Review of Climate Change Adaptation Strategies in Water Management
Publication: Journal of Hydrologic Engineering
Volume 29, Issue 1
Abstract
Climate change (CC) is considered one of the most critical threats to human lives and activities due to dramatic increases in the frequency and severity of droughts and floods under global warming. To alleviate such impacts, many studies on CC adaptation strategies in water management have emerged. This review covers 131 relevant studies published over the past two decades. It aims to robustly synthesize the applied strategies/techniques and identify findings and gaps. In addition, a bibliometric analysis is performed to describe the co-citation network and statistical characteristics of the reviewed papers and identify the related research clusters. A typical procedure for CC adaptation studies is proposed based on previous studies. It is found that systems reoperation was preferred for CC adaptation in water resources management, specifically by updating the reservoir operation curves using optimization algorithms. However, low impact development (LID) measures were favored in storm drainage and flood mitigation systems. As for future relevant research, the main recommendations are integrating environmental, social, and economic aspects in evaluating CC adaptation strategies and incorporating land use and land cover (LULC) and water demand changes in CC adaptation studies. This state-of-the-art review represents essential information for improving CC adaptation strategies in water management.
Introduction
The Intergovernmental Panel on Climate Change (IPCC) defines climate change (CC) as “change in the state of the climate which can be identified by changes in the mean and variability of its properties which persist for an extended period, typically decades or longer” (IPCC 2007; Khaniya et al. 2021). CC is described by temperature increase and global warming (Shao et al. 2021). It is triggered by many factors, such as human-induced increases in aerosols, land-use change, and mainly greenhouse gas (GHG) emissions (Shrestha et al. 2014; Olabanji et al. 2020). CC impacts are noticed in many sectors, i.e., agriculture, coasts, ecosystems, energy, forests, human health, society, transportation, and water resources (EPA 2016).
CC dramatically impacts the water sector, as reported in many studies (e.g., Khaniya et al. 2021; Barría et al. 2021; Onyutha et al. 2021; Papadimitriou et al. 2019; Anh et al. 2019; Zhang 2015; Rehana and Mujumdar 2013). It may increase the temperature and raise evapotranspiration rates, exacerbating water demand (Azhoni et al. 2018; Onyutha et al. 2021). It may also cause fluctuations in the precipitation patterns, particularly the precipitation intensity (e.g., Onyutha et al. 2021; Shrestha et al. 2014; Schuster et al. 2012; Barría et al. 2021; Olabanji et al. 2020) and the periods between rainfall events (e.g., Garnier and Holman 2019; Feng and Wu 2016; Giuntoli et al. 2015). An increased precipitation intensity may increase flood severity, threatening the safety of human lives and properties (Yang and Liu 2020), while long dry periods and reduced precipitation amounts may result in droughts, endangering the security of water and food (Garnier and Holman 2019; Azhoni et al. 2018; Singh et al. 2016; Jaswal et al. 2015; Upgupta et al. 2015).
Chang et al. (1992) reviewed many studies on CC impacts on water resources and categorized the impacts into two groups. The first group represents CC impacts on water management variables (e.g., water supply infrastructure and drainage systems). For example, impacts on (1) drainage systems of coastal cities due to rises in seawater level (e.g., Titus et al. 1987), (2) urban sewerage systems due to increases in precipitation (Chang et al. 1992), and (3) reservoirs performances due to fluctuations in climatic variables (e.g., Callaway and Currie 1985). The second group comprises CC impacts on water-use variables (e.g., water quality and instream and offstream water use). Fluctuations in hydroelectric power production (e.g., Singh 1987) and water quality degradation (Chang et al. 1992) are examples of CC impacts on water-use variables. There are also many other reviews on CC impacts on streamflow, floods, droughts (e.g., Kahaduwa and Rajapakse 2022; Singh et al. 2014), reservoir operations, water demand, hydropower generation, ecological flow (e.g., Kahaduwa and Rajapakse 2022), snowmelt and glacier melt, and groundwater (e.g., Singh et al. 2014).
To mitigate CC impacts on water resources, proactive, urgent, and sustainable adaptation actions are required (Füssel 2007; Simonet and Fatorić 2016). CC adaptation is defined as “the process of adjustment to actual or expected climate and its effects” (IPCC 2014). Depending on its objectives, it can be classified into two major types: (1) reducing GHG emissions; and (2) adapting systems to the expected future CC (referred to as CC adaptation strategies in this study), which is the focus of the current review. There are many reviews (e.g., Khaniya et al. 2021; Garnier and Holman 2019; Papadaskalopoulou et al. 2015; Saraswat et al. 2016; Pandey et al. 2003) related to CC adaptation strategies in water management that do not cover relevant modeling techniques. These reviews are on specific regions or topics, such as ecosystem-based solutions in Sri Lanka (Khaniya et al. 2021), drinking water resources in Europe (Garnier and Holman 2019), improving the adaptive capacity of the water sector in Cyprus (Papadaskalopoulou et al. 2015), practices and governance in stormwater management (Saraswat et al. 2016), and rainwater harvesting (Pandey et al. 2003).
