Smart Irrigation Controllers in Residential Applications and the Potential of Integrated Water Distribution Systems
Publication: Journal of Water Resources Planning and Management
Volume 150, Issue 1
Abstract
Drought and population growth, especially in the western United States, are propelling a need for more efficient irrigation. Smart irrigation controllers, which interface with soil moisture, evapotranspiration (ET), or weather sensors, have been promoted as a demand-side management tool for this purpose. This paper reviews the body of research on residential smart irrigation controllers and their effectiveness. We find that smart irrigation controllers consistently reduce water demand by 15% among general users and more than 40% among indulgent users. Gaps in research include studies addressing peak demand reduction, centralized communication, data verifiability, and human factors of landscape management. Future work may develop techniques for coordinating networks of smart irrigation controllers to enable greater shifting and shaving of discretionary irrigation demands, similar to what is already happening in the electric grid, thereby creating an integrated water distribution system (IWDS). An IWDS may utilize smart irrigation controllers as direct load control devices at customer end points and interface with advanced metering infrastructure (AMI) and supervisory control and data acquisition (SCADA) systems at a central location to create more effective demand-side management (DSM) strategies for water conservation.
Practical Applications
Weather-based irrigation controllers (WBICs) have become the prevalent smart controllers used for residential irrigation scheduling. They are easy to install, have smartphone control, are easy to program, utilize local real-time weather data, prevent operating the irrigation system during rain or other adverse weather events, adjust watering schedules and duration seasonally, conserve water, and reduce water billing costs. In addition to the ease of use and benefits at the residential user level, smart irrigation controllers have the potential to provide the water utility with controls to buffer or shift peak irrigation demands. This buffering and shifting of peak demands have the potential to lower energy costs, decrease the carbon footprint, and delay costly infrastructure upgrades. With technological advancements and the proliferation of smart controllers, the future for water utilities will become more similar to the electrical grid industry. The smart controllers and technology will enable the development of the IWDS. The IWDS will allow the water utility to utilize data from AMI meters, SCADA systems, smart irrigation controllers, artificial intelligence, and other smart technology to operate more effectively and efficiently.
Introduction
The current Western United States drought dating back to 2000 is the driest 22-year span since at least 800 CE and will likely continue (Williams et al. 2022). The declining health of the Colorado River (Wheeler et al. 2022) and the Great Salt Lake (Abbott 2023) are two strong indicators of this trend. At the same time, the American West’s population and water needs are growing (Hedden-Nicely 2022; Robbins 2022), with implications for both the overall volume and the peak timing of water demand (Arunkumar and Mariappan 2011). The convergence of a changing climate and a growing water demand presents an immediate opportunity for legal reform, efficiency incentives, and significant water resources infrastructure investments (Hedden-Nicely 2022; Sowby 2022). The United States is not alone in experiencing extreme drought—Khan and Gilani (2021) observe that parts of most continents are vulnerable—and solutions may be applied elsewhere.
A particular opportunity in North America is in residential landscape irrigation, a significant end use of water (Coomes et al. 2010; DeOreo et al. 2016; Mayer et al. 2015). The US Geological Survey reports that, on a per capita basis, the Northern and Eastern states use less water and the Mountain and Western states, where outdoor watering is more common, use the greatest (Dieter et al. 2018). More particular studies show that irrigation accounts for 30%–50% or more of residential water use (Devitt et al. 2008; Haley et al. 2007; USEPA 2013, 2022a; Waskom and Neibauer 2014); in the Southwestern United States, the figure is closer to 90% (Cooley and Gleick 2009). Fig. 1 shows the breakdown of residential water use based on the study by DeOreo et al. (2016).
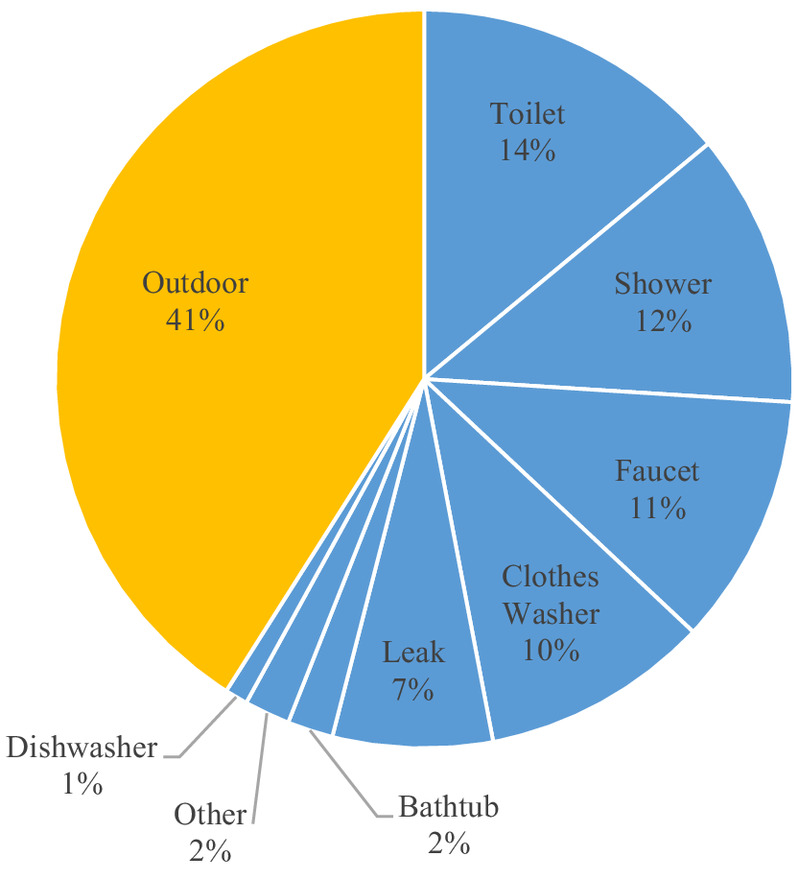
At first, all residential in-ground irrigation systems (sprinkler systems) were manually controlled (and some still are). Under manual control, a homeowner must physically turn the system on and off; the frequency and duration of watering are discretionary. Automatic timers were introduced in the 1960s. Because of the automation they offer, timers are more convenient than manual irrigation, but they often lead to overwatering because they are typically programmed for the peak growing season and are rarely adjusted to reflect seasonal changes or precipitation events. In the United States, for example, a timer-controlled irrigation system applies, on average, 47% more water than manual watering (Mayer et al. 1999).
As the next evolution of irrigation technology, smart irrigation controllers have been promoted since the late 1990s. The devices monitor soil moisture, evapotranspiration, and/or weather and optimize irrigation schedules accordingly. They thus avoid the excess watering associated with timers while still offering benefits of automation over manual watering. For these reasons, a 2016 Water Research Foundation report concluded that water utilities should encourage the use of smart irrigation controllers instead of timers (DeOreo et al. 2016). The USEPA (2022c) estimates that nearly 28 million sprinkler systems are installed across the United States, but despite the availability of the technology, less than 10% of them utilize smart controllers. In the early stages there were just a few commercially available smart controller options. Currently there are nearly 1,000 smart controllers with the WaterSense label available (USEPA 2022b), up from over 700 as reported in 2020 (Dukes 2020).
It is occasionally necessary to compress abundant literature on a specific topic and provide perspective on the state of the research in the form of a review paper (Sowby and Grigg 2022). Reviews on smart irrigation controllers, notably those by Dukes (2012, 2020), have been provided in the past, but they were limited to journal articles and did not include many valuable engineering reports and studies. Given the need to address pressing water conservation issues and learn from advancements in the electric integrated grid and its implications for integrated water distribution systems, we find it prudent to prepare a new review focused on applications of smart irrigation controllers.
To advance the state of the practice, we review here more than 80 studies on residential applications of smart irrigation controllers found through the method described subsequently. We discuss common findings from the studies, including water savings and other benefits, as well as gaps and limitations. We then recommend pathways for future research and the possibilities for integrated water distribution systems to mimic the advancements of the electric grid.
Methods
We began our review by extensively searching Scopus, Web of Science, Google Scholar, the ASCE Library, and American Water Works Association (AWWA) periodicals for relevant sources. Keywords used to query material included “smart controllers,” “water demand side management,” “water conservation,” and “residential landscape irrigation.” We then examined the references in the articles to expand our search. Agricultural studies were excluded, and generally only studies in the past 25 years were of interest specifically documenting residential landscape controller applications and not just research describing new controllers or theories. We digitally or physically verified each primary source before including it in the final list [i.e., we did not rely on citations by others, a problem noted by Chini et al. (2021)]. We excluded nearly 10 studies from this review that were not discoverable and verifiable. For each study, we cataloged the location, conditions, controller technology, baseline definition, water savings, and other pertinent information as applicable. We then used the tabulation to develop our discussion, which follows.
Results and Discussion
Summary of the Studies
The Appendix summarizes the reviewed studies. The list contains 51 journal articles, 24 reports, and five conference proceedings (80 total sources) published between 1984 and 2021, reporting on studies in 20 states across the United States and three countries (United States, Australia, Canada) as shown in Fig. 2. Fig. 3 provides a timeline.
