The Things That Hurt People Are Not the Same as the Things That Kill People: Key Differences in the Proximal Causes of Low- and High-Severity Construction Injuries
Publication: Journal of Construction Engineering and Management
Volume 150, Issue 8
Abstract
The safety profession has been shaped by the assumption that there is a fixed ratio of low- to high-severity injuries and the notion that injuries of all severity levels share the same general causes. There is now very strong empirical evidence that this ratio is not fixed, which has led to a new hypothesis that serious injuries and fatalities (SIFs) happen for different reasons than lower-severity injuries and the idea that a targeted approach is needed for SIF prevention. To better understand this phenomenon, this study explored the question: what, if anything, is different about the causes of SIFs? To answer this question, a research team of construction safety experts and academics hypothesized seven factors that may be unique to high-severity injuries. Concurrently, details of serious injuries and fatalities (SIF, ), potential serious injuries and fatalities (PSIF, ), and low-severity injuries (LSI, ) were collected from utility, oil and gas, commercial, pipeline, and specialized construction trades. Blind to each injury outcome, the team assessed the presence or absence of the seven potential differentiators. The samples were then compared using Fisher’s exact test to determine if any factors explained the differences among the injury types. Although there were no differences between SIF and PSIF cases, two factors differentiated LSI and PSIF/SIF: (1) absent direct controls; and (2) absent or not followed work plan. Surprisingly, no human factors were significant. The results indicate that the control of high-energy hazards through effective work planning, discipline, and execution is vital for targeting SIFs, supporting the theory that a differentiated approach is needed to avoid the concerning plateau in the rate of construction fatal injuries.
Practical Applications
The authors present a study that elucidates two factors highly prevalent in high-severity injuries that are absent in low-severity injuries: (1) absent and not followed work plan; and (2) absent direct controls. This finding supports the theory that a differentiated approach is needed to prevent serious injuries and fatalities (SIFs) in construction, and the key is the control of high-energy hazards (i.e., hazards involving 1,500 J or more) through proper planning, discipline, and execution. Additionally, the authors provide empirical evidence that a targeted SIF approach can be leveraged through the monitoring and learning from near misses with the potential for SIFs, as they share the same contributing factors as SIFs. Given that incidents resulting in SIFs are so infrequent to permit any meaningful learnings from a statistical standpoint, equivalency in learnings from near misses with potential for SIF vastly expands proactive decision-making opportunities for organizations. Interestingly, there were no differences in the human factors that precede injuries of varying severity, implying that safety practices focused on human behavior might be important for injury prevention broadly, but not for targeting the concerning plateau on SIFs directly.
Motivation
For nearly a century, the safety profession has been influenced by the “safety pyramid.” The theory introduced by Heinrich (1931) and later refined by Bird (1966) suggested that there is a constant ratio of minor to major injuries. When building the safety pyramid, Heinrich used insurance data to establish a ratio among accidents of varying severity levels. Specifically, he found that for every 300 near hits (incidents resulting in no injury) there are 29 minor injuries and one major injury (Heinrich 1941). Bird (1966) later expanded this theory to include unsafe acts and used the safety pyramid to support the thesis that minor and major accidents have similar causes, and that injury severity is fortuitous.
Although Heinrich did not suggest that the safety pyramid was causal, his work was cited to support the pervasive belief that preventing low-severity injuries leads to a corresponding reduction in high-severity injuries (Manuele 2002). This fundamental concept served as the foundation of many accident prevention programs (Khanzode et al. 2012; Marshall et al. 2018; Rebbitt 2014), which led to a generalized approach to safety management. Consequently, most traditional safety programs are structured to prevent all types of injuries without specific focus on more severe injuries.
There is an ongoing debate about the validity of the ratios in the safety pyramid. Some researchers found a correlation in the rates of minor and major construction injuries over long periods (e.g., Hubbard and Neil 1986) and a similar distribution of high-risk tasks preceding minor and major injuries (Lozada-Larsen and Laughery 1987; Laughery and Vaubel 1993). However, others disputed these studies, claiming that they failed to account for the confounding factors that influence injury severity such as the interaction with energy, age of the injured employee, nature of injury, and injured body part (e.g., Petersen 1989; Salminen et al. 1992; Manuele 2011; Cooper 2019; Busch et al. 2021). Furthermore, although ratios appeared to be stable, some studies (e.g., Zhou et al. 2012) have found differences in the frequency of causal factors that lead to near misses, less severe accidents, and serious accidents in construction. Thus, the correlations fail to pass as causational evidence (Rebbitt 2014; Dunlap et al. 2019). Although these studies have elucidated the limitations in the validity of the pyramid ratios, the extent to which these limitations justify the need for a differentiated approach to safety management in construction is still uncertain.
If the ratio of low- to high-severity injuries was indeed fixed, then long-term injury rates must trend in parallel. However, macrolevel trends have revealed that, while the rate of lower-severity injuries has declined over the last decade, the rate of fatalities has plateaued in the USA (BLS 2022). This divergent trend is similar when comparing the number of fatalities to the number of nonfatal injuries. To illustrate this trend, the authors created Fig. 1 using fatal and nonfatal injury data from 2010 to 2021 collected by the Occupational Safety and Health Administration (OSHA). The three-year rolling averages in Fig. 1 show that the rate of nonfatal construction injuries has declined dramatically while the fatality rate has plateaued. If the ratio of major to minor injuries were indeed fixed, this divergent trend would be impossible, especially at an industry level representing billions of worker hours. This counterintuitive trend supports the emerging viewpoint that focused injury prevention efforts are needed to prevent serious injuries and fatalities (e.g., Manuele 2008, 2011; Shanon and Manning 1980) and raised the following question: what if anything, is different about the causes of serious injuries and fatalities (SIFs)? Answering this question will reveal key aspects of a differentiated approach to prevent SIF in construction. This is aimed at addressing the disconcerting lack of improvement in the rate of fatal injuries shown in Fig. 1.

Literature Review
To establish the specific gap in knowledge, research related to the causes of high- and low-severity injuries and the proximal causes of SIF was reviewed. For consistency and brevity, the term serious injury or fatality (SIF) is used to refer to higher-severity injuries (i.e., life-threatening, life-altering, and life-ending injuries as defined by Bayona et al. (2023), while low-severity injury (LSI) is used to broadly refer to injuries that are less than serious (i.e., actual injuries that are not life-threatening, life-altering, and life-ending).
Relationship between the Causes of SIFs and LSIs
Researchers have begun to find different contributing factors for higher-severity injuries (Bellamy 2015; Dunlap et al. 2019; Hubbard and Neil 1986; Lozada-Larsen and Laughery 1987). Some differences are related to the work itself such as equipment, tools, and transportation activities (Nussey 1980; Groves et al. 2007), while others show differences in the body parts injured (Salminen et al. 1992). For example, in the mining sector, Nussey (1980) found that haulage and transportation accidents are more linked to lost-time injuries compared to less severe injuries and Groves et al. (2007) found that off-road and underground ore haulage is more linked to fatal injuries than nonfatal injuries. Interestingly, these historical studies were conducted at the industry level and focused on general features of work performed (e.g., transportation).
Several researchers studied more proximal factors contributing to injuries of different severity levels. For example, Salminen et al. (1992) examined 99 SIF cases in Finland and found that working without supervision was more often related to fatalities than less serious injuries; Alizadeh et al. (2015) found that younger workers are more vulnerable to SIFs than older workers and that stronger prior injury history is linked to lower likelihood of severe injuries; Bellamy (2015) found differences in the proportions of common underlying causes of fatal and serious nonfatal accidents (e.g., ventilation failure was present in 36% of fatal accidents but only in 13% of nonfatal accidents resulting from explosions); and Love et al. (2019) found that mobile equipment transport is associated with more severe injuries.
Although most studies suggest that there are differences in the causal factors of injuries of varying severity, Bellamy (2015) found similar causes between nonfatal and fatal accidents when controlling for the same type of hazard. In their study, they examined 36 Dutch safety barrier failures that led to serious accidents and found that all identified causes of fatal accidents were also present in nonfatal injuries (Bellamy 2015). This conflicting evidence has led to debate among researchers and professionals regarding whether targeted interventions are needed to manage SIFs.
