participants to re-engage with the survey.
Comprehension Check and Self-Reported Technical Difficulties
The comprehension check included two multiple-choice questions about key content in the study. The participants were also asked a series of questions about their attention to and technical experience with the study. The full text of these two multiple choice questions can be found in the Supplemental Materials.
Demographics and Participant Characteristics
In addition to the standard demographic questions (e.g., age, race, gender, education), the participants were asked about the familiarity with Richmond, Virginia, their voting habits, their field of employment, and their experiences with the race-related events of 2020. This information was used broadly to explore the data. In future studies, we may also include familiarity with distributive justice principles as a supplementary question, although we would expect the familiarity to be approximately equal across the randomly assigned groups.
Data Analysis
Table 1 summarizes the collected data and analyses used. The dependent variable means were compared using Welch’s test. Because variances are rarely equal in experimental data, we elected to use the Welch’s test in lieu of Student’s t-test. This may help reduce Type 1 error (Delacre et al. 2017; Rasch et al. 2011). Additionally, Welch’s test is robust to assumptions of normality, given a large enough sample size (Rochon et al. 2012). R packages used in the data analysis include dplyr, ggplot2, tidyr, ggpubr, broom, and rstatix (Kassambara 2020, 2021; Robinson et al. 2021; Wickham 2016, 2021; Wickham et al. 2021). As a supplementary analysis, we conducted a mediation analysis using the mediation package in R to better understand the role of a perceived relevance of racial history on the relationship between the independent and the dependent variable (Tingley et al. 2014).
Variable | Hypothesized variable | Supplementary data | |||
---|---|---|---|---|---|
Need | Equality | Merit | Vote | Relevance factors | |
Type | Likert 1 (strongly disagree)—6 (strongly agree) | Likert 1 (strongly disagree)—6 (strongly agree) | Likert 1 (strongly disagree)—6 (strongly agree) | Multiple choice | Likert 0–3 |
Data collected | |||||
Control (n = 831) | Mean: 4.57 SD: 1.25 Median: 5 Min: 1 Max: 6 | Mean: 3.46 SD: 1.39 Median: 4 Min: 1 Max: 6 | Mean: 2.28 SD: 1.28 Median: 2 Min: 1 Max: 6 | Need: n = 609 Equality: n = 162 Merit: n = 60 | Table 2 and Table |
Intervention (n = 900) | Mean: 4.65 SD: 1.22 Median: 5 Min: 1 Max: 6 | Mean: 3.48 SD: 1.35 Median: 4 Min: 1 Max: 6 | Mean: 2.13 SD: 1.19 Median: 2 Min: 1 Max: 6 | Need: n = 694 Equality: n = 163 Merit: n = 43 | |
Distributions | Fig. | Fig. | Fig. | — | Fig. |
Analysis | — | — | — | Not used in final analysis | — |
Results
Inclusion of Place-Based Critical Racial History in This Study Design Did Not Have a Statistically Significant Effect on the Support for a Need-Based Distributive Justice Strategy
We hypothesized that the group exposed to racial history would show stronger support for the need-based allocation of resources than the group not exposed to racial history. The degree of support for a need-based distributive justice strategy did not differ significantly according to a Welch’s t-test, t(1,710.8) = −1.45, P = 0.07 (Table 2). The Likert scale ranged from 1 (strongly oppose) to 6 (strongly support). On average, participants in the intervention group indicated a higher mean support score (M = 4.65, SD = 1.22) than the control group (M = 4.57, SD = 1.25). The 99% confidence interval for the effect of place-based racial history on support for a need-based distributive justice strategy is between-infinity and 0.05. These results do not support the hypothesis.
Distributive justice strategy | Intervention (n = 900) | Control (n = 831) | t(1,710.8) | P | Cohen d | 99% CI | |||
---|---|---|---|---|---|---|---|---|---|
M | SD | M | SD | LL | UL | ||||
Need | 4.65 | 1.22 | 4.57 | 1.25 | −1.45 | 0.073 | 0.07 | −∞ | 0.05 |
Note: 1 = strongly oppose; and 6 = strongly support.
Exploratory Analyses
A full report of the exploratory analyses can be found in the Supplemental Materials. Notable findings include the following: (1) the intervention group perceived racial history to be more relevant to their decision-making than the control group; (2) the relationship between the IV and the DV was mediated by the degree to which participants perceived the racial history presented to be relevant to the stated problem; (3) the intervention was correlated with a significant decrease in support for a merit-based strategy; and (4) support for a need-based strategy did not differ between Black and white participants.