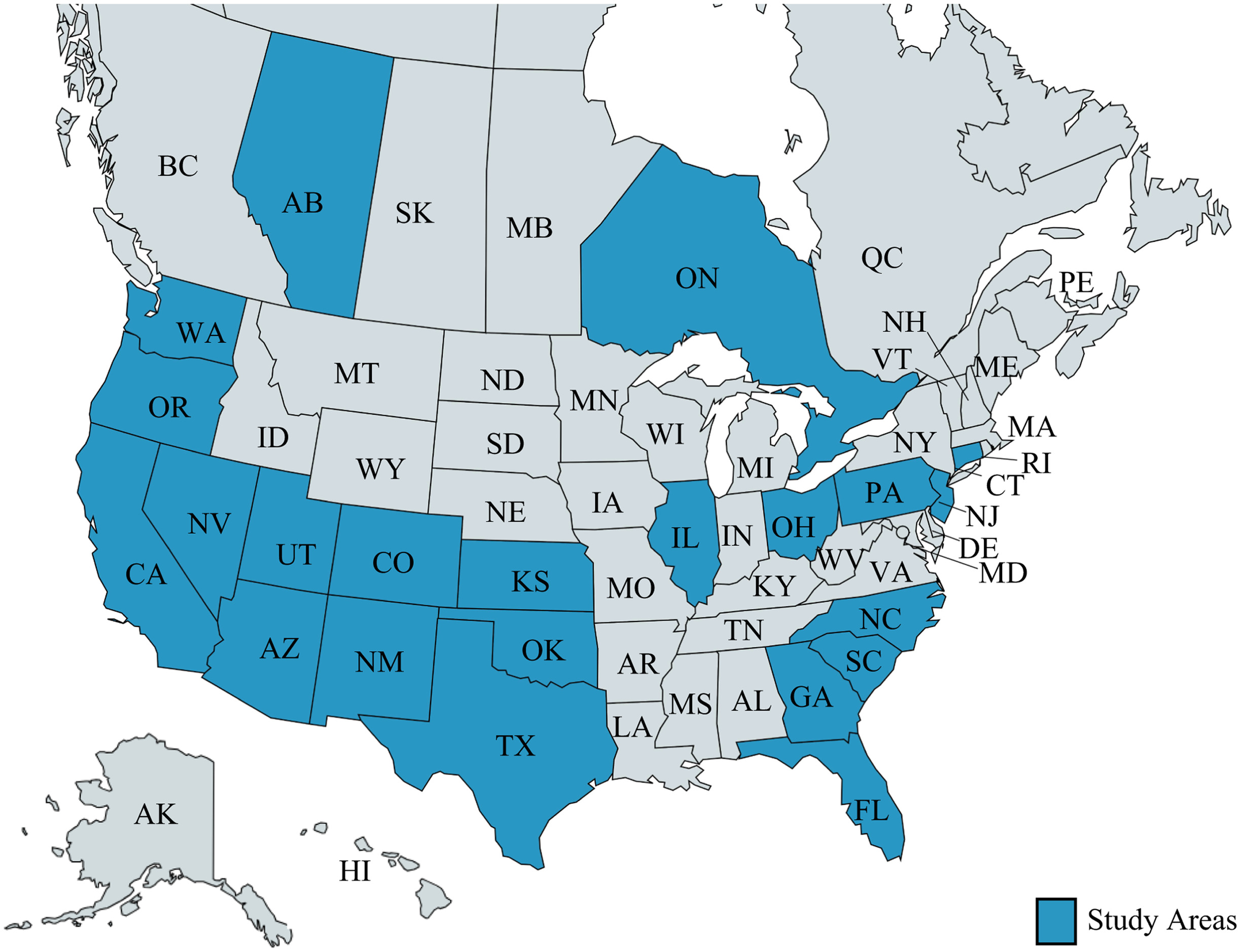
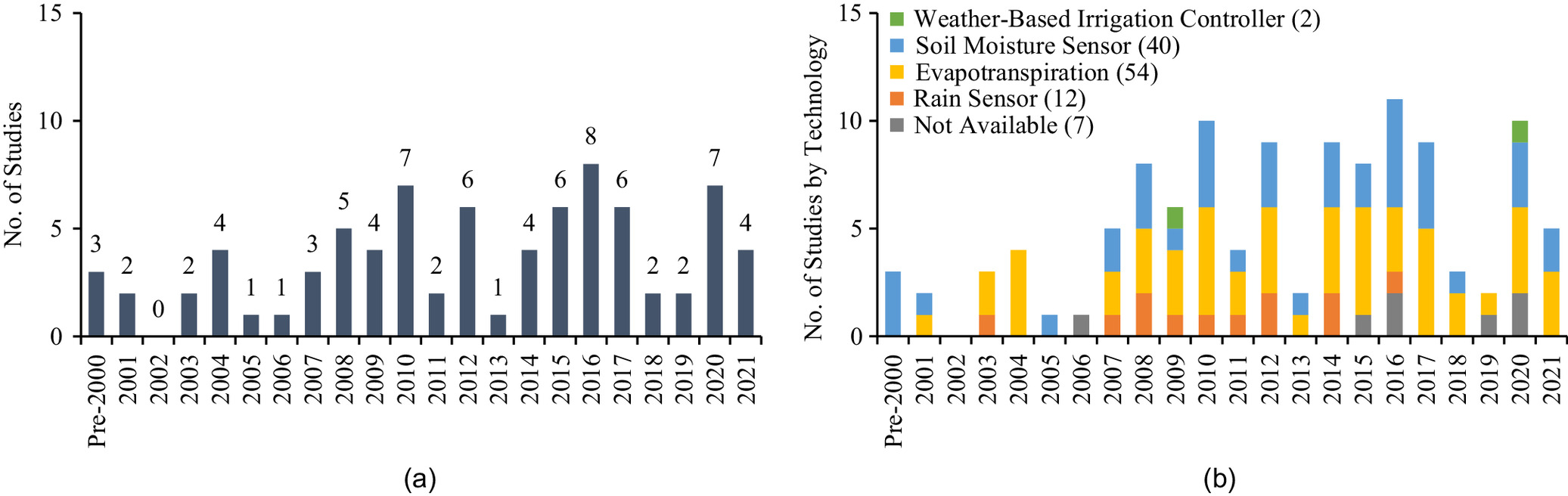
Early studies focused on determining water savings potential in controlled university and field applications. Once smart controllers were established, the studies became more complex and considered seasonal variations, specific field conditions, manufacturer comparisons, more complex statistical analyses, and human elements and preferences.
The studies consider a variety of irrigation control technologies (as either the baseline or the project), which we define here and as depicted in Fig. 4:
•
Manual: physically turning on a valve to start and stop an irrigation event.
•
Timer: automatic controller that starts and stops irrigation based on a set schedule (e.g., day, start time, and duration).
•
Rain sensor (RS): on-site device interfaced with a timer or controller that prevents irrigation during or after a rain event. These devices are not controllers but mechanisms that allow the controller to respond to weather conditions.
•
Soil moisture sensor (SMS): on-site device installed underground in the root zone that collects soil moisture data and interfaces with a controller. Irrigation can be programmed to be bypassed based on threshold soil moisture conditions.
•
Evapotranspiration (ET) controller: controller that adjusts irrigation events (bypass, frequency, or duration) based on preprogrammed historical ET data or on-site ET sensors. ET is the combination of evaporation from the soil plus transpiration from plants and depends on weather factors such as sunlight, temperature, wind, and humidity. ET varies seasonally and daily and is highest in the summer and lowest in the winter. Almost all ET controllers use the ASCE standardized reference evapotranspiration equation (Allen et al. 2005) to calculate ET, which is considered the standard for ET calculations. This method accounts for reference ET, crop ET, and many climate factors.
•
Weather-based irrigation controller (WBIC): controller that schedules irrigation to meet the landscaping needs based on current weather data (e.g., precipitation, sunlight, temperature, wind, and humidity) collected on-site or transmitted from off-site weather stations. ET principles are often used to establish and modify irrigation schedules. Conger and Dukes (2020) note that because WBICs often use ET to estimate watering needs, WBICs are commonly called ET controllers.
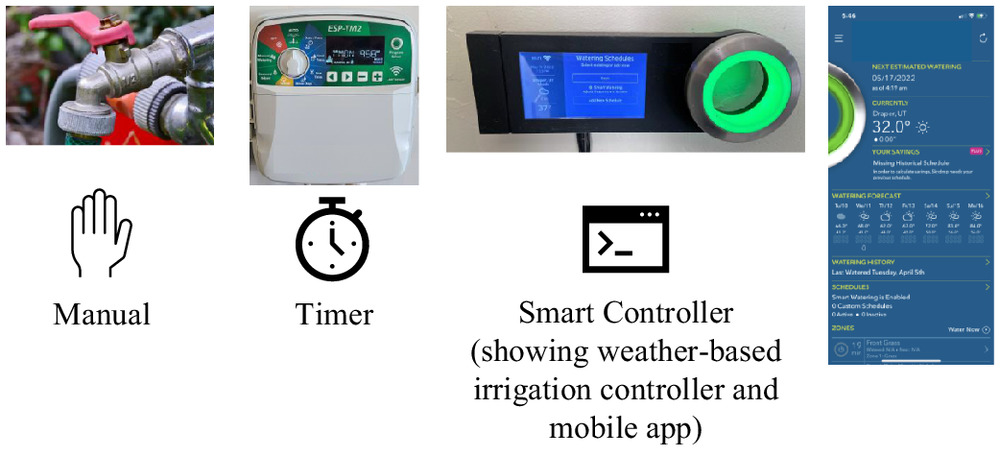
Plot and field studies consistently demonstrate that SMSs provide greater conservation potential (Cardenas-Lailhacar et al. 2008; Cardenas and Dukes 2016b; Cardenas et al. 2021; Davis and Dukes 2015a; Vick et al. 2017). Studies also demonstrate that SMSs remain reliable and have minimal operation and maintenance costs (DeOreo et al. 1997; Qualls et al. 2001). A major disadvantage of SMSs is the more difficult installation or retrofit by a homeowner because SMSs are required to be buried and typically need one sensor per zone. In addition to the sensor being buried, wires need to be run underground back to the controller. RSs and ET controllers requiring on-site instrumentation will also require greater effort and potential excavation to install wiring between the controller and the instrumentation. Studies have found that an SMS controller with an RS is more effective, saving up to an additional 45% more water (Cárdenas-Lailhacar et al. 2005; Rutland and Dukes 2012). These studies indicate the importance of using SMS and RS together.
In the past, controllers with on-site sensors were favored for being more precise to account for local conditions (Dukes 2020), but due to the complexity of SMSs, this has led to a shift from on-site sensors to signal based (WBIC), which are easier to install and do not require modification to the underground irrigation system other than replacing the old timer controller with the new controller. With the proliferation of publicly and locally available weather stations, the precision of WBICs are gaining equal ground to on-site sensors. Due to the factors listed previously with the greater complexity to SMS and RS installations, WBICs should be the preferred controller alternative for targeted education and outreach programs. Data show that customer WBIC installations are equally or even more effective than contractor-installed controllers at increasing water savings (Aquacraft 2015; Kennedy/Jenks 2010).
As stated previously, only 10% of the estimated 28 million irrigation controllers are smart controllers. This is a low percentage given there are nearly 1,000 smart controller options available (USEPA 2022b). From survey results, the major factor for low adoption rates is the cost of the smart controller (Boyer et al. 2016). Homeowners prefer smart controllers, but water rates and annual savings, water savings potential, and price perception are the most important factors in deciding to upgrade to a smart controller (Khachatryan et al. 2019, 2020; Suh et al. 2017; Zhang and Khachatryan 2019).