Despite the ongoing debates, there is a dearth of research into the differences in causes of SIFs that are proximal and actionable. Understanding SIFs are more common for younger workers or studying incidents that result in head, trunk, or lower limb injuries does not provide actionable insight into how work can be managed differently to prevent all SIFs. That is, previous research has not equally considered active and latent human factors, management, and organizational failures that contribute to injuries (Reason 1990), nor has it incorporated variables present in dynamic environments where the sources of hazards are ill-defined (e.g., Rasmussen 1997). Although researchers have attempted to study these factors, they reported legal constraints that have limited how much empirical information could be gathered, often relying only on publicly available information (e.g., Haslam et al. 2005). Nevertheless, tailoring an approach to target high-severity injuries requires knowledge about the actionable differences such as human factors, management activities, or hazard types.
Energy Assessment to Differentiate SIF-Related Hazards
Energy assessment was recently introduced as an avenue of exploring differences in low- and high-severity injuries that relate to the fundamental nature of the hazards involved. Specifically, Hallowell et al. (2017) found a direct connection between the magnitude of physical energy associated with a hazard and the severity of injury. That is, more energy causes more harm, and at a certain level (approximately 1,500 J), a SIF became the most likely outcome. This knowledge confirmed that there are differences in the hazards that cause more serious injuries. However, the study did not elucidate specific precursors or warning signs related to human factors or work context (i.e., why the energy was released and contacted a worker such as human factors, management activities, or controls). Such information is needed to better understand how to manage high-energy work.
Proximal Causes of SIFs in Construction
Although there is relatively limited research that has explored the differences between SIFs and LSIs, there is a wealth of knowledge regarding the immediate factors that contribute to SIFs. For example, precursor analysis has been used to identify anomalous conditions, events, and sequences that precede a catastrophic incident (NAE 2004). Precursor analysis began in nuclear (Ballard 1990), aerospace (Groen et al. 2011), and healthcare (Mitchell et al. 2016) domains. Recently, precursor analysis was adapted to the construction industry by Alexander et al. (2017a, p. 1) who defined a precursor as a “detectable event, condition, or action that serves as a warning sign to a fatal or disabling injury.” Precursors explicitly distinguish conditions with a high probability of a SIF from situations where no incident occurs (i.e., safe work). That is, precursors distinguish safe from unsafe work, but they do not necessarily distinguish SIFs from LSIs.
Some examples of precursors in construction include but are not limited to significant overtime, improvisation, deficient safety enforcement by foremen (Alexander et al. 2017a; Toole 2002), limited supervision, lack of controls, poor pretask planning (Alexander et al. 2017b; Winge et al. 2019), unavailability of construction materials (Zou and Zhang 2009), and unstable ground conditions (Pereira et al. 2020; Suraji et al. 2001). In a controlled experiment, Alexander et al. (2017a, b) found 16 potential precursors that were highly predictive of SIF. Since then, researchers followed a similar approach to identify precursors that are unique to utility construction such as risk normalization, safety attitudes, and perceived safety culture (see Table 1; EEI 2019). Although the precursor research does not specifically differentiate SIF from LSI, they were a starting point for the hypotheses in this study.
Precursor list | From Alexander et al. (2017a, b) | From EEI’s customization study (EEI 2019) |
---|---|---|
Crew is unaware of standard work procedure | X | X |
No/poor plan to address work changes | X | X |
No/poor pretask plan/discussion specific to work | X | X |
Schedule/productivity pressure | X | X |
Lack of control barrier and/or visual warning | X | — |
Line of fire is uncontrolled | X | — |
Congested workspace/crowding | X | — |
Crew members are not active in safety | X | — |
Distracted workers | X | — |
Fatigue | X | — |
Improvisation | X | — |
Limited safety supervision | X | — |
Poor quality or inexperienced foreman | X | — |
Prior safety performance is poor | X | — |
Significant overtime | X | — |
Working alone | X | — |
Departure from routine | — | X |
Familiarity with task | — | X |
Hazard recognition | — | X |
Perceived safety culture | — | X |
Risk normalization | — | X |
Rules and procedures | — | X |
Safety attitudes | — | X |
Stop work execution | — | X |
Workers inactive in safety | — | X |
Each of the previous precursor studies focused on identifying the difference between injury and no-injury cases (i.e., a SIF versus no injury). However, to date, there is no known experiment to study the difference between SIFs and LSIs in construction. Furthermore, it is unclear whether there are any differences between the precursors that precede near misses with the potential for SIFs and the precursors that lead to actual SIFs.
Proximal Causes of Potential SIFs
SIFs are paradoxical. Although it is fortunate that SIF events are rare, the low rate of occurrence hampers our ability to trend and learn (Hallowell et al. 2021). Thus, practitioners and researchers have begun to study incidents that had the potential to result in a SIF (e.g., Cooper 2019; Hallowell 2020). The underlying assumption is that incidents with the potential for a SIF (i.e., PSIF) share the same underlying warning signs as actual SIF events, and the only difference is the outcome. For instance, some authors (e.g., Martin and Black 2015) have relied on this assumption to explore the differences in the causes of SIF events and incidents that had no SIF exposure in various industrial sectors such as oil and natural gas extraction. By extension, it is hypothesized that identifying and monitoring the precursors of PSIF can help organizations learn how to prevent SIF. Unfortunately, just as with the assumptions based on the safety pyramid, the assumption that SIF and PSIF incidents share the same precursors has not yet been empirically validated in the construction sector. This study tested this assumption directly.
Research Objective
Divergent macrolevel trends in high- and low-severity incidents over the last decade suggest that the proximal causes of SIFs and lower-severity injuries are possibly different. Inferential evidence supports this hypothesis and suggests that the differentiating factors could be linked to specific types of equipment and tools used (Nussey 1980; Groves et al. 2007; Love et al. 2019), human–object/machine interaction (Shanon and Manning 1980; Laughery and Vaubel 1993; Salminen et al. 1992; Love et al. 2019), supervision factors (Salminen et al. 1992; Haslam et al. 2005; McSween and Moran 2017; Winge et al. 2019), or even demographic factors and body parts (Alizadeh et al. 2015; Salminen et al. 1992; Love et al. 2019). Thus, there is a growing consensus among researchers that SIFs must be managed differently (Busch et al. 2021; Krause and Murray 2012; Manuele 2008). However, despite this mounting evidence, there is some counterevidence provided by Bellamy (2015, p. 98) who found that, “fatal and (serious) nonfatal accidents share the same causes but not in the same proportions.”
This study aimed to contribute to this debate by undertaking a comparative analysis of SIF, PSIF, and LSI cases and determine whether there are statistically significant differences in the causal factors. This study builds upon but deviates from existing knowledge by examining the proximal causes using actual incidents, a blind assessment by experts, and statistical testing. Specifically, the study was designed to answer the following questions:
1.
What are the differences, if any, in the proximal causes of serious injuries and fatalities (SIFs) and low-severity injuries (LSIs)?
2.
What are the differences, if any, in the proximal causes of serious injuries and fatalities (SIFs) and potential serious injuries and fatalities (PSIFs)?
Answering these questions would provide a deeper understanding of how SIFs can be specifically addressed in modern safety programs. Including PSIFs in the study will elucidate the extent to which near misses can be used for SIF-related learning.
Research Methods
Since the main objective was to identify any differences in the proximal causes of SIFs, PSIFs, and LSIs (i.e., “SIF differentiators”), operational definitions of each group were created. The definitions of these key incident types are as follows:
•
Serious injury or fatality (SIF): a physical injury that results in a life-ending, life-threatening, or life-altering injury (Bayona et al. 2023).
•
Potential serious injury or fatality (PSIF): an incident involving exposure to greater than 1,500 J of energy in the absence of a direct control where a SIF was not sustained (Hallowell 2020). As defined in Hallowell (2020) a direct control is a safeguard that is targeted to the energy source; effectively mitigates the energy when installed, verified, and used properly; and is effective even if someone makes an unintentional human error during work.