A few previous reviews covered CC adaptation modeling techniques but are limited to urban drainage networks (Kourtis and Tsihrintzis 2021) or nature-based strategies for urban flood extremes (Quagliolo et al. 2022). Therefore, there is a need for a comprehensive review of modeling techniques to identify the suitable and commonly used models for each strategy, their functions, capabilities, advantages, disadvantages, and recommended improvements. Such information is vital for planning and designing CC adaptation systems and measuring their efficiency and performance, given that climatic and hydrologic models are significant sources of uncertainty (Kundzewicz et al. 2018).
The objectives of this study are as follows: (1) comprehensively reviewing CC adaptation strategies in water management, including relevant modeling techniques; (2) drawing, from the reviewed papers, a general procedure (i.e., framework) that can assist in the planning and design of CC adaptation strategies in water management; (3) providing a list of relevant research gaps; and (4) performing a nonextensive bibliometric analysis to provide essential statistics on the reviewed documents and their connections (Ellegaard and Wallin 2015). The bibliometric analysis has been applied in many studies (e.g., Islam et al. 2021; Nasture et al. 2022; Chen et al. 2022) but not in CC adaptation strategies in water management.
The following are questions that this review will answer: (1) “What are the types of CC adaptation strategies in water management?”; (2) “What are the commonly used adaptation strategies?”; (3) “How were these strategies modeled?”; and (4) “What are the gaps within the reviewed studies that can be further investigated?” The current review is limited to peer-reviewed journal/conference publications in English, available on the Web of Science database (see details in the “Methodology” section). It is worth mentioning that this review does not include other document types, such as technical research reports/monographs from government/industry practitioners and standards published by institutions. These limitations bound all the identified research gaps and drawn conclusions.
Methodology
The methodology of this review (Fig. 1) consists of four main parts: selection of relevant publications, synthesis and discussion, bibliometric analysis, and identified research gaps. The publications selection passed through three steps: (1) identification, (2) screening, and (3) eligibility (Shaffril et al. 2021). This procedure was followed in different studies, for example, Heslehurst et al. (2018) in health and medicine and Kourtis and Tsihrintzis (2021) in engineering. The Web of Science, a widely used database, was used as a search engine. Three sets of keyword combinations (listed in Fig. 1) were used to search in the topics for publications until November 20, 2022. The search resulted in 253 documents (Fig. 1), which were screened to exclude the missing, non-English, and duplicated ones. Then, the articles’ eligibility was checked, based on the title and abstract or the body, to consider only the relevant ones (i.e., articles that discussed or modeled CC adaptation strategies in water management). Therefore, only 131 documents were found suitable and considered for this review (listed in the Supplemental Materials). These documents include 106 peer-reviewed journal articles, 14 peer-reviewed literature reviews, 6 proceeding papers, 4 book chapters, and 1 editorial material.
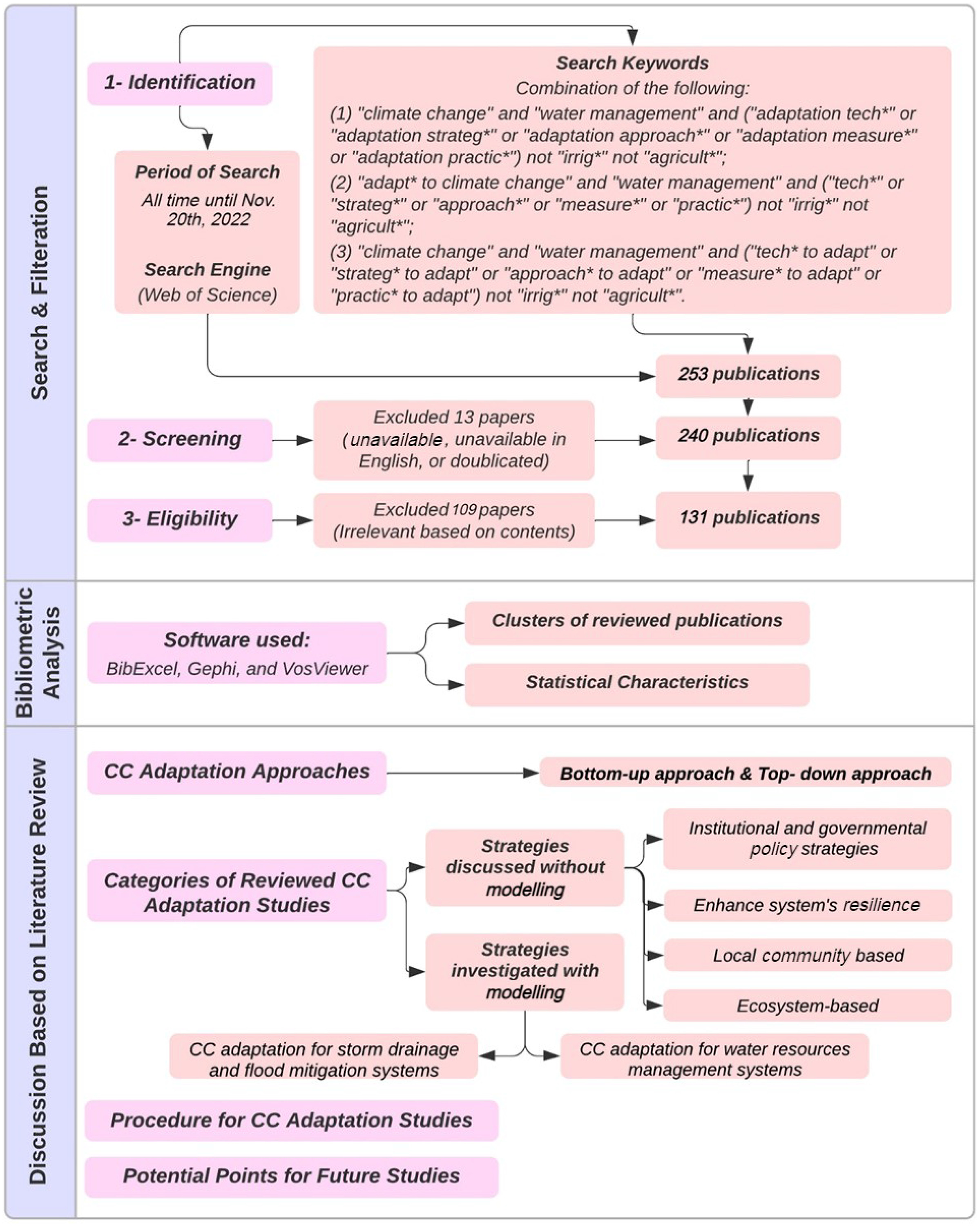
The selected documents were reviewed to identify their main contributions, gaps, and the general procedure for investigating CC adaptation strategies in water management. A bibliometric analysis was then performed using BibExcel, Gephi, and VosViewer software packages (i.e., each was used for a specific step; further details are provided in the “Bibliometric Analysis” section). BibExcel was chosen for its capability of importing and handling data from many databases, including the Web of Science. Gephi and VOSviewer were selected for their capabilities of dealing with big network data and providing visualization alternatives, enhanced filtering, built-in network analysis, and clustering (Islam et al. 2021). In this review, the bibliometric analysis was mainly performed to identify clusters of the reviewed publications based on their co-citation network.