Intervention Group Perceived Racial History to Be More Relevant to Their Decision-Making Than the Control Group
After completing the dependent variable, the participants were asked to self-report the relevance of seven factors to their decision to support or oppose the distributive justice strategies from 0 (completely irrelevant) to 3 (completely relevant). Racial history was the factor of most interest. The majority of participants did not believe racial history to be a relevant factor in their decision-making (Table 3). However, it should be noted that at the group level, the intervention group perceived racial history to be more relevant (M = 1.17, SD = 1.08) than the control group (M = 0.70, SD = 0.99). The difference in means was statistically significant according to a two-sided Welch’s t-test, t(1,729) = −9.4, P < 2.2 × 10−16.
Self-reported relevance of racial history | Intervention (n = 900) | Control (n = 831) | Total | |||
---|---|---|---|---|---|---|
N | % | N | % | N | % | |
Completely irrelevant | 327 | 36.3 | 505 | 60.8 | 832 | 48.1 |
Somewhat irrelevant | 226 | 25.1 | 133 | 16.0 | 359 | 20.7 |
Somewhat relevant | 215 | 23.9 | 129 | 15.5 | 344 | 19.9 |
Completely relevant | 132 | 14.7 | 64 | 7.7 | 196 | 11.3 |
Relationship between a Knowledge of Racial History and Preference for Need-Based Distributive Justice Was Mediated by the Degree to Which Participants Perceived the Racial History Presented to Be Relevant to the Stated Problem
Furthermore, we conducted a mediation analysis to further examine the role of a perceived relevance of racial history on the relationship between a knowledge of racial history and support for a need-based distributive justice strategy (Fig. 2). We added participants’ perceptions of the relevance of racial history information (i.e., the mediator) to a generalized linear model of the relationship between racial history information (−1 = control, 1 = intervention) and support for a need-based distributive justice strategy. This analysis was done using the mediation package in R (Tingley et al. 2014).

The results revealed a significant indirect effect: effect of a knowledge of racial history on the support for a need-based strategy through a relevance of racial history (β = 0.093, z = 5.614, P < 0.0001) (Table 4). That is, the history of racial history had a significant effect on the support for a need-based strategy among participants who perceived information about racial history to be relevant to climate adaptation decision-making. The total effect, the impact of a knowledge of racial history on the support for a need-based strategy without any relevance of racial history, was not significant (β = 0.087, z = 1.455, P = 0.146). This shows that the perceived relevance of racial history fully mediates the relationship between a knowledge of racial history and a support for a need-based strategy.
Effects | Estimate | 95% CI lower | 95% CI upper | P-value |
---|---|---|---|---|
ACME (indirect) | 0.09244 | 0.06104 | 0.13 | < 2 × 10−16 |
ADE (direct) | −0.00651 | −0.11640 | 0.11 | 0.91 |
Total effect | 0.08593 | −0.02593 | 0.20 | 0.13 |
Intervention Was Correlated with a Significant Decrease in Support for a Merit-Based Strategy
In the exploratory analysis, support for equality-based and merit-based distributive justice strategies were tested for differences in group means using Welch’s two-sided tests. The equality-based strategy showed no difference between the two groups, but opposition to the merit-based strategy was greater for the intervention group. The merit-based strategy proposed to allocate resources according to tax contributions from the previous year, which was operationalized as an inverse relationship to heat vulnerability. Although we did not hypothesize about this effect, it was operationalized as the exact opposite of the need-based strategy, which makes the results particularly relevant. The degree of opposition for a merit-based strategy differed significantly according to the two-sided Welch’s t-test, t(1,688) = 2.60, P = 0.009 (Table 5). The Likert scale ranged from 1 (strongly oppose) to 6 (strongly support). On average, participants in the intervention group indicated a stronger opposition (M = 2.13, SD = 1.19) than the control group (M = 2.28, SD = 1.28). The 99% confidence interval for the effect of place-based racial history on support for a need-based distributive justice strategy is between 0.001 and 0.31. These results are exploratory and were not included in a formal hypothesis. The small effect size (d = 0.125) was anticipated given the brevity of the intervention.