•
Low-severity injury (LSI): a physical injury requiring first-aid treatment or less and involves less than 500 J of energy [threshold discussed by Hallowell et al. (2017); e.g., a minor bruise resulting from fall to the same level].
Research Process
The research process involved six steps: (1) convening a panel of safety experts; (2) brainstorming and prioritizing a small set of hypothesized SIF differentiators; (3) collecting actual incident cases that resulted in outcomes of varying severity; (4) using a standardized retrospective data collection tool to determine the presence of the hypothesized SIF differentiators in the incidents collected; (5) conducting a focus group to examine the information from the field to determine whether the hypothesized SIF differentiators were present; and (6) testing the statistical significance of any relationship between the severity outcome from the cases and the frequency of the hypothesized SIF differentiators determined by the experts to be present. Fig. 2 outlines the overall research process. The expert panelists and the cases obtained were from the North American construction industry.
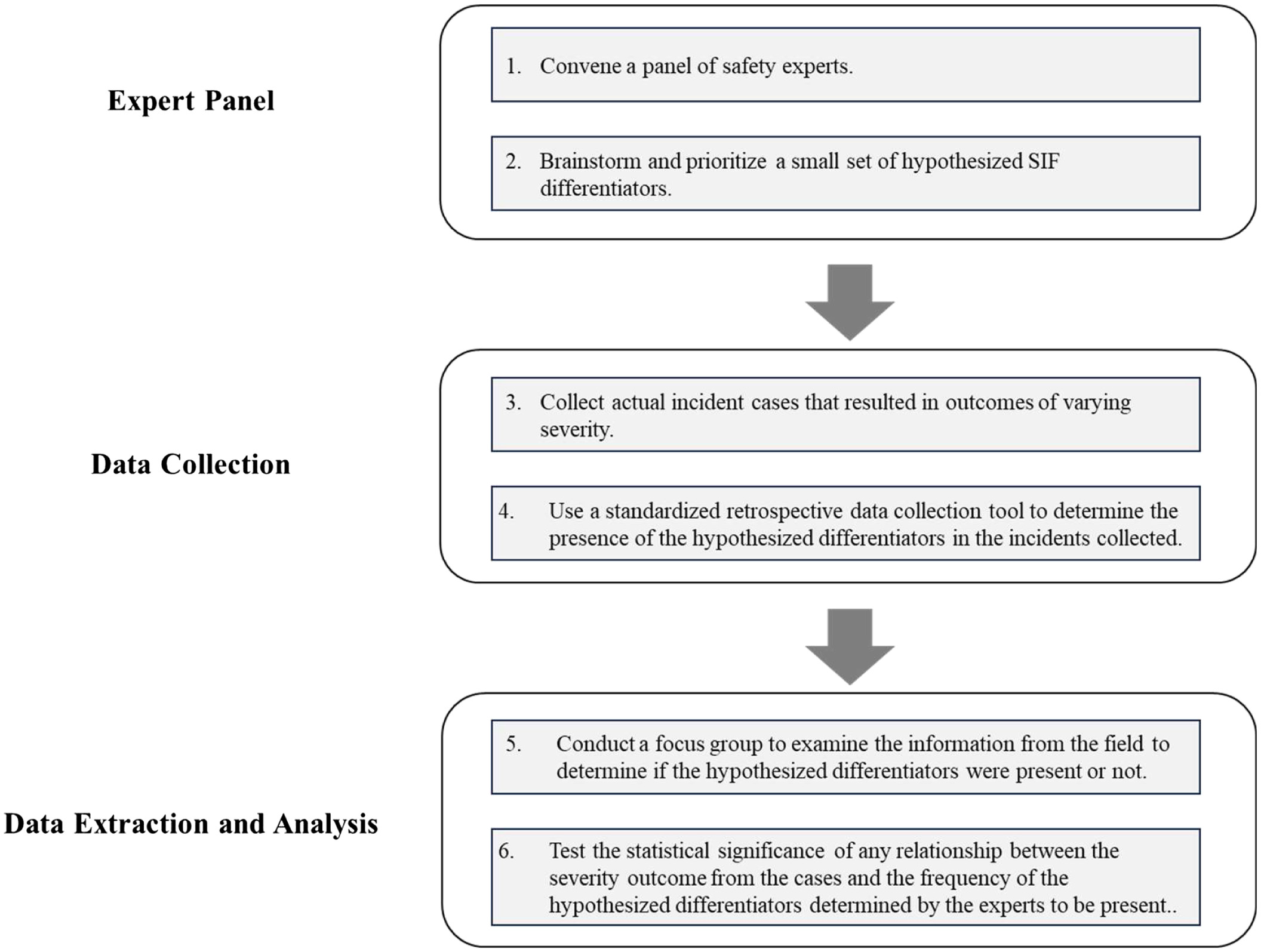
Creating a Focus Group of Construction Safety Experts
A panel of safety experts was assembled to participate in the study by hypothesizing potential differentiators, collecting actual injury cases for review, participating in a focus group to assess the presence of the differentiators in each case, and interpreting the results. Seventeen safety experts from across North America were recruited from the membership in the Construction Safety Research Alliance (CSRA). Their participation was voluntary and uncompensated. The participants represented different sectors of the industry such as utilities (34%); commercial, infrastructure, and industrial construction (24%); oil and gas (24%); and specialty trades (18%). The group had an average of 16 years of professional experience in the safety field (collective total of 270 years). All met the following expertise requirements suggested by Hallowell and Gambatese (2010): (1) five or more years of professional experience in the construction industry; (2) a bachelor’s degree in construction engineering and management or related fields; and (3) certification as a safety professional.
The expert panel deliberations were best characterized as a focus group based on open discussions and the controls implemented to mitigate bias (see Table 2). Here, 17 safety experts served as the participants and three academic researchers served as the facilitators. Because a larger group is recommended for developing hypotheses (Cortini et al. 2019; Dawson et al. 1993), the full panel convened to brainstorm potential SIF differentiators. Once the list of potential factors was narrowed down, a subset of the full panel convened to prioritize the hypothesized factors through richer and more in-depth discussions per the recommendations of Cortini et al. (2019). These smaller subcommittees of the expert panel included five to seven experts each.
Threats to validity | Experimental controls | Impact on validity | Citations |
---|---|---|---|
Individual’s preexisting beliefs (i.e., confirmation and memory bias) | Selection of expert panel representing different industry sectors | Objective selection of variables to test hypotheses (i.e., shortlist) | Bleijenbergh et al. (2011) and Bouma and Ling (2004) |
Groupthink (i.e., bandwagon bias) | Iterative rounds of discussion | Improved decision-making process and accountability of ideas | Bhandari and Hallowell (2021) and Morewedge et al. (2015) |
Bias in the materials used (i.e., primacy effect) and moderator bias | Open-ended questions and structured prompts that sparked discussion. Consistent moderator. | Decisions based on panel’s viewpoints, not researchers’ intuition. | Nahari and Ben-Shakhar (2013), Tang (2020), and Smithson (2000) |
During each case review, one academic and the expert who provided the case served as the discussion leaders to encourage an organized and informed deliberation as suggested by O’ Nyumba et al. (2018). Several steps were taken to enhance the internal validity of the focus group discussions such as iterative rounds of discussion and open-ended questions used during these discussions. These steps helped to proactively address threats to validity linked to preexisting beliefs, groupthink, and bias in the materials used. These experimental controls and their impact on validity are summarized in Table 2.
Prioritizing Potential SIF Differentiators
Once the expert panel was established, they were asked to identify a small subset of factors with the highest potential to be proximal and actionable differentiators of SIF. The panel relied on a few additional controls to prevent domineering participants from largely influencing the focus group. First, the panel was composed of seasoned professionals with similar occupational positions. This relative homogeneity in experience of experts and the use of a consistent set of predefined questions may assist in minimizing bandwagon effects (Leung et al. 2014; Smithson 2000; Bhandari and Hallowell 2021). Furthermore, the facilitator of the focus groups ensured active participation by directly asking all participants for their answers one at a time (round-robin format; Smithson 2000). However, as with any group-based opinion study, elimination of these confounding effects cannot be guaranteed, which is a limitation.