Synthesis and Discussion
This section categorizes and discusses different approaches and strategies of CC adaptation and their applications. It also presents a general procedure for studying CC adaptation developed based on the methodologies used in the reviewed papers.
Top-Down and Bottom-Up CC Adaptation Approaches
The two main approaches for CC adaptation in water management are top-down and bottom-up (Ludwig et al. 2014). The top-down approach starts by studying the CC impact on climatic drivers (e.g., precipitation and temperature) and its reflection on the study basin’s hydrology. Then, different CC adaptation strategies are applied. This approach incorporates various GHG emission scenarios, general circulation models (GCMs) with different downscaling techniques, and hydrologic models’ uncertainties (Ludwig et al. 2014). On the contrary, the bottom-up approach focuses on increasing the resilience of the water management systems to improve their adaptive capacities and reduce their vulnerability to future negative impacts (Ludwig et al. 2014).
Categories of CC Adaptation Strategies
The CC adaptation strategies discussed in the reviewed documents are identified and classified as follows.
CC Adaptation Strategies without Modeling
This section contains the CC adaptation strategies that were discussed but not modeled in the reviewed documents. These strategies are categorized into the following four subcategories:
Institutional and Governmental Policies as an Adaptation to CC
Studies under this category discuss necessary policies, regulations, and agreements, especially for transboundary basins, that foster CC adaptation in water management. For example, Jafroudi (2020) studied policies and agreements for equitable water use within transboundary water basins under CC. Some studies investigated and recommended adapting policies to CC through enhanced coordination within transboundary water systems (e.g., Timmerman et al. 2017) and shared basins (e.g., Mgquba and Majozi 2020). Khacheba et al. (2018) recommended shifting policy approaches from a sectoral focus to an integrated approach with policy coherence among the sectors facing CC challenges. Quandt et al. (2022) reviewed policies adopted in three case studies from different regions to solve water security issues under CC. They concluded that “there is no one-size fits all policy solution to water scarcity.” Balaz et al. (2021) reviewed the Delphi and analytical hierarchy process (AHP) methods for ranking policy options for CC adaptation.
Resilience as a CC Adaptation Approach
Some of the reviewed articles studied CC adaptation strategies to improve the resilience of cities against urban flood risk (e.g., Shao et al. 2021; Fu et al. 2022; Rodrigues and Antunes 2021). Shao et al. (2021) examined different techniques (e.g., smart water utilities, shared scheduling of several water sources, and coupling water system management with flood control measures using artificial intelligence) to adapt Shenzhen City, Guangdong, China, to CC. Fu et al. (2022) reviewed bottom-up community-based techniques, including “resilience mapping, property-based resistant and resilient measures, flood insurance, and social resilience building,” in 50 cities in China. Rodrigues and Antunes (2021) investigated the usage of best management practices (BMPs; e.g., porous pavements, rainwater harvesting systems, and bioretention) to transition a coastal city in the south of Portugal into a water-sensitive city. They identified flood-prone areas and developed strengths, weaknesses, opportunities, and threats (SWOT) analysis, which helped select and allocate BMPs to increase the city’s resilience.
Local Community-Based Adaptation Strategies
This category includes strategies applied on local scales (i.e., at community levels) using simple techniques. Rankoana (2020) reviewed strategies (e.g., limiting household withdrawal from connections and regulating water supply between specific hours per day) applied by communities in Limpopo province, South Africa, to face increasing droughts under CC. Lebel (2013) discussed local communities’ adaptation strategies (e.g., underground canals and connected storage ponds) used by farmers, residents, and islanders in societies relying on natural water resources.