To overcome the cost hurdle, Spanish Fork, Utah, took a unique strategy to meet a state 25% reduction goal in water use. Spanish Fork’s approach was to provide free, professionally installed smart controllers to residents. As reported in 2019, with approximately 1,400 controllers installed, the customers have a 17% average water use savings. Additional benefits to the city include peak demand reductions enabling them to delay infrastructure upgrades (Paxton et al. 2019). As an update, Spanish Fork has installed 3,250 controllers with 250 remaining to be installed in the summer of 2022 with water savings continuing to be near 17% (Paxton, personal communication, 2022).
Water Savings Performance
The reviewed studies suggest a wide range of water savings (6% to 92%) associated with smart irrigation controllers. The locations of the reviewed studies varied widely with considerable diversity in location and climate. Studies from humid, semiarid, and arid climates demonstrated great effectiveness. While no definitive comparison is possible because the technologies, baselines, and conditions vary across the studies, some general patterns are apparent and are supported by the literature. SMS seemed to perform the best, with savings of 47%, followed by ET controllers, with savings of 30%. This is consistent with Dukes’s (2020) ET and SMS combined reported savings of 51% for plot studies and 30% for residential studies.
Negative savings indicate that more water was used because of the smart controller; such cases may be explained by a smart controller being installed on a previous underirrigated landscape. The increased irrigation usage is typically due to the smart controller functioning properly based on controller settings and applying the required theoretical irrigation requirement based on the reference ET (Aquacraft 2015; Davis and Dukes 2014, 2015b).
Another important distinction is the group of water users being targeted. Many studies report savings at 40% or higher, but Dukes (2012), Mayer et al. (2009), and Williams et al. (2014) commented that such results came from studies focusing on excessive irrigators rather than typical applications and hypothesized that a more reasonable expectation would be 10% savings or less. Still, the law of the few should not be overlooked; focusing smart controllers and other water conservation efforts toward a few excessive irrigators can be effective (Davis and Dukes 2015a, b; Mayer and DeOreo 2010; Shurtz et al. 2022). Indeed, DeOreo et al. (2016) found that about 20% of water users applied more than the theoretical ET requirement, but a few indulgent users accounted “for the bulk of excess irrigation for the whole group.”
Given the comments by others and the sources we reviewed here, we estimate that smart irrigation controllers reduce water use on the order of 40% or more when targeting excessive irrigators and 15% or less when including typical users, and that SMSs paired with RSs are the most effective devices (Cárdenas-Lailhacar et al. 2005; Rutland and Dukes 2012).
Other Benefits
Beyond saving water and customers benefiting by lowering their water utility bill by using less water for irrigation (Cardenas et al. 2020; Kleinman 2017; USEPA 2021), smart irrigation controllers help limit peak water demand, save energy, improve landscape health, and reduce runoff.
Water conservation from smart irrigation controllers can benefit the water utility in both volume and peak flow rate (Hoyenga and Reaves 2006; Mayer et al. 2018; Mayer and Smith 2017). Because water distribution systems are designed based on peak demand, the peak demand reductions may enable water utilities to modify or delay infrastructure investments (MWDOC&IRWD 2004). Mayer and Smith (2017) estimated a total of (10 MGD) peak reduction could be achieved based on data from a small study. Hoyenga and Reaves (2006) described how the Eugene Water & Electric Board (EWEB) in Oregon was over capacity in one area of its system but was able to put off emergency capital upgrades by changing only the timing of irrigation, a solution that also reduced maintenance costs and better regulated system pressures.
Because water supply requires energy (Chini and Stillwell 2018; Griffiths-Sattenspiel and Wilson 2009; Sowby and Burian 2017; Sowby and Dicataldo 2022; Zib et al. 2021), water conservation will reduce energy use and the associated carbon emissions. Mutchek and Williams (2010) noted this linkage specifically for smart irrigation controllers. Zib et al. (2021) estimated that the average operational carbon footprint of US water utilities is 0.46 kg per cubic meter of water, so every unit of water saved through smart irrigation controllers reduces carbon emissions accordingly. Because water suppliers are prone to reactively meet peak water demand with pumps (Jones and Sowby 2014), the implications for energy and/or cost savings are particularly relevant in energy markets with dynamic pricing or limited capacity.
There are some environmental benefits too. Several studies have reported that through proper irrigation application, landscapes are healthier and more resilient (Cardenas and Dukes 2016a; MWDOC&IRWD 2004; Mayer et al. 2009; Shurtz et al. 2022). Further, large field studies have documented that when landscapes receive optimum irrigation rates, runoff drops by 49% to 71% (Aquacraft 2003; Berg and Diamond 2004; MWDOC&IRWD 2004; Kennedy/Jenks 2010; Koeller 2004) and downstream water quality improves thanks to lower pollutant loads (MWDOC&IRWD 2004).
Research Gaps and Limitations
The literature review identified several research gaps. The key findings for discussion are peak demand reduction, centralized communication, human factors of landscape management (plant choice, layout, and maintenance), and data verifiability.
A few studies (Hoyenga and Reaves 2006; Kleinman 2017; Mayer and Smith 2017; Paxton et al. 2019) identified potential benefits from peak demand management through smart irrigation controllers, including cost savings from delaying future infrastructure capital facility projects, more efficient operation and maintenance, lower energy costs, and water quality improvements. Few such studies exist, however, and they have been limited in size, number of participants, scope, and objectives. Hydraulic modeling studies and larger, more complex studies are needed to determine the limitations and the maximum potential of smart controllers in peak demand reduction.
Centralized communication is greatly needed in the water sector to harness growing data streams from smart irrigation controllers, advanced metering infrastructure (AMI), and supervisory control and data acquisition (SCADA) systems. Current limitations in data synchronization do not allow for optimization of peak demand reduction and facilitation of artificial intelligence (AI) capabilities in operation and management decisions. The electric grid system uses centralized communications (Abrahamsen et al. 2021; Panda et al. 2022) to create an integrated electric grid for accurate demand forecasting and peak demand reduction management. The water sector needs to follow the lead of the electrical sector and start implementing centralized communication. Several studies describe the inaccuracies of outdoor water usage and provide methodologies for making this estimate (Boyer et al. 2014; Davis and Dukes 2015b; Devitt et al. 2008; Romero and Dukes 2016; Torbert et al. 2016). The synchronization of smart controllers and meters will allow for accurate measurement of indoor and outdoor water usage per connection and ultimately for the entire water system.
The human factor continues to be an area of study lacking sufficient evidence to establish connections between user preferences, long-term conservation, and landscape management. Dukes (2020) noted that in his 2012 review paper (Dukes 2012) there had been no studies including the human factor, but studies in the following decade started to include customer feedback and customer interaction with the controller and smartphone apps. The Spanish Fork approach (Paxton et al. 2019) of supplying free smart controllers to opt into the program needs additional follow-up to determine long-term effectiveness and whether cost and social benefits outweigh the controller costs.
Additionally, in reviewing the body of research, we could not locate some sources cited by others or could not verify some results cited by others. We had to exclude nearly 10 reports from the Appendix because we could not find the original source online, through library requests, or through direct requests to the authors. Although other researchers have cited a primary source and may have had access, we too sought the primary source and a secondary reference was not adequate. We also encountered a few inconsistencies in referenced data that could not be verified from the original source. These were cases where data from the primary source were misquoted, miscalculated, or misunderstood when presented in a secondary source. Data availability, verifiability, and reproducibility (Rosenberg and Watkins 2018) are important to ensure smart controller research can advance.
Toward Integrated Water Distribution Systems
Integrated water resource management (IWRM), as defined by the Global Water Partnership, is a process that “promotes the coordinated development and management of water, land and related resources in order to maximize economic and social welfare in an equitable manner without compromising the sustainability of vital ecosystems and the environment” (Global Water Partnership 2022). IWRM is based on the principles of social equity, economic efficiency, and environmental sustainability (de Oliveira Vieira et al. 2020). Integrated urban water management (IUWM) is a subset of IWRM with a narrower focus on water supply, drinking water, wastewater, and stormwater (Bahri 2012). The natural next step is an integrated water distribution system (IWDS) as depicted in Fig. 5.

Mayer and Smith (2017) propose to do in the water sector what the electrical utility industry has been doing since the 1950s with direct load control demand-side management (DSM) strategies. Direct load control, through an agreement between the utility and customer, allows the utility to remotely control the customer’s appliances, which are most typically an air conditioner or water heater. The utility installs a remote-control switch on the customer’s appliance for this purpose. The DSM strategy, applied across many customers, reduces stress on the grid to avoid blackouts or brownouts. DSM strategies now affect over a million water heaters and nearly a million central air conditioners (Gellings 2017). The electrical utility sector has continued to make enhancements to DSM strategies and demand forecasting to become an integrated grid.
The integrated electric grid is more than just AMI and direct load control strategies; it is the utilization of two-way electricity and communication flow of data (Judge et al. 2022). The integrated electric grid involves self-healing, congestion management, real-time pricing, reliability, security, advanced analytics with artificial intelligence, machine learning, AMI, integration of renewable energy sources, electric vehicles, and energy storage systems, making the electric grid far more complex than a water distribution system (Judge et al. 2022; Panda et al. 2022). AMI enables the two-way communication of data, but the full capabilities of smart grid infrastructure is made possible through a centralized command center controlling the communication and facilitating analysis and decisions (Abrahamsen et al. 2021).
We encourage water utilities to follow the same path as electrical utilities and develop an integrated water distribution system that can better stay within its capacity by dynamically controlling some portion of the customer demand. Similar to benefits seen in the electric grid, IWDS will help water utilities become more efficient, reduce operation and maintenance costs, and reduce peak demand. Additional benefits can be achieved from an IWDS by enhancing water conservation potential and better drought restriction enforcement and monitoring (when in effect).