The team elected to study a small set of hypothesized differentiators because of time and resource constraints and the desire to study a few potential differentiators thoroughly rather than superficially examining a long list. To seed these discussions, the expert panel reviewed the 29 SIF precursors relevant to the construction industry (Alexander et al. 2017a, b; EEI 2019; see Table 1). To facilitate the prioritization, the expert panel, through discussions, grouped some of the precursors with similar characteristics (e.g., significant overtime and fatigue). This resulted in a list of 13 candidate differentiators (see Table 3).
Number | Candidate SIF differentiators |
---|---|
1 | Perceived safety culture, safety attitudes, workers inactive in safety |
2 | Fatigue, significant overtime |
3 | No/poor pretask plan or discussion specific to work |
4 | Limited safety supervision, poor quality, or inexperienced foreman |
5 | Distraction |
6 | Congestion |
7 | Abnormal schedule/productivity pressure |
8 | Risk normalization |
9 | Improvisation, poor plan to address change, stop work execution |
10 | Knowledge of safe work procedures, rules, and procedures |
11 | Familiarity with the task, departure from routine |
12 | Poor hazard recognition |
13 | Lack of control barriers, line of fire uncontrolled |
The panelists met through four sessions to prescreen, discuss, and prioritize the potential differentiators. This number of sessions was adequate to balance the logistical constraints of the focus group participants and ensure that every hypothesized factor was clearly defined before making a group decision about which of these would be further tested. After iterative rounds of discussions, seven potential SIF differentiators were selected by majority vote: () unfamiliarity with task; () risk normalization; () poor hazard recognition; () absent or not followed work plan; () absent direct controls; () poor response to change; and () unavailable resources.
These were used as variables for the subsequent research steps. Unsurprisingly, most of the variables were human factors such as unfamiliarity with task, risk normalization, and poor response to change, as they are most proximal to any incident.
Collecting Empirical Case Data with a Retrospective Investigation Template
Once the hypothesized SIF differentiators were selected, the team created a standardized data collection template and protocol to facilitate the collection of empirical data from the field. For each potential SIF differentiator, a set of questions was created by adapting the work of Alexander et al. (2017a, b). The seven hypothesized differentiators, their definitions, and the protocol questions are detailed in Table 4. From this set of questions, a retrospective investigative data collection template was created to capture all relevant information pertaining to the incident. Data were collected using this template and were populated with information gathered from interviewing the personnel involved in the incident, reviewing photos, and accessing official investigation reports. The triangulation of these various forms of data helped to identify inconsistencies and mitigate hindsight bias. For instance, one case involved a worker that fell from an unsecured ladder. When interviewed, the worker attributed the incident to not having a person holding the ladder; however, further investigation revealed that the ladder used lacked shoes and was supported on uneven ground, mitigating bias by uncovering a missing control that was previously overlooked.
Potential differentiator | Definitions | Protocol questions |
---|---|---|
Unfamiliarity with task | Workers are unfamiliar with task expectations or performance standards. | How long have you been doing this task? |
How often do you perform this task with this configuration? | ||
Risk normalization | Drift toward feelings of comfort around a hazard over time that may increase risk-taking behavior. | Since the first time you performed this task, how has the task changed? |
What do you think about a new worker being assigned to this task? | ||
Before the incident occurred, how comfortable were you with this task? | ||
Poor hazard recognition | Workers do not recognize hazards or correctly evaluate or understand the severity of risks. | What hazards were identified before the incident? |
What method was used to identify the hazards? | ||
Which life-threatening hazards were emphasized before the incident? | ||
Before the incident, describe any discussions that you had about the hazard(s) that caused the incident. | ||
What surprised you about this hazard and the incident? | ||
Absent or not followed work plan | A written or verbal work plan is absent, inadequate, infeasible, or violated. A work plan is a description of the logical sequence of work indicating exactly how a job is to be carried out in a way that secures health and safety and includes all the control measures. | Describe the work plan for this task. |
How was the task performed? | ||
If you deviated from the work plan, please explain why? | ||
If the work plan was followed, would the outcome have been different? Why? | ||
How would you suggest changing the work plan in the future? | ||
What could have changed to improve the rules, procedures, or work plan? | ||
Absent direct controls | Missing or insufficient necessary direct controls against one or more hazards. A direct control is a physical barrier or condition that (1) is targeted to the hazard(s); (2) mitigates the effects of energy when installed and used properly; and (3) works if someone makes a mistake. | Describe any direct controls that were present. |
How was the adequacy of the controls verified? | ||
What other controls would have prevented this incident from happening? | ||
Have you ever had to modify the controls for this task in the past? If so, why? | ||
Poor response to change | Inadequate response to a change after work starts. (Preexisting conditions are not examples of changes—e.g., unstable pole). A change is defined as a significant deviation from initial conditions that impacts the execution of the work plan. | What happened today that was different than expected or planned? |
How might the change have contributed to the incident? | ||
When and how did you notice the change? | ||
How often does this change occur? | ||
How have you responded to changes like these in the past? | ||
When was the last time you stopped work to formally replan a task? | ||
Please describe how you replanned and were confident that work was safe to restart. | ||
Unavailable resources | Essential resources are not present in reasonable proximity of the work performed. | Describe the condition of the equipment and tools that were used on the day of the incident. |
What other resources (time, equipment, tools, etc.) would have made your job easier or safer that day? | ||
How do you obtain tools and resources if they are not present or adequate? | ||
How do you troubleshoot, and access information related to your task? | ||
How often does your supervisor assist you in obtaining tools, resources, and information? |
The data collection template ensured consistency and replicability of the data collection and helped to mitigate the potential impacts of subjective interpretations, demand characteristics, and experiential biases. For example, leading questions were avoided that could potentially introduce confirmation bias (Klayman 1995). Additionally, when collecting data retrospectively for an incident, instead of asking workers if they thought a work plan was present, they were asked to describe how the task was performed the day of the incident or what methods were used to identify the hazards. This ensured that workers expressed their views openly without fear of retaliation from their company. Because the protocol was retrospective, data that were unverifiable or based on vague recollections were not included. Finally, only incidents from the past two years were considered to ensure consistency and relevancy within the data.
In total, data were collected for 38 past incidents. The academic team members reviewed each case to ensure formatting and tense was consistent across them all and any information alluding to the actual outcome (e.g., SIF, PSIF, LSI) was scrubbed before the cases were evaluated by the expert team.
Identifying the Presence of Potential SIF Differentiators in Each Case
Once the cases were received, the focus group reviewed every completed case template to determine whether each of the seven potential differentiators were present or not. After reviewing each case, the focus group would sequentially discuss the seven hypothesized SIF differentiators and whether each were present or absent in a case. For example, the written data from the data collection template was reviewed to determine whether poor response to change was a contributing factor. Since this study was conducted during the Covid-19 pandemic lockdown in the State of Colorado, part of the focus group was conducted online using the Zoom platform. The focus group participants were given time to read cases on the live Web meeting, and the case was paraphrased to ensure common understanding before discussion as recommended by Vance et al. (2022). Although there are inherent limitations of conducting focus groups online such as the lack of depth or contextual data when sharing insights (Tremblay et al. 2021), the online platform reduced attrition and coercion that commonly occurs when meeting in person (O’Nyumba et al. 2018).
After the discussion, each panelist was asked to submit an anonymous survey where they marked each potential SIF differentiator as (1) present; (2) absent; or (3) not enough information and/or not applicable to the case. The majority vote was recorded as the focus group decision, and the data were subsequently analyzed. To mitigate confirmation bias, any missing or nonapplicable information was treated as an absent factor. Importantly, the expert panel was blind to the actual outcome of the case (i.e., they did not know whether the case was a SIF, PSIF, or LSI when reviewing the case information). The academic team members and anyone involved in the completion of the case template were not involved in the focus group deliberation or assessments.
Analysis and Results
In total, the focus group analyzed 38 incidents that were distributed as follows: 13 SIF, 13 LSI, and 12 PSIF. These incidents came from the utility sector, commercial and pipeline construction, and specialized construction. Aligned with OSHA’s Construction Focus Four (Brown et al. 2021), most cases involved struck by, caught-in or between, and falls. Tables 5 and 6 show the complete data sets for the 38 incidents analyzed.