Distributive justice strategy | Intervention (n = 900) | Control (n = 831) | df | t | P | Cohen d | 99% CI | |||
---|---|---|---|---|---|---|---|---|---|---|
M | SD | M | SD | LL | UL | |||||
Equality | 3.48 | 1.35 | 3.46 | 1.39 | 1,709.58 | −0.31 | 0.75 | 0.015 | −0.19 | 0.15 |
Merit | 2.13 | 1.19 | 2.28 | 1.28 | 1,688.68 | 2.60 | 0.009* | 0.125 | 0.001 | 0.31 |
Support for a Need-Based Strategy Did Not Differ between Black and white Participants
Much of the literature that this work builds off examines group differences by race. Specifically, differences between Black and white participants were examined given the content of the place-based racial history. The descriptive statistics for the dependent variable, support for a need-based distributive justice strategy, are listed by race in Table 6. Table 6 shows that the mean responses for the Black control and intervention groups were (M = 4.47, SD = 1.28) and (M = 4.52, SD = 1.24), demonstrating an increase in support of 0.05. The white intervention group also showed an increase in support (M = 4.65, SD = 1.25) compared with the control group (M = 4.58, SD = 1.26), demonstrating an increase in support of 0.07. The group of multiracial Black and white participants was very small (n = 11), and therefore, they could not be considered in this analysis.
Race | Intervention | Control | ||||||||
---|---|---|---|---|---|---|---|---|---|---|
n | M | SD | Median | Mode | n | M | SD | Median | Mode | |
Black | 82 | 4.52 | 1.24 | 5 | 5 | 78 | 4.47 | 1.28 | 5 | 5 |
Black and white | 6 | 4.33 | 1.51 | 4 | 4 | 5 | 5 | 1.41 | 6 | 6 |
White | 716 | 4.65 | 1.25 | 5 | 5 | 669 | 4.58 | 1.26 | 5 | 5 |
Note: 1 = strongly oppose; and 6 = strongly support.
Discussion
This study aimed to understand how the inclusion of place-based racial history affects support for a need-based distributive justice strategy. This study indicated that the brief racial history intervention did not present a significant impact on support for a need-based distributive justice strategy. The study did uncover additional influencing factors and statistically significant exploratory findings that suggest directions for future work exploring the intersection of place-based racial history and distributive justice for climate adaptation.
While the Intervention Group Perceived Racial History as More Relevant to Decision-Making, the Majority of Participants in Both Groups Indicated That It Was Irrelevant
Despite there being a shift toward perceived greater relevance at the group level, the shift occurred between completely irrelevant and somewhat irrelevant. Therefore, this suggests that, in this case, the majority of participants did not believe that the racial legacy of the highway should be tied to decision-making in the present and about the future. This result adds complexity to and possibly represents a deviation from an assumption based off suggestions from previous studies that incorporating place-focused racial history education would increase an acknowledgment of the relevance of racial history to the built environment (Bonam et al. 2018). Specifically, for engineering decisions, which have traditionally involved less consideration of more complex social factors—like place-based racial history—there may be more of a need to elucidate exactly what that relationship is and why it matters. It may also be the case that a brief intervention providing the information for a single situation is not the only intervention that would be needed to expand this perspective. For example, systems thinking techniques may need to be explored to allow individuals to make the connections for themselves before project-level information can be useful. Future work may address this dynamic by testing the interventions of varying durations and context.
Efficacy of a Knowledge of Racial History Approach Depends on a Participant’s Perceived Relevance of That Information to the Problem They Are Being Asked to Consider
A mediation analysis revealed a strong indirect effect of perceived relevance of racial history on the relationship between a knowledge of racial history and a support for a need-based distributive justice strategy and a weak, statistically nonsignificant direct effect. These results suggest that whatever effect this intervention may have on decision-making is strongly informed by the positionality of the individual and that there is potential to see stronger effects if more participants are convinced about the relevance of the racial history being discussed to the decision being made. Because history is presented through a subjective lens and can, to an extent, be accurately presented in a variety of ways, future work should interrogate which vantage points for presenting racial history are most effective for helping a diverse population connect that history to present-day built environment decision-making. This may be a challenging prospect for engineering to grapple with because of the acknowledgment that the intervention is subjective in nature. Previous work has illustrated how engineering culture can benefit from moving away from culture aligned with depoliticization and objectivity (Cech 2013). While it is important to strive for designs that accommodate all people, these aspects of traditional engineering disregard the value that comes from a more nuanced understanding of these factors. When engineers consider the social power dynamics of their work—when they consider the multiple vantage points of a historical context—the work that they do will be politically engaged in a way that can be socially responsible. Similarly, objectivity presumes that all relevant design factors that can be quantified are removed from context, which is not the case for socially responsible projects.