Ecosystem-Based Adaptation Strategies
The ecosystem-based adaptation (EbA) measures are cost-effective and eco-friendly; therefore, they are preferred to gray infrastructure-based ones. Khaniya et al. (2021) discussed the advantages of applying EbA at the field level, watershed scale, and urban and coastal environments for CC adaptation. EbA measures applied at the field level include contour drains, contour walls, and home gardening (Khaniya et al. 2021). However, means used in the watershed scale include (1) reforestation or afforestation, which decreases GHG and alleviates flooding through increasing stormwater capture and infiltration; and (2) riparian buffer strips nearby the streams, ponds, or streams to control water quality and quantity, and reduce soil erosion (Khaniya et al. 2021). In urban environments, natural wetlands were favored as an efficient EbA strategy for enhancing stormwater quality, attenuating flood peaks, and enhancing groundwater recharge (Khaniya et al. 2021). In coastal systems, EbA strategies, such as mangroves, coastal marshes, dunes, and barrier reefs, play a key role in alleviating CC impact. They decrease structural destruction by absorbing waves’ energy and lessening storm surges impact on upland areas (Khaniya et al. 2021).
CC Adaptation Strategies with Modeling
This section reviews the articles on CC adaptation that incorporated modeling. Based on their objectives, the CC adaptation strategies are classified into water resources management strategies and storm drainage and flood mitigation ones. These categories, subcategories, strategies, corresponding models, and study areas are summarized in Table 1 and discussed below.
Category of strategies | Reference | Study area | Adaptation strategies | Climate model/GHG emission scenarios | Hydrologic, hydraulic, and water management models |
---|---|---|---|---|---|
For water resources management | |||||
Water supply augmentation; demand management; and reuse | Mirdashtvan et al. (2021) | Tehran province, Iran | Water demand management: declining population growth rate, decreasing domestic water distribution network’s losses, reducing domestic water consumption, and improving irrigation water efficiency and decreasing agriculture water consumption | GCMs/RCPs 2.6, 4.5, and 8.5 | WEAP model |
Olabanji et al. (2020) | Olifants River basin, South Africa | Construction of a new reservoir, water conservation and demand management strategy, and water reuse strategy | RCMs/RCP4 and RCP8.5 | WEAP model | |
Hernández-Hernández et al. (2020) | Alicante city, Spain | Rainwater harvesting using rainwater tanks | Delta change from AEMET model/2RCPs | Not mentioned | |
Momblanch et al. (2020) | River basin in Himalayas, India | Optimized irrigation schedule, afforestation of bare soil areas, construction of hydropower plants, and setting minimum flows downstream reservoirs | RCM bias corrected/RCPs 2.6, 4.5, 6, and 8.5 | WEAP model | |
Sampson et al. (2020) | Phoenix metropolitan area, Arizona, US | Supply management, demand management, and improving the water use efficiency | High and low flow from the historical record | WaterSim-6 | |
Papadimitriou et al. (2019) | Europe and five European subregions (Alpine, Northern, Atlantic, Continental, and Southern) | Reducing water use, and maximizing environmental allocation of water | RCMs/RCP2.6 | Integrated Assessment Platform 2 (IAP2) | |
Escriva-Bou et al. (2017) | Jucar River basin, Spain | Demand management: efficiency improvement of irrigation systems, and decreasing demand by agricultural policies | RCMs and statistical best fit/not mentioned | Hydrologic: lumped water management: SIMGES | |
System management actions: same priority to all irrigation areas or water markets based | |||||
Xiao-Jun et al. (2014) | Yulin city, China | Water demand management: water-saving measures (water price) | CC projections-National Climate Center of China (CNCCP 2007) | Multicriteria evaluation system | |
Water supply management: engineering projects such as dam building, pipelines, diversion systems, and distribution systems | |||||
Bonelli et al. (2014) | Maipo River basin, central Chile | Water rights purchase; reservoir storage capacity and operation, and improvements in water demand efficiency | GCMs/A1b and B1 | Hydrologic: WEAP | |
Baena-Ruiz et al. (2020) | Plana de Oropesa-Torreblanca aquifer, Castellon province, Spain | Demand management: land use changed from agricultural to residential | RCM/RCP8.5 | Auxiliary models and density-dependent flow (SEAWAT) | |
Supply augmentation: increasing the complementary resources, water reusing for golf courses irrigation, and using desalination plants water for human consumption in the new urban areas | |||||
Updating reservoirs control curves and reservoir management | Sant’Anna et al. (2022) | Lievre River basin in Quebec, Canada | Updating reservoirs operation rules for energy production, recreational and ecological use, and flood control | Ensemble of 828 GCMs/RCPs 2.6, 4.5, 6, and 8.5 | Simulation model: HEC-ResSim |
Optimization: stochastic dual dynamic programming (SDDP) algorithm | |||||
Sterle et al. (2020) | Truckee River basin, US | Updating the reservoir operation control curves for future changes in snowmelt | GCM from (Dettinger et al. 2016) | Hydrologic: PRMS | |