Incident number | Incident severity type | Potential differentiator (1 = Present, 0 = Absent) | ||||||
---|---|---|---|---|---|---|---|---|
Unfamiliarity with task | Risk normalization | Poor hazard recognition | Absent/not followed work plan | Absent direct controls | Poor response to change | Unavailable resources | ||
1 | L | 0 | 1 | 1 | 1 | 0 | 1 | 1 |
2 | S | 0 | 1 | 1 | 1 | 1 | 0 | 0 |
3 | L | 0 | 1 | 0 | 0 | 0 | 0 | 1 |
4 | P | 0 | 1 | 1 | 0 | 1 | 0 | 0 |
5 | S | 1 | 1 | 1 | 1 | 1 | 1 | 1 |
6 | P | 1 | 1 | 1 | 1 | 1 | 0 | 0 |
7 | P | 1 | 0 | 1 | 1 | 1 | 0 | 0 |
8 | P | 0 | 0 | 0 | 0 | 1 | 0 | 0 |
9 | P | 0 | 0 | 1 | 1 | 1 | 0 | 0 |
10 | L | 0 | 1 | 1 | 0 | 1 | 0 | 0 |
11 | S | 0 | 0 | 1 | 1 | 1 | 1 | 0 |
12 | P | 0 | 1 | 1 | 1 | 1 | 0 | 1 |
13 | L | 0 | 0 | 0 | 0 | 0 | 0 | 0 |
14 | S | 1 | 1 | 1 | 1 | 1 | 0 | 0 |
15 | P | 1 | 1 | 1 | 1 | 1 | 1 | 0 |
16 | L | 0 | 1 | 0 | 0 | 0 | 0 | 0 |
17 | S | 1 | 1 | 1 | 1 | 1 | 1 | 1 |
18 | L | 1 | 1 | 1 | 0 | 0 | 1 | 0 |
19 | P | 0 | 1 | 1 | 1 | 1 | 1 | 1 |
Note: For the severity type row, L = LSI; P = PSIF; and S = SIF.
Incident number | Incident severity type | Potential differentiator (1 = Present, 0 = Absent) | ||||||
---|---|---|---|---|---|---|---|---|
Unfamiliarity with task | Risk normalization | Poor hazard recognition | Absent/not followed work plan | Absent direct controls | Poor response to change | Unavailable resources | ||
20 | S | 0 | 1 | 1 | 1 | 1 | 0 | 0 |
21 | S | 0 | 0 | 1 | 1 | 1 | 1 | 0 |
22 | L | 0 | 1 | 1 | 1 | 0 | 0 | 0 |
23 | S | 0 | 1 | 1 | 1 | 1 | 0 | 0 |
24 | P | 0 | 1 | 1 | 1 | 1 | 0 | 0 |
25 | P | 0 | 1 | 1 | 0 | 1 | 0 | 0 |
26 | S | 0 | 1 | 1 | 1 | 1 | 0 | 0 |
27 | P | 0 | 1 | 1 | 1 | 1 | 0 | 0 |
28 | S | 0 | 1 | 1 | 1 | 1 | 0 | 0 |
29 | S | 0 | 1 | 1 | 0 | 1 | 0 | 0 |
30 | L | 0 | 1 | 0 | 0 | 0 | 0 | 0 |
31 | L | 0 | 1 | 0 | 1 | 0 | 0 | 0 |
32 | S | 1 | 0 | 1 | 1 | 1 | 1 | 1 |
33 | S | 0 | 1 | 0 | 1 | 1 | 1 | 0 |
34 | P | 0 | 1 | 1 | 1 | 1 | 1 | 0 |
35 | L | 0 | 1 | 1 | 0 | 0 | 1 | 1 |
36 | L | 0 | 1 | 1 | 0 | 0 | 0 | 0 |
37 | L | 0 | 0 | 1 | 0 | 0 | 0 | 0 |
38 | L | 0 | 1 | 0 | 0 | 0 | 0 | 0 |
Note: For the severity type row, L = LSI; P = PSIF; and S = SIF.
Analysis
Using the data from the focus group, statistical tests were conducted to determine the extent to which the seven hypothesized factors differentiated SIF, PSIF, and LSI. When selecting the proper statistical tests, the variable type (e.g., continuous, categorical, etc.), distribution, and sample size were considered. A Fisher’s exact test was used to test the proportion of countable independent observations meeting a given condition, such as the presence of a potential SIF differentiator within incidents of various types (i.e., SIFs, LSI, or PSIFs) against the observations that do not meet such condition, where the potential SIF differentiator was absent in each incident type. Therefore, there were 14 statistical tests needed, seven for each of the two null hypotheses (NH) explored in this study (to match the seven hypothesized factors , , , , , , and ) as illustrated next:
•
NH1: The likelihood of factor being found in SIFs is equal to its presence in LSIs (for to 7).
•
NH2: The likelihood of factor being found in SIFs is equal to its presence in PSIFs (for to 7).
Rejecting the null hypothesis implies that there is an association between incident type and whether a factor was present or not (i.e., the factor is a differentiator of SIF). To use the Fisher’s exact test, the data were cross tabulated in a contingency table where each cell shows the frequency count of incidents that correspond to each category of the two dichotomous variables in our study (i.e., incident type and presence of a potential SIF differentiator). To illustrate, Table 7 shows a contingency table for one hypothesized differentiator, unfamiliarity with task. Although a chi-square () test is typically suitable for count or discrete observations, a Fisher’s exact test was used because it provides a more accurate estimate for smaller samples sizes (Connelly 2016).
Incident type/presence of a potential SIF differentiator | Unfamiliarity with task present | Unfamiliarity with task absent | Row totals |
---|---|---|---|
LSI | 1 | 12 | 13 |
SIF | 4 | 9 | 13 |
Column totals | 5 | 21 | 26 |
A power analysis using RStudio was conducted to assess the statistical power of this study. Using an alpha level of 0.05, the sample size of SIFs (), LSIs (), and PSIFs () yielded a statistical power above 0.90. This result indicates that our test had at least a 90% chance of detecting a significant difference in the proportion of hypothesized factors present in each incident type, assuming that there is a true difference in their proportions.
Key Results
The statistical tests produced insights related to the two primary research questions. Although the sample size was small, Fisher’s exact tests provided compelling answers with high statistical significance.
1.
What are the differences, if any, in the proximal causes of serious injuries and fatalities (SIFs) and low-severity injuries (LSIs)?
Two of the seven hypothesized factors were shown to statistically differentiate SIFs from LSIs: (1) absent or not followed work plan (); and (2) absent direct controls (). Because the odds ratio for these two differentiators was significantly larger than 1, the likelihood of encountering these in SIFs was significantly higher than seeing them in LSIs. That is, these two factors were involved in a much greater proportion of SIF cases than LSIs while the other five factors were equally present in both SIFs and LSIs. Table 8 shows the percentage of each potential SIF differentiator present in all SIFs () and LSIs () analyzed, along with the -values and the 95% confidence intervals for the odds ratio of SIF frequency relative to LSI.
Potential SIF differentiator | Presence in SIFs (%) | Presence in LSIs (%) | Odds ratio (SIFs/LSIs) | ||
---|---|---|---|---|---|
-value | 95% CI lower bound | 95% CI upper bound | |||
Unfamiliarity with task | 31 | 8 | 0.32 | 0.40 | 283.55 |
Risk normalization | 77 | 85 | 1.00 | 0.04 | 6.61 |
Poor hazard recognition | 92 | 54 | 0.07 | 0.87 | 509.80 |
Absent or not followed work plan | 92 | 23 | 2.92 | 1,846.80 | |
Absent direct controls | 100 | 8 | 11.01 | ∞ | |
Poor response to change | 38 | 23 | 0.67 | 0.29 | 17.25 |
Unavailable resources | 23 | 23 | 1.00 | 0.11 | 9.40 |
To better illustrate this finding, Fig. 3 provides the partial results for absent direct controls compared to unavailable resources. The small sample suggests that the odds that a SIF involves a missing direct control is about two orders of magnitude higher than an LSI. As illustrated, the factor unavailable resources was equally present in both SIF and LSI, and the odds ratio was exactly one (i.e., no difference). This indicates that unavailable resources is an issue for safety broadly, but not a concern unique to SIFs.