We discuss the possibility of IWDS in the context of smart irrigation controllers because they are the enabling technology. Residential irrigation, unlike the public health function of indoor water supply, is a nonessential water use and has the potential for greater DSM optimization to ensure drinking water is available for indoor use. Landscape irrigation is discretionary and can occur at any time during a relatively large window (or not at all, if capacity is unavailable). For example, in a given week, depending on weather, a typical customer’s watering cycle may need to run for 1 h during a 10-h overnight window on two separate days, so there are then 35 possible 1-h slots in which it may run. Coordinated across thousands of customers with smart irrigation controllers, water demand could be spread out to best manage peak flows, storage, and pressure. The concept is analogous to what Khatami et al. (2018) described for scheduling the charging of electric vehicles to avoid spikes in power demand.
The next application phase for smart irrigation controllers is to become integrated along with AMI and SCADA systems. Smart irrigation controllers are the water utilities’ direct load control devices. Demand forecasting and machine learning capabilities (Doorn 2021; Hadjimichael et al. 2016) will be greatly enhanced in an integrated water distribution system benefiting water utilities with increased conservation potential and peak demand management strategies. A recent survey of US water utilities indicated that about 24% have experimented with AI and that automation and water conservation are among the top motivations for more water utilities to do so (Rapp et al. 2023). The integrated water distribution system can become one of the tools to reform values and ensure a cooperative future as water becomes more scarce (Hedden-Nicely 2022).
Conclusion
Motivated by the Western US drought, developments in irrigation technology, and successful concepts from the electric grid, we reviewed 80 studies on residential applications of smart irrigation controllers. We found typical savings of 15% among general users and more than 40% among indulgent users. Soil moisture sensors paired with rain sensors have demonstrated the highest water savings potential when connected with smart irrigation controllers. However, the complexity of modifying the underground irrigation system with these devices has led to a preference for weather-based irrigation controllers, which only require replacing the old timer controller with the new smart controller. We recommend that WBICs should be the preferred controller alternative for targeted education and outreach programs. Even the most efficient and top-of-the-line controller cannot compensate for improper controller setup, lack of operation and maintenance, leaks, and poor irrigation design and management (Nautiyal et al. 2015). Overall, smart irrigation controllers are an effective demand-side management tool to conserve outdoor water use and provide extensive benefits to both the residential customer and the water utility.
The literature is sparse in its coverage of how smart irrigation controllers reduce peak water demand, how centralized communication should be handled, and how to address the human elements of landscape management informed by smart controllers. We suggest these topics for further study. An important limitation in our review was the inability to verify (or even locate) certain sources or reproduce results, an aspect that should be considered in future studies.
We recommend that water utilities build on the advances of the smart integrated electric grid and more fully mimic practices that have become standard for electrical utilities. Integrated electric grids have long been using direct load control devices for demand-side management of peak power loads. Smart irrigation controllers have the potential to provide similar benefits to water utilities. As many parts of North America and other urbanized locations around the globe are dealing with water scarcity and population growth, IWDSs built on smart irrigation controllers may open the way to DSM capabilities that are better suited to the challenges of a modern sustainable water supply.
Appendix. Summary of Reviewed Studies on Residential Smart Irrigation Controllers
Study (listed chronologically) | Type | Technology | Conditions | Location | Savings | Savings comparison | Comments |
---|---|---|---|---|---|---|---|
Augustin and Snyder (1984) | Journal | SMS | Study period December 1979 through June 1983 on test plots in sandy soil | Florida | 26% | Timer | Benefit during frequent but unpredictable rainfall |
Allen (1997) | Report | SMS | Field study with 37 homes during 1996 irrigation season | Providence and Salt Lake City, Utah | 10% | Control group | Small town versus metro comparison |
DeOreo et al. (1997) | Report | SMS | 1997 field study with 23 sensors | Boulder, Colorado | 76% | Theoretical requirements | Sensors remained reliable for 5 years |
Hunt et al. (2001) | Report | ET | Field study with 33 homes | Orange County, California | 16% | Control group | Used postal mailings advising of irrigation schedules |
Qualls et al. (2001) | Journal | SMS | Field study with 23 test sites (21 residential, two public) | Boulder, Colorado | 73% | Theoretical requirements | Minimal operations and maintenance costs over the 3- to 5-year period |
Aquacraft (2003) | Report | ET | 3-year field study with nine homes during drought conditions | Colorado | 21% | Theoretical requirements | Average of $190 water bill savings |
Saving Water Partnership (2003) | Report | ET and RS | Field study with 106 homes during drought conditions (53% of historical average) | Puget Sound area, Washington | N/A | Study coalition included 24 water utilities | |
() | |||||||
Berg and Diamond (2004) | Journal | ET | 18-month study | Irvine, California | 10% | Control group | Larger landscape = better savings; 49% reduction in stormwater runoff |
Koeller (2004) | Report | ET | Review report | California | N/A | N/A | Summary of ET controller water savings and potential to reduce runoff |
MWDOC&IRWD (2004) | Report | ET | 18-month field study with 112 homes and 26 nonresidential controllers, 417 in control group, 225 in education group | Irvine, California | Residential 10%; Nonresidential 16% | Control group | Targeted education programs; 50%–71% reduction in runoff |
Pittenger et al. (2004) | Report | ET | University plots in sandy loam soil | Riverside, California | Variable | Timer | Setup of WBIC is most important factor in performance |
Cárdenas-Lailhacar et al. (2005) | Conference | SMS | University plots in fine sand | Gainesville, Florida | Average 72% | Control group | SMS increased efficiency; SMS without RS used 45% more water |
Hoyenga and Reaves (2006) | Journal | N/A | Overview of SWAT and need for partnerships between utilities and builders | Eugene, Oregon | N/A | N/A | Example of peak demand reduction to delay capital project |
Davis et al. (2007) | Conference | ET | 20 university plots in fine sand | Florida | Controller 1 & 2 = 11% − 40%; Controller 3 = −63% | Timer | Comparison study of 3 different controllers |
Pathan et al. (2007) | Journal | SMS | Six university plots in sandy soil | Perth, Australia | 25% | Timer | Water savings with little loss to turfgrass health; decreased risk of nitrogen leaching |
Shedd et al. (2007) | Conference | ET, RS, and SMS | 72 university plots in sand and fine sand | Florida | ; ; | Timer | Two dry seasons during test period ( rainfall) |
Cárdenas-Lailhacar and Dukes (2008) | Journal | RS | University plots during rainy period (62% days had rainfall) | Gainesville, Florida | 3%–44% | Timer | Test to determine effectiveness of rain sensors; payback period less than a year |
Cárdenas-Lailhacar et al. (2008) | Journal | RS, SMS | 72 university plots in fine sand | Gainesville, Florida | ; | Timer | SMS-based treatments more efficient than timer |
Devitt et al. (2008) | Journal | ET | 18-month field study with 27 homes | Las Vegas | 20% | Control group | 17 sites with ET controllers and 10 sites with non-ET controllers |
Grabow et al. (2008) | Conference | ET and SMS | 20-week university plot study in sandy loam during warmer and dryer than normal conditions | Raleigh, North Carolina | 11% | Timer | Important to use representative weather station |
USBR (2008) | Report | ET and SMS | Review report | Various | Various | Various | Summary of research and studies related to smart irrigation controllers |
Davis et al. (2009) | Journal | ET | 15-month study in 20 university plots in sandy soil | Florida | 43% | Timer | ET controller twice as effective compared to RS alone |
Mayer et al. (2009) | Report | WBIC | 3,112 smart controllers across more than 20 water agencies | California | 6% | Weather-normalized change in irrigation volume | High users not targeted; not all reductions statistically significant; 41.8% increased water use in first year; decrease of 16.4% in Year 3 versus. preinstall year |
McCready et al. (2009) | Journal | ET, RS, and SMS | 72 university plots in sandy and fine sand during drought conditions | Citra, Florida | ; ; | Timer | Four treatment periods with three relatively dry periods |
Shober et al. (2009) | Journal | ET | 16 university plots in fine sand | Wimauma, Florida | Variable | Timer | No negative effect on plant health; ET controllers did not result in savings over the reduced time treatment |
Cárdenas-Lailhacar and Dukes (2010) | Journal | SMS | University plots in sandy soil during warm season | Gainesville, Florida | 40%–88% | Timer | Savings were percentage of scheduled irrigation cycles bypassed |
Cárdenas-Lailhacar et al. (2010) | Journal | RS, SMS | 64 university plots in fine sand during drought conditions | Gainesville, Florida | ; | Timer | SMS saves water and maintains turf quality |
Davis and Dukes (2010) | Journal | ET | 20 university plots in fine sand | Wimauma, Florida | 42% (previous research) | Timer | Proper ET controller programming is important; potential to be more efficient than manual irrigation |
Kennedy/Jenks (2010) | Report | ET | Field study with 1,222 homes | Orange County, California | ; residential | Control group | Eight controller brands tested; excessive users not targeted; runoff reduction was significant; smart timers installed by homeowners performed better than those installed professionally |
Mayer and DeOreo (2010) | Journal | ET | 3,112 smart controllers in residential and commercial landscapes | California | 6% | Weather-normalized change in irrigation volume | Savings include sites with both decreased and increased use |
Mutchek and Williams (2010) | Journal | ET and SMS | Carbon footprint analysis of smart controllers | N/A | N/A | N/A | A need to lower controller prices and increase water savings to make controllers more widely used in the Southwest |
Swanson and Fipps (2010) | Report | ET and SMS | 2-year study with virtual landscapes using six controllers in 2008 and 10 controllers in 2009 | College Station, Texas | N/A | N/A | Improvement in controller performance and reduction in excessive irrigation |
Dukes and Davis (2011) | Report | ET | University test plots | Gainesville, Florida | N/A | N/A | Ability of the controller to handle rainfall has the most influence over SWAT scores |
McCready and Dukes (2011) | Journal | ET, RS, and SMS | 72 university test plots | Citra, Florida | N/A | N/A | Evaluation of only one 30-day SWAT test period may not completely capture controller performance |
Al-Ajlouni et al. (2012) | Journal | ET | University plots in clay loam during cool season | Las Cruces, New Mexico | to 259% of control | Timer | Control set to 80% of ET; two of five ET controllers saved significant water, two increased irrigation, and one had no change |