Reservoir operations: RiverWare | |||||
Stagge and Moglen (2017) | Washington, DC metropolitan area, US | Adopting supply system and reservoirs operation rules | 5 GCM and stochastically generated streamflow and precipitation | Hydrologic routing and reservoir operations: OASIS | |
Water supply: Stagge and Moglen (2014) model | |||||
Optimization algorithm: S-Metric Selection-Evolutionary Multiobjective Optimization Algorithm | |||||
(SMSEMOA) | |||||
Steinschneider and Brown (2012) | Westfield River basin, Springfield, MA, US | Best-guess strategy based on future CC | GCMs downscaled/A2 | Optimization algorithm | |
Dynamic strategy (dynamically manages for short-term climate variability) | Hydrologic: DHSVM | ||||
Koch et al. (2010) | Part of Elbe River basin, Czech Republic | Reservoir water management | GCM ECHAM5/A1B | Hydrologic: SWMM | |
Management: Interactive Simulating System | |||||
Water Balance: WBalMo | |||||
Medellin-Azuara et al. (2008) | California state, US | Updating operation rules of a complex optimized water management system (groundwater and surface water storage and water reallocation) | GCM downscaled/A2 | Hydrologic: no model | |
Optimization: CALVIN | |||||
Adapting water allocation | Rheinheimer et al. (2016) | Upper Yuba River basin, California, US | Redefining the WYT definition thresholds: adaptive with Historical WYT Distribution (AHD); and Adaptive with Historical WYT Distribution and Future hydrology (AHDF) | 4 GCMs/RCP8.5 from CMIP5 | WEAP |
Optimization: MILP | |||||
Yang et al. (2014) | Indus River basin, Pakistan | Adapting surface water allocation schemes: basin-wide economic allocation and Indus River System Authority (IRSA) water allocation | Historical data and delta change from literature | Indus Basin Model Revised-Multi-Year (IBMR–MY) hydro-agro-economic model | |
Null and Viers (2013) | California’s Sacramento Valley and San Joaquin Valley, US | Maintaining historical WYT thresholds with changing flow regimes for a new distribution of WYT | GCM Statistically downscaled/A2 and B1 | Hydrologic: variable infiltration capacity (VIC) | |
Maintaining the historical distribution of WYTs by modifying WYT thresholds for changing flow regimes | |||||
For storm drainage and flood mitigation | |||||
LID to reduce surface runoff | Binesh et al. (2019) | Tehran province, Iran | Best management practices (BMP): bioretention basins and porous pavement | GCM (MRI-CGCM3)/RCP4.5 | US EPA SWMM |
Kirshen et al. (2018) | Exeter, NH, US | LID measures: bioretention basins, infiltration basins, and porous pavement | 8 GCM models/RCPs 4.5 and 8.5 | Hydrologic: HSPF, HEC-HMS | |
Hydraulic: HEC-RAS | |||||
Hydrologic and hydraulic: SWMM | |||||
Chen et al. (2017) | Xingshi Village, Hukou Township, Hsinchu County, Taiwan | 12 LID measures including infiltration trench, infiltration basin, dry detention pond, wet detention pond, vegetated filter strip, grassed swale, constructed wetlands, sand filter, green roof, rain barrel, porous pavement, and bioretention | GCM - Richardson-type weather generator/ RCP 8.5 | Hydrologic: SWMM | |
Spatial planning: SA algorithm | |||||
Wang et al. (2017) | Outram Park, Singapore | LID measures | Percentage of increase based on (WB group, Singapore) | EPA SWMM | |
Urich and Rauch (2014) | Innsbruck city, Austria | On-site infiltration systems (infiltrating rainwater from roofs) | No model (applied CC factor) | SWMM | |
LID, conventional strategy or updating masterplans | Kourtis et al. (2021) | Small urban basin in Athens, Greece | Sewer enlargement (gray) and permeable surfaces (green) | RCMs bias corrected | Hydrologic and hydraulic: SWMM |
Manocha and Babovic (2018) | Kent Ridge catchment in campus of National University of Singapore | Gray: expansion of drainage canals | GCM/RCPs 4.5 and 8.5 | Hydrology: not mentioned | |
Sustainable: implementation of green roofs and porous pavements | Optimization: Matlab 2015R | ||||
Xie et al. (2022) | Dealim3 catchment in Seoul, South Korea | Optimal scheduling strategy generated by the Intelligent Scheduling Model (ISM) | 8 GCMs/2.6 and 8.5 | Hydrology: SWMM | |
Upgrading existing facility strategy (enlarging the detention pond) | Optimization: ISM | ||||
Simplified LID strategy (directly altering the impervious surface ratio) | |||||
Löwe et al. (2017) | Scotchman’s Creek catchment, Melbourne, Australia | Large-scale implementation of rainwater harvesting (RWH) facilities | No climate model/Three scenarios from the literature | Hydrodynamic: Mike Flood 1D-2D | |
Increasing the pipe’s capacity | Urban development: DAnCE4Water | ||||
Master plans that control the form of urban development and flood zoning |
Adaptation Strategies in Water Resources Management Systems
Strategies discussed in this section aim to reduce the future gap between water supply and demand, preserve the water resources allocation scheme, or optimize it to maximize net income benefit under CC. They are classified into three subcategories (Table 1): (1) water supply conservation, water demand management, and water reuse adaptation strategies; (2) updating reservoir control curves and reservoir management; and (3) adapting water allocation using different techniques.