2.
What are the differences, if any, in the proximal causes of serious injuries and fatalities (SIFs) and potential serious injuries and fatalities (PSIFs)?

There were no statistical differences between SIFs and PSIFs for any of the seven potential differentiators. Table 9 summarizes the overall results and shows the percentage of each potential SIF differentiator present in all SIFs () and PSIFs (), along with the -values and the 95% confidence intervals for the odds ratio of SIF frequency relative to PSIF. This is strong empirical evidence that supports the popular notion that companies may learn from PSIFs as a means to prevent SIFs.
Potential SIF differentiator | Presence in SIFs (%) | Presence in PSIFs (%) | Odds ratio (SIFs/PSIFs) | ||
---|---|---|---|---|---|
-value | 95% CI lower bound | 95% CI upper bound | |||
Unfamiliarity with task | 31 | 25 | 1.00 | 0.17 | 11.75 |
Risk normalization | 77 | 75 | 1.00 | 0.12 | 10.54 |
Poor hazard recognition | 92 | 92 | 1.00 | 0.01 | 92.61 |
Absent or not followed plan | 92 | 75 | 0.32 | 0.25 | 226.79 |
Absent direct controls | 100 | 100 | 1.00 | — | — |
Poor response to change | 38 | 33 | 1.00 | 0.18 | 8.87 |
Unavailable resources | 23 | 17 | 1.00 | 0.14 | 21.33 |
Limitations
As with any study based on human interpretation, there are several limitations of the results. First, a convenience sample of volunteering safety experts and companies limits the external validity of the results. Although the authors captured a broad range of industry sectors within the cases examined, a larger and more diverse set of incidents may produce more generalizable conclusions. Second, a larger repository of cases may allow for the testing of differences for categories of injury types, part of the body affected, and other confounding factors. Third, the retrospective data collection template may be subject to confirmation bias because answers were unavoidably reconstructed from previous incident investigation reports and worker interviews. Although strict rules and a standardized data collection tool were created, confirmation bias could be reduced, but not eliminated. Fourth, although the combination of focus groups and surveys has been effective in addressing assumptions and uncertainties (Abu-Taleb and Murad 1999), it was challenging to eliminate survey responses grounded in incorrect technical assumptions. In an ideal setting, a Delphi procedure would have been implemented to include controlled feedback and private reflections over iterative rounds to build consensus. However, Covid-19 pandemic restrictions made such controls practically infeasible. Fifth, this work does not provide causal explanations of the two SIF differentiators, nor does it examine potential confounding variables and extraneous factors. These are suggested as topics for future research. For example, researchers may wish to explore the reasons why some factors are more present in SIFs than in LSIs by examining a greater number of cases or by further analyzing PSIFs, which may exist in greater numbers than SIFs. Finally, future studies should challenge this work and test other potential differentiators and extend the study to geographies outside of North America.
Discussion and Conclusions
Faced with compelling evidence that the ratio of fatal and nonfatal injuries is not constant, there has been a desire to identify what is different about SIFs. Within the safety profession, the idea that the things that hurt people are not the same as the things that kill people has begun to replace the antiquated view that SIFs will be prevented by addressing LSIs. To contribute to the body of knowledge, this study involved hypothesizing proximal factors that may differentiate SIFs from LSIs, collecting empirical data from real incident cases, evaluating the cases with an expert focus group, and conducting statistical tests to determine the extent to which any hypothesized factors differentiate SIFs from LSIs. Several key findings will help support SIF-focused prevention efforts that may address the plateau in fatality rates observed in North America.
Finding 1: Absent or not followed work plan is a key differentiator of SIFs.
Proper work planning and execution is well documented as a vital safety practice (Borys 2012; Mroszczyk 2015; Sawacha et al. 1999). In fact, issues with work planning, discipline, and execution have been repeatedly identified as contributing factors to SIFs (Cohen 1976; Alexander et al. 2017a, b; Winge et al. 2019; Mirzaei Aliabadi et al. 2020). However, this study was the first to identify this factor as being possibly unique to SIFs. In review of the cases, it was evident that in most SIF and PSIF cases, the issues in planning and execution revolved around the control of a specific and salient hazard. However, this was an issue for only one of the 13 LSI cases studied. The implication is that proper work planning and execution is needed to specifically address the control of high-energy hazards, which may be a cornerstone of a program that targets SIFs. This finding provides the impetus for further investigations that seek to elucidate on the antecedents (e.g., characteristics of a comprehensive work plan, understanding of a work plan across different stakeholders) to absent or poorly executed work plans, which could help in designing effective interventions.
Finding 2: Absent direct controls is a key differentiator of SIFs.
Related to finding 1, a missing or insufficient direct control almost always precedes a SIF but is almost never involved in an LSI. That is, LSIs often occurred in situations where no specific identifiable control was missing. When dealing with hazards associated with environmental conditions (such as uneven surfaces or dust) or routine movements, a singular physical control was rarely identified. With most LSIs, it is difficult to propose a necessary physical safeguard and perform construction tasks in a dynamic environment.
Interestingly, the factors absent direct controls and absent or not followed work plan were almost always involved in the same SIF cases (i.e., they covaried). This suggests that they may be combined into one factor in future research and in practical application. To broaden the previous statement, the implication of these two key findings is that organizations should focus on ensuring the presence of direct controls against high-energy hazards through effective work planning and execution. This supports the recent research that suggests that safety should be measured by the extent to which life-threatening hazards are observed to be controlled (Oguz Erkal and Hallowell 2023). It also provides practitioners with an actionable factor to prioritize when focusing on SIF prevention efforts.
Finding 3: The human factors studied were not differentiators of SIF.
Perhaps most surprisingly, the other five hypothesized factors were not found to be significant. Although the expert panel had strong reasoning for hypothesizing unfamiliarity with the task, risk normalization, poor hazard recognition, and other human factors as differentiators, the study found that they were equally present in both SIFs and LSIs. This suggests that, although human factors are prevalent causal factors of injuries, they are not differentiators of SIF. The implication is that generalized safety practices that focus on human behavior might be important for injury prevention broadly, but that they may not help to target SIFs directly. This is interesting because of the historical emphasis of research and practice on human behavior and error precursors (e.g., Reason 1990; Senders and Moray 1991; Shappell and Wiegmann 1997).
Finding 4: PSIFs and SIFs happen for the same reasons.
Another important but unsurprising finding was that none of the factors examined differentiated SIFs and PSIFs. That is, the seven potential SIF differentiators were present in approximately equal proportion in both SIFs and PSIFs indicating that, at least for the factors studied here, these two incident types involved the same contributing factors. Although practitioners have long recommended studying PSIFs (e.g., Martin and Black 2015), the results presented here provide enhanced empirical evidence that PSIFs can be leveraged as part of a SIF prevention strategy. The implication is that organizations can greatly expand their SIF prevention programs by collecting and learning from PSIF incidents, which is extremely helpful since actual SIFs are exceedingly rare.
The findings presented here may be useful to those who attempt to combat the observed plateau in fatality rates by addressing SIFs directly. As companies focus their safety programs, the results suggest that the control of high-energy hazards through effective work planning, discipline, and execution should be a primary focus. Future researchers should challenge these findings and expand on this study by examining additional factors and testing the efficacy of different approaches to SIF prevention.
Data Availability Statement
Some or all data, models, or code generated or used during the study are proprietary or confidential in nature and may only be provided with restrictions.
Acknowledgments
The authors would like to thank the Construction Safety Research Alliance (CSRA) and its members who participated in this study for providing continuous support, dedication, and insight.
References
Abu-Taleb, M. F., and M. M. Murad. 1999. “Use of focus groups and surveys to evaluate water conservation campaign.” J. Water Resour. Plann. Manage. 125 (2): 94–99. https://doi.org/10.1061/(ASCE)0733-9496(1999)125:2(94).
Alexander, D., M. Hallowell, and J. Gambatese. 2017a. “Precursors of construction fatalities. I: Iterative experiment to test the predictive validity of human judgement.” J. Constr. Eng. Manage. 143 (7): 04017023. https://doi.org/10.1061/(ASCE)CO.1943-7862.0001304.