Cárdenas-Lailhacar and Dukes (2012) | Journal | SMS | 56 university plots in Gainesville, 36 university plots in Citra, 58 homes in Pinellas; sandy soils during warm season | Citra, Gainesville, and Pinellas, Florida | 42%–72% | Timer | Savings reported for conditions where turf quality remained good; field application rates appear to be lower than plot experiments |
Davis and Dukes (2012) | Journal | ET | Three field studies in southwestern and central Florida; 36 homes in southwestern Florida study | Florida | 63% | Timer | Users already irrigating less than well-watered conditions and accepting declines in landscape quality will not benefit |
Dukes (2012) | Journal | ET and SMS | Review paper | Various | 10%–70% | Various | Excellent summary of pilot- and plot-scale scientific studies |
Haley and Dukes (2012) | Journal | RS and SMS | 26-month field study with 58 homes in sandy soil | Palm Harbor, Florida | 65% | Nonintervention residential landscapes | SMS controllers showed significant irrigation savings |
Rutland and Dukes (2012) | Journal | RS and ET | 14 months, 20 university plots in sand during warm season | Wimauma, Florida | 25%–41% | Timer | Adding RS increased water savings |
Grabow et al. (2013) | Journal | ET and SMS | 3-year study of 40 university plots during cool season | Raleigh, North Carolina | ; | Timer | ET excessive watering due to overestimation of reference ET |
Davis and Dukes (2014) | Journal | ET | 2-year field study with 36 homes | Apollo Beach, Riverview, and Valrico, Florida | 23%–41% | Timer | Users already irrigating less than well-watered conditions and accept declines in landscape quality will not benefit |
Dobbs et al. (2014) | Journal | ET and SMS | 16 university plots in gravelly loam during warm season | Homestead, Florida | ; ; | Timer | Leaching coincided with rainfall events and excessive irrigation |
Melody et al. (2014) | Report | ET, RS, and SMS | Various | Various | Various | Various | Summary of research on smart irrigation controllers |
Williams et al. (2014) | Report | ET, RS, and SMS | Meta-analysis of water savings of 42 data points | Various | ; ; | Various | Eliminated duplicative and secondary results along with studies of high-water users |
Aquacraft (2015) | Report | ET | Evaluation of 1,365 smart controllers | Santa Clarita Valley, California | Not uniform | Control group | Programs should target excessive irrigators; water use increased among underirrigators and reduced among overirrigators; homeowner versus contractor installed had same results |
Davis and Dukes (2015b) | Journal | ET | Two independent field studies; 36 Hillsborough and 139 Orange County homes | Hillsborough and Orange Counties, Florida | Reduction | N/A | Programs should target excessive irrigators to increase water conservation potential |
Davis and Dukes (2015a) | Journal | ET and SMS | 22-month field study with 167 homes in sandy soil | Orange County, Florida | 21%–52% | Nonintervention residential landscapes | SMS controllers perform better under test conditions; proper programming important; additional savings from education and detailed programming |
Mayer et al. (2015) | Journal | N/A | N/A | N/A | N/A | N/A | Outdoor water savings are achievable and can be significant; outdoor conservation programs and measures are challenging, cost savings are rarely documented, and standardization of methods and measures are needed |
Migliaccio et al. (2015) | Journal | ET | Smartphone app developed and tested in university plot during warm season | Homestead, Florida | 42%–57% | Timer | Smartphone app was comparable to ET controllers; limitation is that app is not directly connected to controller |
Nautiyal et al. (2015) | Journal | ET and SMS | 20-week field study with 24 homes | Wake County, North Carolina | ; | Standard irrigation controller with no intervention | Variable rainfall and weather made it difficult to discern changes in behavior due to smart technology; smart controller cannot overcome poor irrigation system design, installation, and maintenance |
Boyer et al. (2016) | Journal | SMS | Survey of 3,000 commercial customers | Oklahoma City | N/A | N/A | At current water rates, commercial users unlikely to install smart controllers |
Cárdenasand Dukes (2016a) | Journal | SMS | 64 university plots in fine sand during warm season | Gainesville, Florida | ; | Timer | All water savings were achieved without a decline in the landscape quality |
Cárdenasand Dukes (2016b) | Journal | SMS | 32-month field study with 64 homes using reclaimed water | Palm Harbor, Florida | 44% | Nonintervention residential landscapes | SMS treatment effective at conserving water |
Davis and Dukes (2016) | Journal | ET and SMS | 31-month study, three independent studies; smart controller study; two virtual tests | Gainesville, Florida | Timer | Virtual testing with controllers not physically connected to irrigation systems and unable to fully account for rainfall | |
DeOreo et al. (2016) | Report | N/A | 4,643 customer survey responses; 2 weeks of potable meter usage from 762 customers | Arizona, California, Colorado, Connecticut, Georgia, North Carolina, Oregon, Pennsylvania, Florida, New Mexico, Nevada, Illinois, Texas, Washington, Alberta, and Ontario | N/A | N/A | In-ground irrigation systems were present in 53% of respondents, with smart controllers in 1%–8%; approximately 20% of homes overirrigated; outdoor irrigation accounted for the largest peak flows |
Mayer (2016) | Journal | N/A | 4,643 customer survey responses; 2 weeks of potable meter usage from 762 customers | Arizona, Californi, Colorado, Connecticut, Georgia, North Carolina, Oregon, Pennsylvania, Florida, New Mexico, Nevada, Illinois, Texas, Washington, Alberta, and Ontario | N/A | N/A | Irrigation use highly variable; follows consistent pattern with majority of homes applying at or below the theoretical irrigation requirement; small group of overirrigators accounted for bulk of excess irrigation |
Tess (2016) | Report | ET and RS | 12-month field study at three city test locations | Urbana, Illinois | Inconclusive | Timer | Inconclusive because high precipitation precluded need to irrigate for most of the study period |
Torbert et al. (2016) | Report | ET and SMS | Field study of 167 homes and four commercial properties in sandy soils | Orange County, Florida | (non-edu. and edu.); (commercial); (non-edu. and edu.) | Control group | Educational groups irrigate less due to optimized controller settings; post survey, majority satisfied; greater dissatisfaction with SMS versus ET |
Chabon et al. (2017) | Journal | SMS | 2-year field study in silty clay during cool season | Manhattan, Kansas | 31%–70% | Timer | Larger savings in a wetter year |
Kleinman (2017) | Conference | ET | Field study with 30 homes | Delaware, Ohio | 12% | Control group | Peak day demand study found 43%–45% peak reduction |
Mayer and Smith (2017) | Report | ET | Field study with 15 homes | New Jersey | N/A | N/A | Pilot-scale peak shifting experiment with remote interruption on two separate dates; estimated total of () peak demand reduction |
Morera et al. (2017) | Journal | ET and SMS | Survey of 167 customers, with 143 responding (86% response rate) | Florida | N/A | N/A | Controller satisfaction tied to satisfaction with appearance of landscape and perceived water-saving effectiveness |
Suh et al. (2017) | Journal | ET and SMS | Survey responses of 2,641 homeowners (88% response rate) | California, Florida, Texas | N/A | N/A | Study on perception and knowledge correlated with smart controller purchases; perceptions of conservation, restrictions, neighbors’ habits, and sociodemographic variables influenced decisions to purchase |
Vick et al. (2017) | Journal | ET and SMS | 3-year field study with 36 homes | Catawba-Wateree River basin, North Carolina and South Carolina | ET increase; ; | Timer | Savings largely negative, but SMS provided savings compared to baseline; water savings in highly controlled settings may not achieve equal benefits in residential applications |
Mayer et al. (2018) | Journal | ET | Field study with 15 homes | New Jersey | N/A | N/A | Peak shifting experiment with remote interruption on two separate dates; estimated total of () peak demand reduction |
USBR (2018) | Report | ET and SMS | Review report | N/A | N/A | N/A | Smart controller overview and product features |
Paxton et al. (2019) | Report | ET | 1,400 free controllers installed | Spanish Fork, Utah | 17% | Control group | Effective in peak demand reduction; update: 3,500 controllers installed through summer 2022 |
Zhang and Khachatryan (2019) | Journal | N/A | N/A | California, Florida, and Texas | N/A | N/A | Survey (); technology features focusing on the ease of use, reliability, and failure notifications are important and could increase adoption of controllers |
Cárdenas et al. (2020) | Journal | SMS, ET | 2-year university plots in fine sand | Gainesville, Florida | ; ; | Timer | Compared ET and SMS control to smartphone app; to encourage adoption, need to highlight water-saving features |
Conger and Dukes (2020) | Journal | WBIC | Virtual tests and 16 university plots | Citra, Florida (virtual), Balm, Florida (test plots) | 43% average based on previous findings | N/A | Summary of SWAT and EPA WaterSense programs; water savings for high users but increased irrigation for underirrigators; unable to recreate the SWAT test using just the protocol documentation |
Dukes (2020) | Journal | ET and SMS | Review paper | Various | Plot studies ; residential studies | Various | Studies have expanded to multiple climates and various soil and plant types; 17 identified studies in the past decade, five reported negative savings, typically due to ET controllers improperly set up or maintained |
Khachatryan et al. (2020) | Journal | N/A | Survey () assessing consumer perceptions of smart irrigation technologies | California, Florida, Texas | N/A | N/A | Results indicate consumers value smart irrigation technology |
Khachatryan et al. (2019) | Journal | N/A | Survey () assessing consumer perceptions of smart irrigation technologies | California, Florida, and Teaxas | N/A | N/A | Findings suggest that sensor-based controllers are preferred over the alternatives |
Serena et al. (2020) | Journal | ET and SMS | 2-year university plots in sandy loam | Las Cruces, New Mexico | 29%–45% | Timer | Demonstrated that smart controllers can be effectively used in arid environments in both cool and warm seasons without negatively affecting landscape quality |
Swanson and Fipps (2020) | Conference | ET | Virtual landscape over three seasons (summer, fall, winter) in various soil types | Texas | N/A | N/A | Nine controllers evaluated; inconsistent performance; each controller showed water savings compared to a standard timer; controller performance was better during summer |
Cárdenas et al. (2021) | Journal | SMS, ET | 5-year field study with 167 homes in sandy and flatwoods soils | Orange County, Florida | 17% to 31% (ET); 18% to 42% (SMS) | — | Review of past studies; SMS more efficient; demonstrated long-term ability of SMS and ET controllers to be effective |
Haghverdi et al. (2021a) | Journal | ET | 3 years, 36 university plots in fine sandy loam soil | Parlier, California | 39% to 103% of theoretical ET | N/A | 75% theoretical ET irrigation application could maintain hybrid bermudagrass quality; deficit irrigation could sustain quality for shorter durations before falling below minimum acceptable threshold |
Haghverdi et al. (2021b) | Journal | ET | 2 years, 36 university plots in fine sandy loam soil | Parlier, California | N/A | N/A | Limiting watering to specific days each week (common strategy of California drought response) did not provide substantial water savings |
USEPA (2021) | Report | SMS | N/A | N/A | N/A | N/A | Potential SMS performance based on system being properly designed, installed, and maintained; controller being properly programmed; and user monitoring water use and landscape healthier for periodic adjustments; nationally, potential for 1.77 trillion L (390 billion gal.) of water savings |
Note: SWAT = smart water application technologies; and edu. = educational.