In the first subcategory, Olabanji et al. (2020) modeled all three strategies (i.e., water supply conservation, water demand management, and water reuse) individually and combined in the South Alborz Region, Iran. They found that the combined option achieved the highest performance. In general, the techniques used for water supply conservation are constructing new dams to regulate streamflow (e.g., Olabanji et al. 2020; Xiao-Jun et al. 2014) and rainwater harvesting (e.g., Sampson et al. 2020; Hernández-Hernández et al. 2020). The techniques used for water demand management are canal/pipe leaks reduction (e.g., Olabanji et al. 2020; Sampson et al. 2020; Mirdashtvan et al. 2021) and water use efficiency enhancement through metering devices and billing (e.g., Olabanji et al. 2020; Xiao-Jun et al. 2014). These techniques also included water demand reduction through changing land-use plans (e.g., Sampson et al. 2020), revising agricultural policies (e.g., Escriva-Bou et al. 2017; Mirdashtvan et al. 2021), improving irrigation efficiency, and reducing population growth rate and domestic water consumption (e.g., Mirdashtvan et al. 2021). Mirdashtvan et al. (2021) found that reducing population growth rate and water distribution network losses can significantly affect water demand management. The techniques used to study the water reuse strategy included the reuse of treated wastewater for domestic purposes and revising nonpotable water policies (e.g., Olabanji et al. 2020; Sampson et al. 2020).
Reoperating water resources systems is preferred to constructing/rehabilitating infrastructure projects since reoperation eliminates or decreases the required costs (Sant’Anna et al. 2022). Therefore, updating reservoir control curves and operations (the second subcategory) was widely used for CC adaptation. This strategy achieved the required energy production, recreational and ecological use, and flood control in the Lievre River basin in Quebec, Canada (Sant’Anna et al. 2022). It was also used to alleviate the expected future changes in snowmelt under CC by coupling the Precipitation Runoff Modeling System (PRMS) with the RiverWare reservoir operations model (Sterle et al. 2020). In addition, it was used to sustain adequate river flow for navigation in the studied river while guaranteeing the drinking and industrial water demand (Koch et al. 2010). Steinschneider and Brown (2012) updated the reservoir control curves by investigating two strategies: (1) the best guess strategy, which optimizes operations for the mean projection of future climate as simulated by GCMs, and (2) the dynamic strategy, which dynamically manages the system for short-term climate variability using seasonal hydrologic forecasts.
The third subcategory comprises studies intended to cope with CC by preserving/optimizing water allocation under future conditions, e.g., updating the water year type (WYT) definition. WYTs are indices that classify similar water years (e.g., dry year and wet year) to indicate water availability, which helps with water management and planning. They are “typically defined by comparing forecasted or observed total annual runoff from a river basin against specified flow thresholds, with thresholds defined based on the distribution of historical runoff observations” (Rheinheimer et al. 2016). For CC adaptation, water managers may rely on the existing WYTs and only update the water allocation rules. Otherwise, they may prefer to keep the water allocation rules unchanged and update the current WYT classifications by modifying their thresholds. Rheinheimer et al. (2016) studied preserving the historical WYT distribution to satisfy environmental and hydropower objectives under CC using the Water Evaluation and Planning (WEAP) and mixed integer linear programming (MILP) models. They redefined the WYT thresholds considering two alternatives: adaptive with historical WYT distribution (AHD) and adaptive with historical WYT distribution and future hydrology (AHDF). Null and Viers (2013) used the variable infiltration capacity (VIC) hydrologic model to compare maintaining historical WYT thresholds while updating WYT distribution against maintaining the historical WYT distribution while updating WYT thresholds for changing flow regimes. Updating the WYT definitions is a nonstructural measure and has shown its significant role in CC adaptation in water resources management systems. However, further investigations are recommended (Rheinheimer et al. 2016).
Adaptation Strategies in Storm Drainage and Flood Mitigation Systems
Since CC increases flood severity (Yang and Liu 2020), adaptation strategies are required in flood mitigation and storm drainage systems. LIDs and BMPs (e.g., bioretention basins, porous pavements and infiltration basins) were commonly used strategies for reducing or attenuating runoff, as presented in Table 1. They played a prominent role in mitigating/reducing flooding, especially in urban drainage systems, according to many studies (e.g., Binesh et al. 2019; Kirshen et al. 2018; Wang et al. 2017; Chen et al. 2017). However, it is worth mentioning that LID measures could not prevent the streamflow changes caused by urbanization, as reported by other studies (e.g., Hopkins et al. 2020). Kourtis et al. (2021) studied sewer enlargement (a gray strategy), permeable surfaces (LID), and a combination of them. They concluded that the performance of these strategies is significantly sensitive to climate scenarios. Löwe et al. (2017) investigated reducing potential runoff by changing master plans and implementing multistory buildings to control imperviousness increase. They also investigated other effective CC adaptation measures such as flood zoning through a buyback of properties, LIDs (i.e., rainwater harvesting facilities), and stormwater pipes enlarging.
CC Adaptation Study Procedure
Based on the reviewed studies, we developed a general procedure depicted as a framework (Fig. 2). This framework describes the main stages and their sequence, dependencies, and modeled/assessed components required for a comprehensive CC adaptation study in water management. However, this procedure can be customized (e.g., by adding/removing components) to fit a study’s objectives. This section discusses the modeling techniques, uncertainties, and challenges associated with CC adaptation studies.