Alexander, D., M. Hallowell, and J. Gambatese. 2017b. “Precursors of construction fatalities. II: Predictive modeling and empirical validation.” J. Constr. Eng. Manage. 143 (7): 04017024. https://doi.org/10.1061/(ASCE)CO.1943-7862.0001297.
Alizadeh, S. S., S. B. Mortazavi, and M. Mehdi Sepehri. 2015. “Assessment of accident severity in the construction industry using the Bayesian theorem.” Int. J. Occup. Saf. Ergon. 21 (4): 551–557. https://doi.org/10.1080/10803548.2015.1095546.
Ballard, G. M. 1990. “Precursor analysis-a way of testing safety analysis?” Reliab. Eng. Syst. Saf., 27 (1): 77–89. https://doi.org/10.1016/0951-8320(90)90032-I.
Bayona, A., S. Bhandari, M. Hallowell, F. Sherratt, J. M. Bailey, and J. Upton. 2023. “What is a serious injury? A model for defining serious injuries & fatalities.” Prof. Saf. 68 (9): 22–30.
Bellamy, L. J. 2015. “Exploring the relationship between major hazard, fatal and non-fatal accidents through outcomes and causes.” Saf. Sci. 71 (Jan): 93–103. https://doi.org/10.1016/j.ssci.2014.02.009.
Bhandari, S., and M. R. Hallowell. 2021. “Identifying and controlling biases in expert-opinion research: Guidelines for variations of Delphi, nominal group technique, and focus groups.” J. Manage. Eng. 37 (3): 04021015. https://doi.org/10.1061/(ASCE)ME.1943-5479.0000909.
Bird, F. E., Jr. 1966. Damage control: A new horizon in accident prevention and cost improvement. New York: American Management Association.
Bleijenbergh, I., H. Korzilius, and P. Verschuren. 2011. “Methodological criteria for the internal validity and utility of practice oriented research.” Qual. Quant. 45 (Jan): 145–156. https://doi.org/10.1007/s11135-010-9361-5.
BLS (US Bureau of Labor Statistics). 2022. “Injuries, illnesses, and fatalities.” Accessed August 2, 2023. https://www.bls.gov/web/osh/table-1-industry-rates-national.htm.
Borys, D. 2012. “The role of safe work method statements in the Australian construction industry.” Saf. Sci. 50 (2): 210–220. https://doi.org/10.1016/j.ssci.2011.08.010.
Bouma, G. D., and R. Ling. 2004. The research process. 5th ed. Oxford, UK: Oxford University Press.
Brown, S., W. Harris, R. D. Brooks, and X. S. Dong. 2021. Fatal injury trends in the construction industry. Silver Spring, MD: The Center for Construction Research and Training.
Busch, C., C. Usrey, J. Loud, N. Goodell, and R. A. Carrillo. 2021. “Serious injuries & fatalities: Why are they constant while injury rates decrease?” Prof. Saf. 66 (1): 26–31.
Cohen, H. H. 1976. “Causal factors in select work accidents: Investigation of worker fatalities.” In Proc., Human Factors Society Annual Meeting, 240–242, Los Angeles: SAGE.
Connelly, L. M. 2016. “Fisher’s exact test.” Med. Surg. Nurs. 25 (1): 58–60.
Cooper, M. D. 2019. “The efficacy of industrial safety science constructs for addressing serious injuries & fatalities (SIFs).” Saf. Sci. 120 (Dec): 164–178. https://doi.org/10.1016/j.ssci.2019.06.038.
Cortini, M., T. Galanti, and S. Fantinelli. 2019. “Focus group discussion: How many participants in a group?” Encyclopaideia 23 (54): 29–43. https://doi.org/10.6092/issn.1825-8670/9603.
Dawson, S., L. Manderson, and V. L. Tallo. 1993. A manual for the use of focus groups. Boston: International Nutrition foundation for developing countries.
Dunlap, E. S., B. Basford, and M. L. DePoy Smith. 2019. “Remodeling Heinrich: An application for modern safety management.” Pro. Saf. 64 (5): 44–52.
EEI (Edison Electric Institute). 2019. Precursor research summary. The power to prevent serious injuries & fatalities. Washington, DC: EEI.
Groen, F., C. Everett, A. Hall, and S. Insley. 2011. “NASA accident precursor analysis handbook.” Accessed September 19, 2023. https://ntrs.nasa.gov/api/citations/20120003292/downloads/20120003292.pdf.
Groves, W. A., V. J. Kecojevic, and D. Komljenovic. 2007. “Analysis of fatalities and injuries involving mining equipment.” J Safety Res 38 (4): 461–470. https://doi.org/10.1016/j.jsr.2007.03.011.
Hallowell, M., M. Quashne, R. Salas, B. MacLean, and E. Quinn. 2021. “The statistical invalidity of TRIR as a measure of safety performance.” Prof. Saf. 66 (4): 28–34.
Hallowell, M. R. 2020. Safety classification and learning model. Washington, DC: Edison Electric Institute.
Hallowell, M. R., D. Alexander, and J. A. Gambatese. 2017. “Energy-based safety risk assessment: Does magnitude and intensity of energy predict injury severity?” Construct. Manage. Econ. 35 (1–2): 64–77. https://doi.org/10.1080/01446193.2016.1274418.
Hallowell, M. R., and J. A. Gambatese. 2010. “Qualitative research: Application of the Delphi method to CEM research.” J. Constr. Eng. Manage. 136 (1): 99–107. https://doi.org/10.1061/(ASCE)CO.1943-7862.0000137.
Haslam, R. A., S. A. Hide, A. G. Gibb, D. E. Gyi, T. Pavitt, S. Atkinson, and A. R. Duff. 2005. “Contributing factors in construction accidents. Appl. Ergon. 36 (4): 401–415. https://doi.org/10.1016/j.apergo.2004.12.002.
Heinrich, H. W. 1931. Industrial accident prevention; a scientific approach. New York: McGraw-Hill.
Heinrich, H. W. 1941. Industrial accident prevention. A scientific approach. 2nd ed. New York: McGraw-Hill.
Hubbard, R. K. B., and J. T. Neil. 1986. “Major-minor accident ratios in the construction industry.” J. Occup. Accid. 7 (4): 225–237. https://doi.org/10.1016/0376-6349(86)90015-5.
Khanzode, V. V., J. Maiti, and P. K. Ray. 2012. “Occupational injury and accident research: A comprehensive review.” Saf. Sci. 50 (5): 1355–1367. https://doi.org/10.1016/j.ssci.2011.12.015.
Klayman, J. 1995. “Varieties of confirmation bias.” Psychol. Learn. Motivation 32 (Jan): 385–418. https://doi.org/10.1016/S0079-7421(08)60315-1.
Krause, T. R., and G. Murray. 2012. “On the prevention of serious injuries and fatalities.” In Proc., ASSE Professional Development Conf. and Exposition. Laguna Beach, CA: ASSE.
Laughery, K. R., and K. P. Vaubel. 1993. “Major and minor injuries at work: Are the circumstances similar or different?” Int. J. Ind. Ergon. 12 (4): 273–279. https://doi.org/10.1016/0169-8141(93)90097-W.
Leung, M. Y., J. Yu, and Y. S. Chan. 2014. “Focus group study to explore critical factors of public engagement process for mega development projects.” J. Constr. Eng. Manage. 140 (3): 04013061. https://doi.org/10.1061/(ASCE)CO.1943-7862.0000815.
Love, P. E., P. Teo, J. Smith, F. Ackermann, and Y. Zhou. 2019. “The nature and severity of workplace injuries in construction: Engendering operational benchmarking.” Ergonomics 62 (10): 1273–1288. https://doi.org/10.1080/00140139.2019.1644379.
Lozada-Larsen, S. R., and K. R. Laughery Sr.1987. “Do identical circumstances precede minor and major injuries?” In Proc., Human Factors Society Annual Meeting, 200–204. Los Angeles: SAGE.
Manuele, F. A. 2002. Heinrich revisited: Truisms or myths. Itasca, IL: US National Safety Council.