Data Availability Statement
All data, models, and code generated or used during the study appear in the published article.
References
Abbott, B. W., et al. 2023. Emergency measures needed to rescue Great Salt Lake from ongoing collapse. Provo, UT: Brigham Young Univ.
Abrahamsen, F. E., Y. Ai, and M. Cheffena. 2021. “Communication technologies for smart grid: A comprehensive survey.” Sensors 21 (23): 8087. https://doi.org/10.3390/s21238087.
Al-Ajlouni, M. G., D. M. Vanleeuwen, and R. St Hilaire. 2012. “Performance of weather-based residential irrigation controllers in a desert environment.” J. Am. Water Works Assoc. 104 (12): 608–621. https://doi.org/10.5942/jawwa.2012.104.0155.
Allen, R. 1997. Demonstration of potential for residential water savings using a soil moisture controlled irrigation monitor: Project completion report. Provo, UT: US Bureau of Reclamation.
Allen, R. G., I. A. Walter, R. L. Elliott, T. A. Howell, D. Itenfisu, M. E. Jensen, and R. L. Snyder. 2005. The ASCE standardized reference evapotranspiration equation. Reston, VA: ASCE.
Aquacraft. 2003. Report on performance of ET based irrigation controller. Boulder, CO: Aquacraft.
Aquacraft. 2015. Evaluation of water savings from weather based irrigation controllers in Santa Clarita Valley. Boulder, CO: Aquacraft.
Arunkumar, M., and N. Mariappan. 2011. “Water demand analysis of municipal water supply using EPANET software.” Int. J. Appl. Bioeng. 5 (1): 9–19. https://doi.org/10.18000/ijabeg.10072.
Augustin, B., and G. Snyder. 1984. “Moisture sensor-controlled irrigation for maintaining bermudagrass turf.” Agron. J. 76 (5): 848–850. https://doi.org/10.2134/agronj1984.00021962007600050030x.
Bahri, A. 2012. “Integrated urban water management.” TEC Background Papers 16 (Jun): 1–89.
Berg, J., and R. Diamond. 2004. “Reducing urban runoff with smart ET controllers: Report review.” J. Am. Water Works Assoc. 96 (10): 44–45. https://doi.org/10.1002/j.1551-8833.2004.tb10720.x.
Boyer, M. J., M. D. Dukes, L. J. Young, and S. Wang. 2014. “Irrigation conservation of Florida-friendly landscaping based on water billing data.” J. Irrig. Drain. Eng. 140 (12): 04014037. https://doi.org/10.1061/(ASCE)IR.1943-4774.0000774.
Boyer, T. A., D. H. W. Jayasekera, and J. Q. Moss. 2016. “An assessment of Oklahoma City commercial businesses’ willingness to adopt irrigation water conservation methods.” Horttechnology 26 (6): 793–802. https://doi.org/10.21273/HORTTECH03433-16.
Cárdenas, B., and M. D. Dukes. 2016a. “Soil moisture sensor irrigation controllers and reclaimed water; Part I: Field-plot study.” Appl. Eng. Agric. 32 (2): 217–224. https://doi.org/10.13031/aea.32.11196.
Cárdenas, B., and M. D. Dukes. 2016b. “Soil moisture sensor irrigation controllers and reclaimed water; Part II: Residential evaluation.” Appl. Eng. Agric. 32 (2): 225–234. https://doi.org/10.13031/aea.32.11197.
Cárdenas, B., M. D. Dukes, E. Breder, and J. W. Torbert. 2021. “Long-term performance of smart irrigation controllers on single-family homes with excess irrigation.” AWWA Water Sci. 3 (2): e1218. https://doi.org/10.1002/aws2.1218.
Cárdenas, B., K. W. Migliaccio, M. D. Dukes, I. Hahus, and J. K. Kruse. 2020. “Irrigation savings from smart irrigation technologies and a smartphone app on turfgrass.” Trans. ASABE 63 (6): 1697–1709. https://doi.org/10.13031/trans.13903.
Cárdenas-Lailhacar, B., and M. D. Dukes. 2008. “Expanding disk rain sensor performance and potential irrigation water savings.” J. Irrig. Drain. Eng. 134 (1): 67–73. https://doi.org/10.1061/(ASCE)0733-9437(2008)134:1(67).
Cárdenas-Lailhacar, B., and M. D. Dukes. 2010. “Precision of soil moisture sensor irrigation controllers under field conditions.” Agric. Water Manage. 97 (5): 666–672. https://doi.org/10.1016/j.agwat.2009.12.009.
Cárdenas-Lailhacar, B., and M. D. Dukes. 2012. “Soil moisture sensor landscape irrigation controllers: A review of multi-study results and future implications.” Trans. ASABE 55 (2): 581–590. https://doi.org/10.13031/2013.41392.
Cárdenas-Lailhacar, B., M. D. Dukes, and G. L. Miller. 2005. Sensor-based control of irrigation in bermudagrass. St. Joseph, MI: American Society of Agricultural and Biological Engineers.
Cárdenas-Lailhacar, B., M. D. Dukes, and G. L. Miller. 2008. “Sensor-based automation of irrigation on bermudagrass, during wet weather conditions.” J. Irrig. Drain. Eng. 134 (2): 120–128. https://doi.org/10.1061/(ASCE)0733-9437(2008)134:2(120).
Cárdenas-Lailhacar, B., M. D. Dukes, and G. L. Miller. 2010. “Sensor-based automation of irrigation on bermudagrass during dry weather conditions.” J. Irrig. Drain. Eng. 136 (3): 184–193. https://doi.org/10.1061/(ASCE)IR.1943-4774.0000153.
Chabon, J., D. J. Bremer, J. D. Fry, and C. Lavis. 2017. “Effects of soil moisture–based irrigation controllers, mowing height, and trinexapac-ethyl on tall fescue irrigation amounts and mowing requirements.” Int. Turfgrass Soc. Res. J. 13 (1): 755–760. https://doi.org/10.2134/itsrj2016.04.0242.
Chini, C. M., L. E. Excell, and A. S. Stillwell. 2021. “A review of energy-for-water data in energy-water nexus publications.” Environ. Res. Lett. 15 (12): 123011. https://doi.org/10.1088/1748-9326/abcc2a.
Chini, C. M., and A. S. Stillwell. 2018. “The state of U.S. urban water: Data and the energy-water nexus.” Water Resour. Res. 54 (3): 1796–1811. https://doi.org/10.1002/2017WR022265.
Conger, S. L. D., and M. D. Dukes. 2020. “Evaluation of testing procedures for weather-based irrigation controllers.” Trans. ASABE 63 (5): 1277–1287. https://doi.org/10.13031/trans.13926.
Cooley, H., and P. Gleick. 2009. “Urban water-use efficiencies: Lessons from United States cities.” In The world’s water 2008–2009: The biennial report on freshwater resources, 101–126. Washington, DC: Island Press.
Coomes, P. A., B. D. Kornstein, T. D. Rockaway, and J. A. Rivard. 2010. North America residential water usage trends since 1992. Denver: Water Research Foundation.
Davis, S., and M. D. Dukes. 2012. “Landscape irrigation with evapotranspiration controllers in a humid climate.” Trans. ASABE 55 (2): 571–580. https://doi.org/10.13031/2013.41390.
Davis, S., M. D. Dukes, S. Vyapari, and G. L. Miller. 2007. “Evaluation and demonstration of evapotranspiration-based irrigation controllers.” In Proc., World Environment Water Resources Congress 2007: Restoring Our Natural Habitat, 1–18. Reston, VA: ASCE. https://doi.org/10.1061/40927(243)237.
Davis, S. L., and M. D. Dukes. 2010. “Irrigation scheduling performance by evapotranspiration-based controllers.” Agric. Water Manage. 98 (1): 19–28. https://doi.org/10.1016/j.agwat.2010.07.006.
Davis, S. L., and M. D. Dukes. 2014. “Irrigation of residential landscapes using the Toro Intelli-Sense controller in Southwest Florida.” J. Irrig. Drain. Eng. 140 (3): 04013020. https://doi.org/10.1061/(ASCE)IR.1943-4774.0000694.
Davis, S. L., and M. D. Dukes. 2015a. “Implementing smart controllers on single-family homes with excessive irrigation.” J. Irrig. Drain. Eng. 141 (12): 04015024. https://doi.org/10.1061/(ASCE)IR.1943-4774.0000920.
Davis, S. L., and M. D. Dukes. 2015b. “Methodologies for successful implementation of smart irrigation controllers.” J. Irrig. Drain. Eng. 141 (3): 04014055. https://doi.org/10.1061/(ASCE)IR.1943-4774.0000804.
Davis, S. L., and M. D. Dukes. 2016. “Importance of ET controller program settings on water conservation potential.” Appl. Eng. Agric. 32 (2): 251–262. https://doi.org/10.13031/aea.32.11182.
Davis, S. L., M. D. Dukes, and G. L. Miller. 2009. “Landscape irrigation by evapotranspiration-based irrigation controllers under dry conditions in Southwest Florida.” Agric. Water Manage. 96 (12): 1828–1836. https://doi.org/10.1016/j.agwat.2009.08.005.
de Oliveira Vieira, E., S. Sandoval-Solis, V. de Albuquerque Pedrosa, and J. P. Ortiz-Partida. 2020. Integrated water resource management. Cham, Switzerland: Springer.
DeOreo, W., P. Mayer, B. Dziegielewski, and J. Kiefer. 2016. Residential end uses of water, Version 2. Denver: Water Research Foundation.
DeOreo, W. B., P. W. Lander, R. J. Qualls, and J. M. Scott. 1997. Soil moisture sensors: Are they a neglected tool. Boulder, CO: Aquacraft.