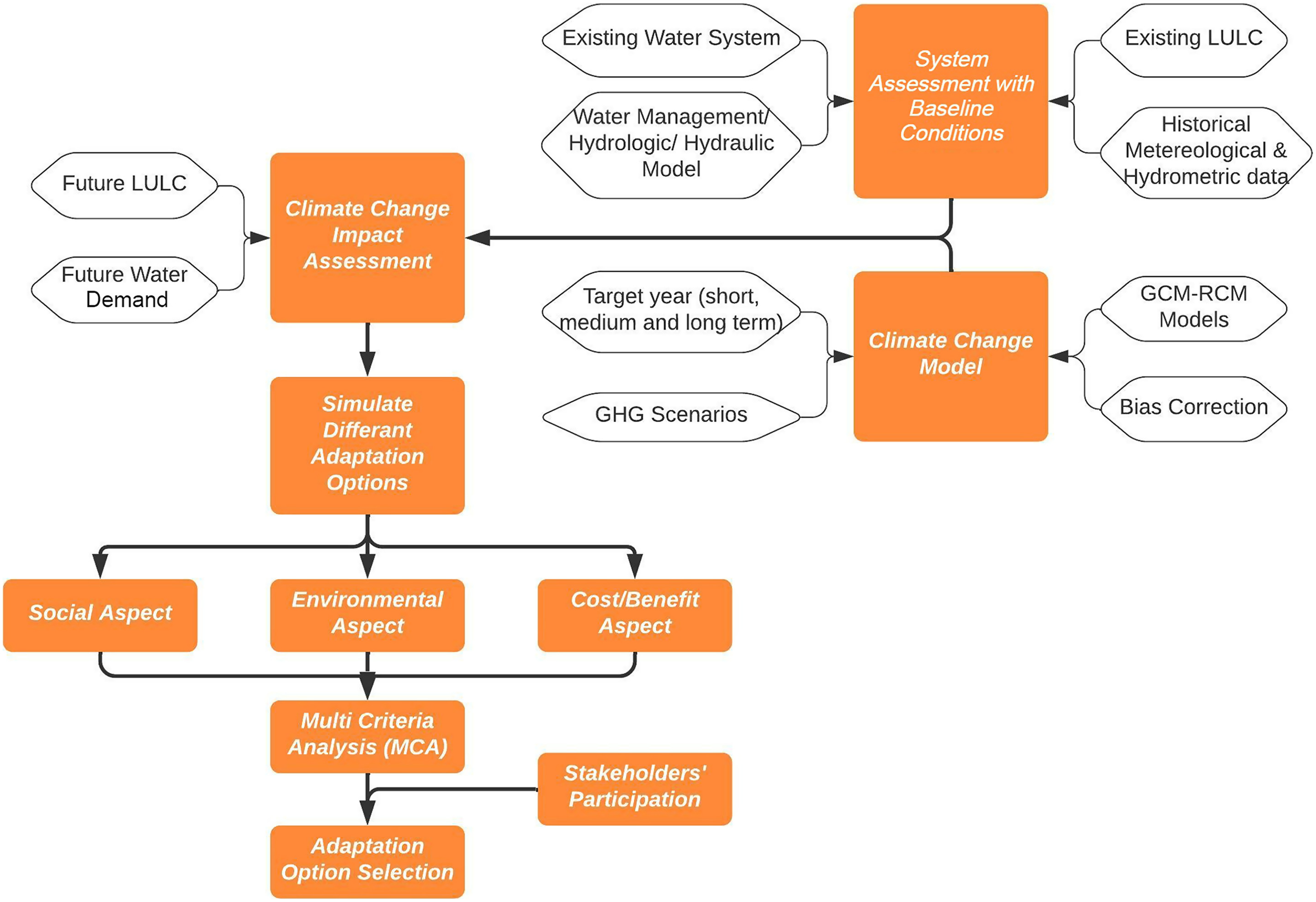
Hydrologic, Hydraulic, and Water Management Modeling
The model selection depends on the field in which CC adaptation strategies are studied. In the reviewed studies, the WEAP model developed by the Stockholm Environment Institute was the most commonly used (Table 1). It integrates hydrologic processes, water resources allocation, water management, water infrastructure, and water demand. However, it lacks an optimization routine to facilitate calibrating its hydrologic model, and it is time-consuming (Olabanji et al. 2020). These limitations should be investigated in future studies to improve the WEAP model’s performance. Water resources management models (Table 1) include similar components, such as water demand and allocation, hydrologic processes, and CC impact. They also have some common criteria; for example, their simulations incorporate long periods with a relatively large time step, i.e., a month (e.g., Mirdashtvan et al. 2021; Momblanch et al. 2020; Koch et al. 2010), a week (e.g., Rheinheimer et al. 2016; Sant’Anna et al. 2022), or a day (e.g., Stagge and Moglen 2017). In addition, they mostly comprise optimization algorithms coupled with the simulation model. For example, optimization algorithms were coupled with the Storm Water Management Model (SWMM) and Water Balance Model (WBalMo) (Koch et al. 2010), Operational Analysis and Simulation of Integrated Systems (OASIS) hydrologic routing and reservoir operations model and a water supply model (Stagge and Moglen 2017), the Distributed Hydrology Soil Vegetation Model (DHSVM) hydrologic model (Steinschneider and Brown 2012), and the Hydrologic Engineering Center-Reservoir System Simulation (HEC-ResSim) simulation model (Sant’Anna et al. 2022).