Manuele, F. A. 2008. “Serious injuries & fatalities a call for a new focus on their prevention.” Prof. Saf. 53 (12): 32–39.
Manuele, F. A. 2011. “Reviewing Heinrich.” Prof. Saf. 56 (10): 52–61.
Marshall, P., A. Hirmas, and M. Singer. 2018. “Heinrich’s pyramid and occupational safety: A statistical validation methodology.” Saf. Sci. 101 (Jan): 180–189. https://doi.org/10.1016/j.ssci.2017.09.005.
Martin, D. K., and A. A. Black. 2015. “Preventing serious injuries & fatalities. Study reveals precursors & paradigms.” Prof. Saf. 60 (9): 35–43.
McSween, T., and D. J. Moran. 2017. “Assessing and preventing serious incidents with behavioral science: Enhancing Heinrich’s triangle for the 21st century.” J. Organ. Behav. Manage. 37 (3–4): 283–300. https://doi.org/10.1080/01608061.2017.1340923.
Mirzaei Aliabadi, M., H. Aghaei, O. Kalatpour, A. R. Soltanian, and A. Nikravesh. 2020. “Analysis of human and organizational factors that influence mining accidents based on Bayesian network.” Int. J. Occup. Saf. Ergon. 26 (4): 670–677. https://doi.org/10.1080/10803548.2018.1455411.
Mitchell, R. J., A. Williamson, and B. Molesworth. 2016. “Application of a human factors classification framework for patient safety to identify precursor and contributing factors to adverse clinical incidents in hospital.” Appl. Ergon. 52: 185–195. https://doi.org/10.1016/j.apergo.2015.07.018.
Morewedge, C. K., H. Yoon, I. Scopelliti, C. W. Symborski, J. H. Korris, and K. S. Kassam. 2015. “Debiasing decisions: Improved decision making with a single training intervention.” Policy Insights Behav. Brain Sci. 2 (1): 129–140. https://doi.org/10.1177/2372732215600886.
Mroszczyk, J. W. 2015. “Improving construction safety: A team effort.” Prof. Saf. 60 (6): 55–68.
NAE (National, A. O. E.). 2004. Accident precursor analysis and management: Reducing technological risk through diligence. Washington, DC: National Academies Press.
Nahari, G., and G. Ben-Shakhar. 2013. “Primacy effect in credibility judgements: The vulnerability of verbal cues to biased interpretations.” Appl. Cognit. Psychol. 27 (2): 247–255. https://doi.org/10.1002/acp.2901.
Nussey, C. 1980. “Studies of accidents leading to minor injuries in the UK coal mining industry.” J. Occup. Accid. 2 (4): 305–323. https://doi.org/10.1016/0376-6349(80)90006-1.
Oguz Erkal, E. D., and M. Hallowell. 2023. “Moving beyond TRIR: Measuring & monitoring safety performance with high-energy control assessments.” Prof. Saf. 68 (5): 26–35.
O’Nyumba, T., K. Wilson, C. J. Derrick, and N. Mukherjee. 2018. “The use of focus group discussion methodology: Insights from two decades of application in conservation.” Methods Ecol. Evol. 9 (1): 20–32. https://doi.org/10.1111/2041-210X.12860.
Pereira, E., S. Ahn, S. Han, and S. Abourizk. 2020. “Finding causal paths between safety management system factors and accident precursors.” J. Manage. Eng. 36 (2): 04019049. https://doi.org/10.1061/(ASCE)ME.1943-5479.0000738.
Petersen, D. 1989. Techniques of safety management: A systems approach. New York: Aloray.
Rasmussen, J. 1997. “Risk management in a dynamic society: A modelling problem.” Saf. Sci. 27 (2–3): 183–213. https://doi.org/10.1016/S0925-7535(97)00052-0.
Reason, J. 1990. Human error. Cambridge, UK: Cambridge University Press.
Rebbitt, D. 2014. “Pyramid power: A new view of the great safety pyramid.” Prof. Saf. 59 (9): 30–34.
Salminen, S., J. Saari, K. L. Saarela, and T. Räsänen. 1992. “Fatal and non-fatal occupational accidents: Identical versus differential causation.” Saf. Sci. 15 (2): 109–118. https://doi.org/10.1016/0925-7535(92)90011-N.
Sawacha, E., S. Naoum, and D. Fong. 1999. “Factors affecting safety performance on construction sites.” Int. J. Project Manage. 17 (5): 309–315. https://doi.org/10.1016/S0263-7863(98)00042-8.
Senders, J. W., and N. P. Moray. 1991. Human error: Cause, prediction, and reduction. Hillsdale, NJ: L. Erlbaum Associates.
Shanon, H. S., and D. P. Manning. 1980. “Differences between lost-time and non-lost-time industrial accidents.” J. Occup. Accid. 2 (4): 265–272. https://doi.org/10.1016/0376-6349(80)90002-4.
Shappell, S. A., and D. A. Wiegmann. 1997. “A human error approach to accident investigation: The taxonomy of unsafe operations.” Int. J. Aviat. Psychol. 7 (4): 269–291. https://doi.org/10.1207/s15327108ijap0704_2.
Smithson, J. 2000. “Using and analysing focus groups: Limitations and possibilities.” Int. J. Soc. Res. Methodol. 3 (2): 103–119. https://doi.org/10.1080/136455700405172.
Suraji, A., A. R. Duff, and S. J. Peckitt. 2001. “Development of causal model of construction accident causation.” J. Constr. Eng. Manage. 127 (4): 337–344. https://doi.org/10.1061/(ASCE)0733-9364(2001)127:4(337).
Tang, H. 2020. Engineering research: Design, methods, and publication. Hoboken, NJ: Wiley.
Toole, T. M. 2002. “Construction site safety roles.” J. Constr. Eng. Manage. 128 (3): 203–210. https://doi.org/10.1061/(ASCE)0733-9364(2002)128:3(203).
Tremblay, S., S. Castiglione, L. A. Audet, M. Desmarais, M. Horace, and S. Peláez. 2021. “Conducting qualitative research to respond to COVID-19 challenges: Reflections for the present and beyond.” Int. J. Qual. Methods 20 (Apr): 16094069211009679. https://doi.org/10.1177/16094069211009679.
Vance, E. A., J. L. Alzen, and H. S. Smith. 2022. “Creating shared understanding in statistics and data science collaborations.” J. Stat. Data Sci. Educ. 30 (1): 54–64. https://doi.org/10.1080/26939169.2022.2035286.
Winge, S., E. Albrechtsen, and B. A. Mostue. 2019. “Causal factors and connections in construction accidents.” Saf. Sci. 112 (Feb): 130–141. https://doi.org/10.1016/j.ssci.2018.10.015.
Zhou, Z., Q. Li, and W. Wu. 2012. “Developing a versatile subway construction incident database for safety management.” J. Constr. Eng. Manage. 138 (10): 1169–1180. https://doi.org/10.1061/(ASCE)CO.1943-7862.0000518.
Zou, P. X., and G. Zhang. 2009. “Comparative study on the perception of construction safety risks in China and Australia.” J. Constr. Eng. Manage. 135 (7): 620–627. https://doi.org/10.1061/(ASCE)CO.1943-7862.0000019.
Information & Authors
Information
Published In
Copyright
This work is made available under the terms of the Creative Commons Attribution 4.0 International license, https://creativecommons.org/licenses/by/4.0/.
History
Received: Sep 19, 2023
Accepted: Feb 20, 2024
Published online: Jun 3, 2024
Published in print: Aug 1, 2024
Discussion open until: Nov 3, 2024
ASCE Technical Topics:
- Business management
- Colleges and universities
- Construction engineering
- Construction management
- Education
- Energy infrastructure
- Gas pipelines
- Human and behavioral factors
- Infrastructure
- Lifeline systems
- Occupational safety
- Oil pipelines
- Practice and Profession
- Public administration
- Public health and safety
- Safety
- Utilities
Authors
Metrics & Citations
Metrics
Citations
Download citation
If you have the appropriate software installed, you can download article citation data to the citation manager of your choice. Simply select your manager software from the list below and click Download.