Future Work: The Concept of a Merit-Based Strategy May Be More Accessible and Could Be Reframed to Function Similarly to a Need-Based Strategy
In addition to the data collected about need-based distributive justice, exploratory data on merit-based and equality-based distributive justice strategies were also collected. While there was no discernible effect on the equality-based strategy, the opposition to the merit-based strategy increased. The merit-based distributive justice strategy presented the exact opposite scenario of the need-based distributive justice strategy.
The merit-based distributive justice strategy was widely unpopular among both groups. However, the data showed a statistically significant increase in opposition between the groups for this strategy. Given the deep-rooted support of meritocratic ideology in US American identity, it makes sense that presenting a narrative of place-based critical racial history might further disrupt the perception that a strategy based on merit—without considering the context of the past—is unjust.
Given the strong response to the merit-based strategy in the study, future work may consider the different ways in which the concept of merit could be framed to prioritize communities in the same way that need-based distributive justice has the potential to. In this study, merit was presented based on a definition from the literature: “According to the principle of equity/merit, resources should be distributed in accordance with individual contributions” (Rothmund et al. 2016). Perhaps in future studies, it would be beneficial to test the framing of merit as a lack of contribution to the effects of climate change (i.e., a small carbon footprint), rather than as a contribution of financial resources. This collectivist framing of merit will differ from other contexts where merit can be more intuitively assessed at the individual level.
Future Work: Ego-Defense and Group Threat Were Not Evident in This Study, but Should Be Considered in Future Studies
The study of denial of systemic racism in the social-psychology literature has two dominant explanations about why white US Americans perceive less systemic racism than US Americans from minority groups: an information-based explanation and a motivation-based explanation (Lesick and Zell 2020). The information-based explanation was studied in this experiment. The motivation-based explanation posits that engaging with the problem of systemic racism can result in feelings of identity threat, which activates a defensive response leading to a desire to deny the existence of systemic racism or distance oneself from it (Knowles et al. 2014). In reality, both explanations are likely to play a role. If this is the case, we would expect to see a smaller increase in support for a need-based distributive justice strategy for white intervention participants than for Black intervention participants. The data did not support this idea: the difference in means between the white intervention group and the white control group was smaller (Δ = 0.07), compared with Black participants (Δ = 0.05). However, a motivation-based influence should not be entirely ruled out because the data compared previously compares two very differently sized groups: The white group comprised 1,385 participants and the Black group comprised 160 participants.
In future studies, it may be helpful to design a more complex experiment that integrates self-affirmation (Lesick and Zell 2020), perspective-taking (Mischinski 2019), or more specific guidance about how to receive and apply information about place-based racial history to the present (Onyeador et al. 2021). While these interventions have not produced replicable effects yet, they have shown promise and are still in the process of development. After stronger interventions are developed from these ideas, they will likely be helpful in developing a more complex experiment that clarifies the effects of place-based racial history for climate adaptation decision-making.
In addition to the plans for future work outlined previously, a measure of support for climate adaptation policy that has multiple items would increase the sensitivity of the measurement and require fewer participants to detect a small effect. The most closely related scale that measures attitudes toward the universal distributive justice principles is the Basic Social Justice Orientation (BSJO) scale (Hülle et al. 2018). This scale was developed and validated in German and cannot be used with an English-speaking audience without further testing. Additionally, it is framed in terms of agreeing or disagreeing with a notion of justice, whereas this study was more interested in measuring support for an operationalized representation of the justice principles.
Limitations: It Is Unlikely That Differential Attrition Had an Effect on the Study Outcomes, but It Is a Possibility
A subanalysis, available in the Supplemental Materials, was conducted to better understand the potential effects of differential attrition within the study. The analysis included examining the question behavior responsible for the differential attrition, the difference in video lengths, a quantitative analysis searching for significant differences between measured demographic variables, and a sensitivity analysis to assess the potential effect of unmeasured factors on the study outcome. The first three items in the analysis suggest that differential attrition probably did not have an impact on the study. In the event that an unmeasured variable did systematically filter out participants who would have responded in the same way to the dependent variable, the sensitivity analysis shows that their responses could have had a significant outcome on a statistically significant conclusion. However, since the hypothesis was not supported in this study, it is unlikely that differential attrition had an effect on the conclusions.