Devitt, D. A., K. Carstensen, and R. L. Morris. 2008. “Residential water savings associated with satellite-based ET irrigation controllers.” J. Irrig. Drain. Eng. 134 (1): 74–82. https://doi.org/10.1061/(ASCE)0733-9437(2008)134:1(74).
Dieter, C., M. Maupin, R. Caldwell, M. Harris, T. Ivahnenko, J. Lovelace, N. Barber, and K. Linsey. 2018. Estimated use of water in the United States in 2015. US Geological Survey Circular 1441. Reston, VA: USGS.
Dobbs, N. A., K. W. Migliaccio, Y. Li, M. D. Dukes, and K. T. Morgan. 2014. “Evaluating irrigation applied and nitrogen leached using different smart irrigation technologies on bahiagrass (Paspalum notatum).” Irrig. Sci. 32 (3): 193–203. https://doi.org/10.1007/s00271-013-0421-1.
Doorn, N. 2021. “Artificial intelligence in the water domain: Opportunities for responsible use.” Sci. Total Environ. 755 (Feb): 142561. https://doi.org/10.1016/j.scitotenv.2020.142561.
Dukes, M. D. 2012. “Water conservation potential of landscape irrigation smart controllers.” Trans. ASABE 55 (2): 563–569. https://doi.org/10.13031/2013.41391.
Dukes, M. D. 2020. “Two decades of smart irrigation controllers in us landscape irrigation.” Trans. ASABE 63 (5): 1593–1601. https://doi.org/10.13031/trans.13930.
Dukes, M. D., and S. L. Davis. 2011. Examination of SWAT protocol utilizing a performance analysis of weather-based irrigation controllers: Update with extended data. Lexington, MA: Eastern Research Group.
Gellings, C. W. 2017. “Evolving practice of demand-side management.” J. Mod. Power Syst. Clean Eng. 5 (1): 1–9. https://doi.org/10.1007/s40565-016-0252-1.
Global Water Partnership. 2022. “About IWRM.” Accessed May 25, 2022. https://www.gwp.org/en/gwp-SAS/ABOUT-GWP-SAS/WHY/About-IWRM.
Grabow, G. L., I. E. Ghali, R. L. Huffman, G. L. Miller, D. Bowman, and A. Vasanth. 2013. “Water application efficiency and adequacy of ET-based and soil moisture–based irrigation controllers for turfgrass irrigation.” J. Irrig. Drain. Eng. 139 (2): 113–123. https://doi.org/10.1061/(ASCE)IR.1943-4774.0000528.
Grabow, G. L., A. Vasanth, D. Bowman, R. L. Huffman, and G. L. Miller. 2008. “Evaluation of evapotranspiration-based and soil-moisture-based irrigation control in turf.” In Proc., World Environment Water Resources Congress 2008, 1–9. Reston, VA: ASCE. https://doi.org/10.1061/40976(316)117.
Griffiths-Sattenspiel, B., and W. Wilson. 2009. The carbon footprint of water. Portland, OR: River Network.
Hadjimichael, A., J. Comas, and L. Corominas. 2016. “Do machine learning methods used in data mining enhance the potential of decision support systems? A review for the urban water sector.” AI Commun. 29 (6): 747–756. https://doi.org/10.3233/AIC-160714.
Haghverdi, A., M. Reiter, A. Singh, and A. Sapkota. 2021a. “Developing irrigation water conservation strategies for hybrid bermudagrass using an evapotranspiration-based smart irrigation controller in inland Southern California.” Agric. Water Manage. 245 (Feb): 106586. https://doi.org/10.1016/j.agwat.2020.106586.
Haghverdi, A., A. Singh, A. Sapkota, M. Reiter, and S. Ghodsi. 2021b. “Hybrid bermudagrass and tall fescue turfgrass irrigation in Central California: I. assessment of visual quality, soil moisture and performance of an ET-based smart controller.” Agron. J. 11 (8): 1666. https://doi.org/10.3390/agronomy11081666.
Haley, M. B., and M. D. Dukes. 2012. “Validation of landscape irrigation reduction with soil moisture sensor irrigation controllers.” J. Irrig. Drain. Eng. 138 (2): 135–144. https://doi.org/10.1061/(ASCE)IR.1943-4774.0000391.
Haley, M. B., M. D. Dukes, and G. L. Miller. 2007. “Residential irrigation water use in Central Florida.” J. Irrig. Drain. Eng. 133 (5): 427–434. https://doi.org/10.1061/(ASCE)0733-9437(2007)133:5(427).
Hedden-Nicely, D. R. 2022. “Climate change and the future of western US water governance.” Nat. Clim. Change 12 (2): 108–110. https://doi.org/10.1038/s41558-021-01141-3.
Hoyenga, J., and R. Reaves. 2006. “Smart application technology: A water utility/irrigation industry partnership.” J. Am. Water Works Assoc. 98 (2): 112–121. https://doi.org/10.1002/j.1551-8833.2006.tb07593.x.
Hunt, T., D. Lessick, J. Berg, J. Wiedmann, T. Ash, D. Pagano, M. Marian, and A. Bamezai. 2001. Residential weather-based irrigation scheduling: Evidence from the Irvine ET controller study. Irvine, CA: Irvine Ranch Water District.
Jones, S. C., and R. B. Sowby. 2014. “Water system optimization: Aligning energy efficiency, system performance, and water quality.” J. Am. Water Works Assoc. 106 (6): 66–71. https://doi.org/10.5942/jawwa.2014.106.0087.
Judge, M. A., A. Khan, A. Manzoor, and H. A. Khattak. 2022. “Overview of smart grid implementation: Frameworks, impact, performance and challenges.” J. Energy Storage. 49 (May): 104056. https://doi.org/10.1016/j.est.2022.104056.
Kennedy/Jenks. 2010. Pilot implementation of smart timers: Water conservation, urban runoff reduction, and water quality. Fountain Valley, CA: Municipal Water District of Orange County.
Khachatryan, H., A. Rihn, D. H. Suh, and M. D. Dukes. 2020. Homeowners’ preferences for smart irrigation systems and features. Gainesville, FL: Univ. of Florida.
Khachatryan, H., D. H. Suh, W. Xu, P. Useche, and M. D. Dukes. 2019. “Towards sustainable water management: Preferences and willingness to pay for smart landscape irrigation technologies.” Land Use Policy 85 (Jun): 33–41. https://doi.org/10.1016/j.landusepol.2019.03.014.
Khan, R., and H. Gilani. 2021. “Global drought monitoring with drought severity index (DSI) using Google Earth engine.” Theor. Appl. Climatol. 146 (1): 411–427. https://doi.org/10.1007/s00704-021-03715-9.
Khatami, R., M. Parvania, and A. Bagherinezhad. 2018. “Continuous-time model predictive control for real-time flexibility scheduling of plugin electric vehicles.” IFAC-PapersOnLine 51 (28): 498–503. https://doi.org/10.1016/j.ifacol.2018.11.752.
Kleinman, J. 2017. “A smart approach to smart controllers: Plan, do, check, act.” In Proc., Fall Conf. Orlando, FL: Florida American Water Works Association.
Koeller, J. 2004. Evaluation of potential best management practices—Residential and small commercial weather-based irrigation controllers. Sacramento, CA: California Urban Water Conservation Council.
Mayer, P. 2016. “Water research foundation study documents water conservation potential and more efficiency in households.” J. Am. Water Works Assoc. 108 (10): 31–40. https://doi.org/10.5942/jawwa.2016.108.0160.
Mayer, P., W. B. DeOreo, M. Hayden, R. Davis, E. Caldwell, T. Miller, and P. J. Bickel. 2009. Evaluation of California weather-based ’smart’ irrigation controller programs. Sacramento, CA: California Dept. of Water Resources.
Mayer, P., W. B. DeOreo, E. M. Opitz, J. C. Kiefer, W. Y. Davis, B. Dziegielewski, and J. O. Nelson. 1999. Residential end uses of water. Denver: American Water Works Association Research Foundation.
Mayer, P., M. Hunter, and R. Smith. 2018. “Peak day water demand management study heralds innovation, connection, cooperation.” J. Am. Water Works Assoc. 110 (5): 42–48. https://doi.org/10.1002/awwa.1078.
Mayer, P., P. Lander, and D. Glenn. 2015. “Outdoor water use: Abundant savings, scarce research.” J. Am. Water Works Assoc. 107 (2): 61–66. https://doi.org/10.5942/jawwa.2015.107.0029.
Mayer, P., and R. Smith. 2017. Peak day water demand management study. Chicago: Alliance for Water Efficiency.
Mayer, P. W., and W. B. DeOreo. 2010. “Improving urban irrigation efficiency by capitalizing on the conservation potential of weather-based ‘smart’ controllers.” J. Am. Water Works Assoc. 102 (2): 86–97. https://doi.org/10.1002/j.1551-8833.2010.tb10054.x.
McCready, M. S., and M. D. Dukes. 2011. “Landscape irrigation scheduling efficiency and adequacy by various control technologies.” Agric. Water Manage. 98 (4): 697–704. https://doi.org/10.1016/j.agwat.2010.11.007.
McCready, M. S., M. D. Dukes, and G. L. Miller. 2009. “Water conservation potential of smart irrigation controllers on St. Augustinegrass.” Agric. Water Manage. 96 (11): 1623–1632. https://doi.org/10.1016/j.agwat.2009.06.007.
Melody, M., H. Stratton, A. Williams, and C. Dunham. 2014. Resources for national water savings for outdoor water use. Berkeley, CA: Ernest Orlando Lawrence Berkeley National Laboratory. https://doi.org/10.2172/1172642.
Migliaccio, K. W., K. T. Morgan, C. Fraisse, G. Vellidis, and J. H. Andreis. 2015. “Performance evaluation of urban turf irrigation smartphone app.” Comput. Electron. Agric. 118 (Oct): 136–142. https://doi.org/10.1016/j.compag.2015.08.015.
Morera, M. C., P. F. Monaghan, M. D. Dukes, and E. Breder. 2017. “Predicting satisfaction with smart irrigation controllers and their long-term use among homeowners in Central Florida.” J. Am. Water Resour. Assoc. 53 (4): 929–943. https://doi.org/10.1111/1752-1688.12542.
Mutchek, M. A., and E. D. Williams. 2010. “Design space characterization for meeting cost and carbon reduction goals.” J. Ind. Ecol. 14 (5): 727–739. https://doi.org/10.1111/j.1530-9290.2010.00282.x.