Implications for Practitioners: The Inclusion of a Place-Based Racial History in Design Can Elicit a Wide Variety of Reactions Depending on the Audience
More empirical data are needed before concrete recommendations can be presented to practitioners. In projects that are equity-focused, it is increasingly common to include place-based racial history in the background and motivation components of project discussions. The data align with a well-known practical finding: audience matters. Within the boundaries of the previously discussed limitations, this study indicated that the place-based racial history presented in this study did not present a significant impact on the overall sample, but that it had much more of an effect on participants who found the specific content of the racial history presented to be relevant. Allowing time for dialogue and conversation specifically on issues of place-based racial history may be necessary to converge on an understanding of the implications of place-based racial history on a fair allocation of resources for climate adaptation.
Conclusion
This experiment sought to examine the relationship between place-based racial history and preferences about a need-based resource allocation strategy for climate adaptation. While the brief intervention did not reveal a significant effect on preferences for a need-based strategy, the data did show a trend in that direction. The exploratory analysis found that the relationship between the independent and the dependent variable was mediated by a perceived relevance of the racial history presented and that the intervention group more strongly opposed the merit-based strategy, suggesting that the intervention helped counter decontextualized attribution of merit. These findings indicate promising directions for future research on the utility of need-based and contextualized merit-based adaptation strategies in creating a navigable design landscape for frontline designers.
Supplemental Materials
File (supplemental materials_aomjah.aoeng-0002_gordon.pdf)
- Download
- 1.89 MB
Data Availability Statement
Some or all data, models, or code that support the findings of this study are available from the corresponding author upon reasonable request.
Acknowledgments
The authors would like to thank Sophie Trawalter, Benjamin Converse, Arsalan Heydarian, and Roslyn Berne for their feedback on this work. Additionally, this material is based on work supported by (1) the United States National Science Foundation Graduate Research Fellowship Program under Grant No. 1842490; and (2) the United States National Science Foundation under Grant No. 153104. Any opinions, findings, and conclusions or recommendations expressed in this material are those of the author(s) and do not necessarily reflect the views of the National Science Foundation.
References
Aguinis, H., I. Villamor, and R. S. Ramani. 2021. “MTurk research: Review and recommendations.” J. Manage. 47 (4): 823–837. https://doi.org/10.1177/0149206320969787.
Axt, J. R. 2018. “The best way to measure explicit racial attitudes is to ask about them.” Social Psychol. Personality Sci. 9 (8): 896–906. https://doi.org/10.1177/1948550617728995.
Ayers, J., and T. Forsyth. 2009. “Community-based adaptation to climate change.” Environ. Sci. Policy Sustainable Dev. 51 (4): 22–31. https://doi.org/10.3200/ENV.51.4.22-31.
Bonam, C. M., V. N. Das, B. R. Coleman, and P. Salter. 2018. “Denying history, denying racism: Replicating and extending the Marley hypothesis.” Social Psychol. Personality Sci. 10: 257–265. https://doi.org/10.1177/1948550617751583.
Bonam, C. M., V. N. Das, B. R. Coleman, and P. Salter. 2019. “Ignoring history, denying racism: Mounting evidence for the Marley hypothesis and epistemologies of ignorance.” Social Psychol. Personality Sci. 10 (2): 257–265. https://doi.org/10.1177/1948550617751583.
Bozeman, J. F. III, E. Nobler, and D. Nock. 2022. “A path toward systemic equity in life cycle assessment and decision-making: Standardizing sociodemographic data practices.” Environ. Eng. Sci. 39 (9): 759–769. https://doi.org/10.1089/ees.2021.0375.
Cech, E. A. 2013. “The (Mis) framing of social justice: Why ideologies of depoliticization and meritocracy hinder engineers’ ability to think about social injustices.” In Engineering education for social justice: Critical explorations and opportunities, philosophy of engineering and technology, edited by J. Lucena, 67–84. Dordrecht, The Netherlands: Springer.
Center on Society and Health. 2016. “Mapping life expectancy.” Virginia Commonwealth University Center on Society and Health. Accessed September 26, 2016. https://societyhealth.vcu.edu/work/the-projects/mapping-life-expectancy.html.
City of Richmond. 2022. “What is RVAgreen 2050? | Richmond.” Accessed February 10, 2022. https://www.rva.gov/sustainability/what-rvagreen-2050-0.
City of Richmond, Office of Sustainability. 2021. “RVAgreen 2050 climate equity index.” City of Richmond Office of Sustainability. Accessed February 10, 2022. https://www.arcgis.com/apps/webappviewer/index.html?id=e4d732f225fe457d83df11fe9bf71daf.
Clayton, S., E. Kals, and I. Feygina. 2016. “Justice and environmental sustainability.” In Handbook of social justice theory and research, edited C. Sabbagh and M. Schmitt, 369–386. New York: Springer.
Coleman, B. R., C. M. Bonam, and C. Yantis. 2019. “‘I thought ghettos just happened’: White Americans’ responses to learning about place-based critical history.” In History and collective memory from the margins: A global perspective, cultural studies in the third millennium, edited by S. Mukherjee and P. S. Salter, 207–228. Hauppauge, NY: Nova Science Publishers, Incorporated.
Cook, K. S., and K. A. Hegtvedt. 1983. “Distributive justice, equity, and equality.” Annu. Rev. Sociol. 9 (1): 217–241. https://doi.org/10.1146/annurev.so.09.080183.001245.
Costanza-Chock, S. 2020. Design justice: Community-led practices to build the worlds we need. Cambridge, MA: The MIT Press.
Delacre, M., D. Lakens, and C. Leys. 2017. “Why psychologists should by default use Welch’s t-test instead of Student’s t-test.” Int. Rev. Social Psychol. 30 (1): 92–101. https://doi.org/10.5334/irsp.82.
DeFazio, P. A. 2021. “H.R.3684—117th Congress (2021–2022): Infrastructure investment and jobs act.” Rep. [D-OR-4]. Legislation. 2021-06-04. Accessed November 15, 2021. http://www.congress.gov/bill/117th-congress/house-bill/3684.
Deutsch, M. 1975. “Equity, equality, and need: What determines which value will be used as the basis of distributive justice?” J. Soc. Issues. 31 (3): 137–149. https://doi.org/10.1111/j.1540-4560.1975.tb01000.x.
Dodman, D., and D. Mitlin. 2013. “Challenges for community-based adaptation: Discovering the potential for transformation.” J. Int. Dev. 25 (5): 640–659. https://doi.org/10.1002/jid.1772.
Evans, M. T. 2015. “Chapter 6: The intersection of I-95 and Black Wall Street.” Main Street, America: Histories of I-95, 192–213. Accessed October 20, 2021. https://scholarcommons.sc.edu/cgi/viewcontent.cgi?article=4228&context=etd.
Faul, F., E. Erdfelder, A. Buchner, and A.-G. Lang. 2009. “Statistical power analyses using G*power 3.1: Tests for correlation and regression analyses.” Behav. Res. Methods 41 (4): 1149–1160. https://doi.org/10.3758/BRM.41.4.1149.
Gabriel, C.-A., and C. Bond. 2019. “Need, entitlement and desert: A distributive justice framework for consumption degrowth.” Ecol. Econ. 156: 327–336. https://doi.org/10.1016/j.ecolecon.2018.10.006.
Gollwitzer, M., and J.-W. van Prooijen. 2016. “Psychology of justice.” In Handbook of social justice theory and research, edited by C. Sabbagh and M. Schmitt, 61–82. New York: Springer.
Gordon, B., and L. Klotz. 2021. “Preregistration: place-focused racial history and climate adapation decision-making.” Center for open science. OSF. Accessed December 20, 2021. https://osf.io/jt5rm.
Hoffman, J. S., V. Shandas, and N. Pendleton. 2020. “The effects of historical housing policies on resident exposure to intra-urban heat: A study of 108 US urban areas.” Climate 8 (1): 12. https://doi.org/10.3390/cli8010012.
Hülle, S., S. Liebig, and M. J. May. 2018. “Measuring attitudes toward distributive justice: The basic social justice orientations scale.” Social Indic. Res. 136 (2): 663–692. https://doi.org/10.1007/s11205-017-1580-x.
IPCC. 2022. “Climate Change 2022: Mitigation of Climate Change.” In Contribution of working group III to the sixth assessment report of the intergovernmental panel on climate change, edited by P. R. Shukla, J. Skea, R. Slade, A. A. Khourdajie, R. van Diemen, D. McCollum, M. Pathak, S. Some, P. Vyas, R. Fradera, M. Belkacemi, A. Hasija, G. Lisboa, S. Luz, and J. Malley. Cambridge, UK: Cambridge University Press.