MWDOC&IRWD (Municipal Water District of Orange County and Irvine Ranch Water District). 2004. The residential runoff reduction study. Fountain Valley, CA: MWDOC&IRWD.
Nautiyal, M., G. L. Grabow, R. L. Huffman, G. L. Miller, and D. Bowman. 2015. “Residential irrigation water use in the Central Piedmont of North Carolina. II: Evaluation of smart irrigation technologies.” J. Irrig. Drain. Eng. 141 (4): 04014062. https://doi.org/10.1061/(ASCE)IR.1943-4774.0000820.
Panda, S., S. Mohanty, P. K. Rout, B. K. Sahu, M. Bajaj, H. M. Zawbaa, and S. Kamel. 2022. “Residential demand side management model, optimization and future perspective: A review.” Energy Rep. 8 (Jun): 377–3766. https://doi.org/10.1016/j.egyr.2022.02.300.
Pathan, S. M., L. Barton, and T. D. Colmer. 2007. “Evaluation of a soil moisture sensor to reduce water and nutrient leaching in turfgrass (Cynodon dactylon cv. Wintergreen).” Aust. J. Exp. Agric. 47 (2): 215–222. https://doi.org/10.1071/EA05189.
Paxton, J., A. Ayaviri McGraw, W. Robison, and G. P. Williams. 2019. Spanish Fork irrigation-water conservation study. Spanish Fork, UT: Spanish Fork City Public Works.
Pittenger, D. R., D. A. Shaw, and W. E. Richie. 2004. Evaluation of weather-sensing landscape irrigation controllers. Sacramento, CA: Office of Water Use Efficiency California Department of Water Resources.
Qualls, R. J., J. M. Scott, and W. B. DeOreo. 2001. “Soil moisture sensors for urban landscape irrigation: Effectiveness and reliability.” J. Am. Water Resour. Assoc. 37 (3): 547–559. https://doi.org/10.1111/j.1752-1688.2001.tb05492.x.
Rapp, A. H., A. M. Capener, and R. B. Sowby. 2023. “Adoption of artificial intelligence in drinking water operations: A survey of progress in the United States.” J. Water Resour. Plann. Manage. 149 (7): 06023002. https://doi.org/10.1061/JWRMD5.WRENG-5870.
Robbins, J. 2022. A quiet revolution: Southwest cities learn to thrive amid drought. New Haven, CT: Yale School of the Environment.
Romero, C. C., and M. D. Dukes. 2016. “A method to estimate residential irrigation from potable meter data.” Appl. Eng. Agric. 32 (2): 245–250. https://doi.org/10.13031/aea.32.11194.
Rosenberg, D. E., and D. W. Watkins Jr. 2018. “New policy to specify availability of data, models, and code.” J. Water Resour. Plan. Manage. 144 (9): 01618001. https://doi.org/10.1061/(ASCE)WR.1943-5452.0000998.
Rutland, D. C., and M. D. Dukes. 2012. “Performance of rain delay features on signal-based evapotranspiration irrigation controllers.” J. Irrig. Drain. Eng. 138 (11): 978–983. https://doi.org/10.1061/(ASCE)IR.1943-4774.0000499.
Saving Water Partnership. 2003. Water efficient irrigation study. Seattle: Seattle Public Utilities.
Serena, M., C. Velasco-Cruz, J. Friell, M. Schiavon, E. Sevostianova, L. Beck, R. Sallenave, and B. Leinauer. 2020. “Irrigation scheduling technologies reduce water use and maintain turfgrass quality.” Agron. J. 112 (5): 3456–3469. https://doi.org/10.1002/agj2.20246.
Shedd, M., M. D. Dukes, and G. L. Miller. 2007. “Evaluation of evapotranspiration and soil moisture-based irrigation control on turfgrass.” In Proc., World Environment Water Resources Congress 2007, 1–21. Reston, VA: ASCE. https://doi.org/doi:10.1061/40927(243)238.
Shober, A. L., S. Davis, M. D. Dukes, G. C. Denny, S. P. Brown, and S. Vyapari. 2009. “Performance of Florida landscape plants when irrigated by ET-based controllers and time-based methods.” J. Environ. Hortic. 27 (4): 251–256. https://doi.org/10.24266/0738-2898-27.4.251.
Shurtz, K. M., E. Dicataldo, R. B. Sowby, and G. P. Williams. 2022. “Insights into efficient irrigation of urban landscapes: Analysis using remote sensing, parcel data, water use, and tiered rates.” Sustainability 14 (3): 1427. https://doi.org/10.3390/su14031427.
Sowby, R. B. 2022. “Like a storm in the desert: John Denver and the American West’s changing hydrology.” J. Sustain. Water Built Environ. 8 (3): 02522002. https://doi.org/10.1061/JSWBAY.0000972.
Sowby, R. B., and S. J. Burian. 2017. “Survey of energy requirements for public water supply in the United States.” J. Am. Water Works Assoc. 109 (7): 320–330. https://doi.org/10.5942/jawwa.2017.109.0080.
Sowby, R. B., and E. Dicataldo. 2022. “The energy footprint of US irrigation: A first estimate from open data.” Energy Nexus 6 (Aug): 100066. https://doi.org/10.1016/j.nexus.2022.100066.
Sowby, R. B., and N. S. Grigg. 2022. “More papers, more authors, more references: What does it mean for water resources planning and management?” J. Water Resour. Plan. Manage. 148 (3): 02522001. https://doi.org/10.1061/(ASCE)WR.1943-5452.0001525.
Suh, D. H., H. Khachatryan, A. Rihn, and M. D. Dukes. 2017. “Relating knowledge and perceptions of sustainable water management to preferences for smart irrigation technology.” Sustainability 9 (4): 607. https://doi.org/10.3390/su9040607.
Swanson, C., and G. Fipps. 2010. Initial evaluation of smart irrigation controllers: Year two (2009) results. College Station, TX: Rio Grande Basin Initiative.
Swanson, C. L., and G. Fipps. 2020. “Evolution of smart irrigation controllers: A 15 year Texas perspective.” In Proc., 6th Decennial National Irrigation Symp., 6–8. St. Joseph, MI: American Society of Agricultural and Biological Engineers. https://doi.org/10.13031/irrig.2020-051.
Tess, S. R. 2016. Urbana irrigation controls study. Champaign, IL: Illinois Sustainable Technology Center.
Torbert, J. W., S. L. Davis, and M. D. Dukes. 2016. Smart irrigation controller demonstration and evaluation in Orange County, Florida. Denver: Water Research Foundation.
USBR (US Bureau of Reclamation). 2008. Summary of smart controller water savings studies. literature review of water savings studies for weather and soil moisture based landscape irrigation control devices. Temecula, CA: USBR Southern California Area Office in the Lower Colorado Region.
USBR (US Bureau of Reclamation). 2018. Weather and soil moisture based landscape irrigation scheduling devices. 6th ed. Temecula, CA: USBR Southern California Area Office in the Lower Colorado Region.
USEPA. 2013. Outdoor water use in the United States. Washington DC: USEPA.
USEPA. 2021. “WaterSense specification for soil moisture-based irrigation controllers supporting statement.” Accessed May 25, 2022. https://www.epa.gov/sites/default/files/2021-02/documents/ws_sms_final_spec_supporting_statement.pdf.
USEPA. 2022a. “How we use water.” Accessed May 25, 2022. https://www.epa.gov/watersense/how-we-use-water.
USEPA. 2022b. “WaterSense product search, irrigation controllers.” Accessed May 25, 2022. https://lookforwatersense.epa.gov/products/Product-Search-Results-IrrigationController.html.
USEPA. 2022c. “Weather-based irrigation controllers.” Accessed May 25, 2022. https://www.epa.gov/watersense/weather-based-irrigation-controllers.
Vick, R. L., G. L. Grabow, G. L. Miller, and R. L. Huffman. 2017. “Water conservation potential of smart irrigation technologies in the Catawba-Wateree River Basin.” J. Irrig. Drain. Eng. 143 (2): 04016079. https://doi.org/10.1061/(ASCE)IR.1943-4774.0001130.
Waskom, R., and M. Neibauer. 2014. Water conservation in and around the home. Fort Collins, CO: Colorado State Univ. Extension.
Wheeler, K. G., B. Udall, J. Wang, E. Kuhn, H. Salehabadi, and J. C. Schmidt. 2022. “What will it take to stabilize the Colorado River?” Science 377 (6604): 373–375. https://doi.org/10.1126/science.abo4452.
Williams, A., H. Fuchs, and C. D. Whitehead. 2014. Estimates of savings achievable from irrigation controller. Berkeley, CA: Lawrence Berkeley National Laboratory.
Williams, A. P., B. I. Cook, and J. E. Smerdon. 2022. “Rapid intensification of the emerging southwestern North American megadrought in 2020–2021.” Nat. Clim. Change 12 (3): 232–234. https://doi.org/10.1038/s41558-022-01290-z.
Zhang, X. M., and H. Khachatryan. 2019. “Investigating homeowners’ preferences for smart irrigation technology features.” Water. 11 (10): 1996. https://doi.org/10.3390/w11101996.
Zib, L., D. M. Byrne, L. T. Marston, and C. M. Chini. 2021. “Operational carbon footprint of the U.S. water and wastewater sector’s energy consumption.” J. Cleaner Prod. 321 (Oct): 128815. https://doi.org/10.1016/j.jclepro.2021.128815.
Information & Authors
Information
Published In
Copyright
This work is made available under the terms of the Creative Commons Attribution 4.0 International license, https://creativecommons.org/licenses/by/4.0/.
History
Published online: Oct 31, 2023
Published in print: Jan 1, 2024
Discussion open until: Mar 31, 2024
ASCE Technical Topics:
- Engineering fundamentals
- Information management
- Infrastructure
- Integrated systems
- Irrigation
- Irrigation engineering
- Irrigation systems
- Residential location
- Systems engineering
- Systems management
- Urban and regional development
- Urban areas
- Water and water resources
- Water conservation
- Water management
- Water policy
- Water shortage
- Water supply
- Water supply systems
Authors
Metrics & Citations
Metrics
Citations
Download citation
If you have the appropriate software installed, you can download article citation data to the citation manager of your choice. Simply select your manager software from the list below and click Download.