Kassambara, A. 2020. “ggpubr:‘ ggplot2’ based publication ready plots.” Accessed March 6, 2022. https://CRAN.R-project.org/package=ggpubr.
Kassambara, A. 2021. “rstatix: Pipe-friendly framework for basic statistical tests.” Accessed March 6, 2022. https://CRAN.R-project.org/package=rstatix.
Kirkby, P., C. Williams, and S. Huq. 2018. “Community-based adaptation (CBA): Adding conceptual clarity to the approach, and establishing its principles and challenges.” Clim. Dev. 10 (7): 577–589. https://doi.org/10.1080/17565529.2017.1372265.
Knowles, E. D., B. S. Lowery, R. M. Chow, and M. M. Unzueta. 2014. “Deny, distance, or dismantle? How White Americans manage a privileged identity.” Perspect. Psychol. Sci. 9 (6): 594–609. https://doi.org/10.1177/1745691614554658.
Konow, J. 2001. “Fair and square: The four sides of distributive justice.” J. Econ. Behav. Organ. 46 (2): 137–164. https://doi.org/10.1016/S0167-2681(01)00194-9.
Kronlid, D. 2014. “Chapter 7: Adaptation for well-being.” In Climate change adaptation and human capabilities: Justice and ethics in research and policy, edited by D. O. Kronlid, 157–180. New York: Palgrave Macmillan US.
Lesick, T. L., and E. Zell. 2020. “Is affirmation the cure? Self-affirmation and European-Americans’ perception of systemic racism.” In Basic and applied social psychology, 1–13. London: Routledge.
Lipsitz, G. 2011. How racism takes place. Philadelphia, PA: Temple University Press.
Litman, L., J. Robinson, and T. Abberbock. 2017. “Turkprime.Com: A versatile crowdsourcing data acquisition platform for the behavioral sciences.” Behav. Res. 49 (2): 433–442. https://doi.org/10.3758/s13428-016-0727-z.
Marquette, M. M. 2020. “Centering social equity in the climate action planning process: Lessons for Richmond, Virginia.” Master of urban and regional planning. Richmond, VA: Virginia Commonwealth University.
Matin, N., J. Forrester, and J. Ensor. 2018. “What is equitable resilience?” World Dev. 109: 197–205. https://doi.org/10.1016/j.worlddev.2018.04.020.
Meerow, S., and J. P. Newell. 2019. “Urban resilience for whom, what, when, where, and why?” Urban Geogr. 40 (3): 309–329. https://doi.org/10.1080/02723638.2016.1206395.
Mischinski, M. M. 2019. Historical knowledge and social identity: Appeasing identity threat among high-identity majority group members through perspective-taking. Ph.D. thesis, Graduate School of Arts and Sciences, Wake Forest Univ.
Nelson, J. C., G. Adams, and P. S. Salter. 2013. “The Marley hypothesis: Denial of racism reflects ignorance of history.” Psychol. Sci. 24 (2): 213–218. https://doi.org/10.1177/0956797612451466.
Norton, M. I., and S. R. Sommers. 2011. “Whites see Racism as a zero-sum game that they are now losing.” Perspect. Psychol. Sci. 6 (3): 215–218. https://doi.org/10.1177/1745691611406922.
Nussbaum, M. 1988. “Nature, function and capability: Aristotle on political distribution.” Oxford Studies in Ancient Philosophy, edited by J. Annas and R. H. Grimm, 145–184. Oxford, New York: Oxford University Press.
Onyeador, I. N., N. M. Daumeyer, J. M. Rucker, A. Duker, M. W. Kraus, and J. A. Richeson. 2021. “Disrupting beliefs in racial progress: Reminders of persistent racism alter perceptions of past, but not current, racial economic equality.” Personality Social Psychol. Bull. 47 (5): 753–765. https://doi.org/10.1177/0146167220942625.
Pereira, R. H. M., T. Schwanen, and D. Banister. 2017. “Distributive justice and equity in transportation.” Transport Rev. 37 (2): 170–191. https://doi.org/10.1080/01441647.2016.1257660.
Rasch, D., K. D. Kubinger, and K. Moder. 2011. “The two-sample t test: Pre-testing its assumptions does not pay off.” Stat. Pap. 52 (1): 219–231. https://doi.org/10.1007/s00362-009-0224-x.
Rawls, J. 1999. A theory of justice: Revised edition. Cambridge, MA: Harvard University Press.
Robinson, D., A. Hayes, and S. Couch. 2021. “Broom: Convert statistical objects into tidy tibbles.” Accessed March 6, 2022. https://CRAN.R-project.org/package=broom.
Rochon, J., M. Gondan, and M. Kieser. 2012. “To test or not to test: Preliminary assessment of normality when comparing two independent samples.” BMC Med. Res. Methodol. 12 (1): 1–11. https://doi.org/10.1186/1471-2288-12-81.
Rothmund, T., J. C. Becker, and J. T. Jost. 2016. “The psychology of social justice in political thought and action.” In Handbook of social justice theory and research, edited by C. Sabbagh and M. Schmitt, 275–291. New York: Springer.
Rothstein, R. 2017. The color of law: A forgotten history of how our government segregated America. New York: Liveright.
Saverino, K. C., E. Routman, T. R. Lookingbill, A. M. Eanes, J. S. Hoffman, and R. Bao. 2021. “Thermal inequity in Richmond, VA: The effect of an unjust evolution of the urban landscape on urban heat islands.” Sustainability 13 (3): 1511. https://doi.org/10.3390/su13031511.
Schlosberg, D. 2012. “Climate justice and capabilities: A framework for adaptation policy.” Ethics Int. Aff. 26 (4): 445–461. https://doi.org/10.1017/S0892679412000615.
Sen, A. K. 2009. The idea of justice. Cambridge, MA: Belknap Press.
Siders, A. R., I. Ajibade, and D. Casagrande. 2021. “Transformative potential of managed retreat as climate adaptation.” Curr. Opin. Environ. Sustainability 50: 272–280. https://doi.org/10.1016/j.cosust.2021.06.007.
Simon, H. A. 1988. “The science of design: Creating the artificial.” Des. Issues 4 (1/2): 67–82. https://doi.org/10.2307/1511391.
Strickhouser, J. E., E. Zell, and K. E. Harris. 2019. “Ignorance of history and perceptions of racism: Another look at the Marley hypothesis.” Social Psychol. Personality Sci. 10 (7): 977–985. https://doi.org/10.1177/1948550618808863.
Tingley, D., T. Yamamoto, K. Hirose, L. Keele, and K. Imai. 2014. “Mediation: R package for causal mediation analysis.” UCLA statistics/American Statistical Association. Accessed October 25, 2022. http://hdl.handle.net/1721.1/91154.
Traub, S., and B. Kittel. 2020. Need-based distributive justice: An interdisciplinary perspective. Cham, Switzerland: Springer Nature.
Vedachalam, S., D. L. Kay, and S. J. Riha. 2014. “Capital investment and privatization: Public opinion on issues related to water and wastewater infrastructure.” Public Works Manage. Policy 19 (2): 118–147. https://doi.org/10.1177/1087724X13500240.
Warshaw, C. 2019. “Local elections and representation in the United States.” Ann. Rev. Political Sci. 22 (1): 461–479. https://doi.org/10.1146/annurev-polisci-050317-071108.
Wickham, H. 2016. ggplot2: Elegant graphics for data analysis. New York: Springer.
Wickham, H. 2021. “tidyr: Tidy Messy data.” Accessed March 6, 2022. https://CRAN.R-project.org/package=tidyr.
Wickham, H., R. François, L. Henry, and K. Müller. 2021. “dplyr: A grammar of data manipulation.” Accessed March 6, 2022. https://CRAN.R-project.org/package=dplyr.
Yarmuth, J. A. 2022. “H.R.5376—117th congress (2021–2022): Inflation reduction act of 2022.” Rep. [D-KY-3]. Legislation. 2021-09-27. Accessed August 16, 2022. http://www.congress.gov/bill/117th-congress/house-bill/5376.
Information & Authors
Information
Published In
Copyright
This work is made available under the terms of the Creative Commons Attribution 4.0 International license, https://creativecommons.org/licenses/by/4.0/.
History
Received: Jan 22, 2023
Accepted: Sep 19, 2023
Published online: Oct 17, 2023
Published in print: Dec 31, 2023
Discussion open until: Mar 17, 2024
Authors
Metrics & Citations
Metrics
Citations
Download citation
If you have the appropriate software installed, you can download article citation data to the citation manager of your choice. Simply select your manager software from the list below and click Download.