SWMM was the most commonly used model for storm drainage and flood mitigation (Table 1) in the reviewed studies (e.g., Binesh et al. 2019; Kirshen et al. 2018; Chen et al. 2017; Wang et al. 2017; Urich and Rauch 2014). It is used for hydrologic and hydraulic simulations and efficiently simulates the pipe network stormwater analysis for urban drainage systems. However, SWMM is a one-dimensional model and is limited when flood hazard and risk assessment and management are required. In such cases, 2D [e.g., Hydrologic Engineering Center-River Analysis System (HEC-RAS) 2D] and 1D-2D (e.g., InfoWorks ICM, an integrated catchment modeling software package) hydrodynamic models are recommended because of their capabilities to accurately simulate the water movement velocity and spread above the surface. However, their accuracy highly depends on the data availability, resolution, and accuracy. One of these models’ primary functions is simulating river flooding (e.g., Kirshen et al. 2018). They are used in conjunction with hydrologic models; for example, Kirshen et al. (2018) used Hydrologic Engineering Center-Hydrologic Modeling System (HEC-HMS) with HEC-RAS. Kourtis and Tsihrintzis (2021) summarized many hydrologic and hydraulic models used for urban drainage systems, e.g., Smart Growth Water Assessment Tool for Estimating Runoff (SG WATER), Mike Urban (software package for modeling urban water), MikeSHE (software package for integrating groundwater and surface water), Mike Flood (software package for modeling floods), XP-SWMM (storm water management model developed by XP Solutions), and the Model for Urban Sewers (MOUSE).
Models simulations for storm drainage systems can be continuous (e.g., Binesh et al. 2019) or event-based with short durations, typically ranging from 1 h to 1 day (e.g., Binesh et al. 2019; Chen et al. 2017; Wang et al. 2017). Optimization models were also used in modeling these systems to achieve the optimum adaptation options combinations (Manocha and Babovic 2018) and spatial planning (Chen et al. 2017) and reach the optimal scheduling for the drainage network’s elements, i.e., pumps and ponds (Xie et al. 2022).
Assessment and Performance Indices
For evaluating the CC adaptation strategies, different indices are used to assess the performance of water management systems before and after applying these strategies. For example, the adaptability index was used to measure the role of bioretention basins and porous pavement in enhancing the urban drainage system performance against flooding under CC (Binesh et al. 2019). Also, water shortage rate and water supply proportions indices were used to assess the role of different adaptation options in improving water resources security under CC (Xiao-Jun et al. 2014).
CC Modeling Techniques
GCMs are commonly used for CC modeling, employing a mathematical model of the general circulation of a planetary atmosphere or ocean (Marchuk et al. 1982). Since GHG emissions are the governing cause of global heat and climate change (Olabanji et al. 2020), the GCM results alter based on the GHG representative concentration pathways (RCPs). IPCC adopted four pathways, i.e., RCP2.6, RCP4.5, RCP6, and RCP8.5, corresponding to a range of potential radiative forcing values (i.e., 2.6, 4.5, 6, and , respectively) occurring by 2100. The possibility of each pathway occurrence depends on the expected future GHG emissions, where RCP2.6 is the most optimistic scenario with low GHG emissions, and RCP8.5 is the worst-case one with high GHG emissions. The choice of the RCP to be simulated within the CC impact study is a critical source of uncertainties. It is typically recommended to analyze different GHG scenarios to define the uncertainties and then engage stakeholders in the decisions. However, more scenarios require more analysis, modeling, and processing time. Schwalm et al. (2020) argued that “RCP8.5 is the best match out to mid-century under current and stated policies with still highly plausible levels of emissions in 2100.” Therefore, we suggest that future studies should consider RCP8.5 as an expected pathway and RCP6 as an optimistic one.
GCM modeling encompasses coarse spatial and temporal resolution. However, downscaling techniques improve the resolution (Kourtis and Tsihrintzis 2021). There are two main downscaling methods of the GCM: dynamic downscaling using regional climate models (RCMs) and statistical downscaling. RCMs improve the spatial resolution of the GCMs, while the statistical downscaling technique enhances the dynamic model’s results based on historical trends (Kourtis and Tsihrintzis 2021). Many statistical downscaling methods have been investigated, varying from simple interpolation to stochastic weather generators and neural networks. Statistical modeling is not only used for enhancing the resolution of climate models but also for the bias correction of RCMs (Kourtis and Tsihrintzis 2021). However, statistical downscaling is associated with uncertainties (Gutiérrez 2019). Climate models used in the reviewed studies and the corresponding simulated GHG emissions are presented in Table 1.
Incorporating the Impact of LULC and Socioeconomic Development Change
The land use and land cover (LULC) change and population growth, which are constantly changing, impact water management strategies. LULC change may increase imperviousness and, consequently, increase surface runoff, exacerbating flood risk under future conditions. Previous studies showed a disparity in the impact of LULC change on the drainage systems compared to the CC impact. Based on these studies, the LULC change can impact drainage systems more, equally, or less than CC (Kourtis and Tsihrintzis 2021). In water management, population growth and LULC change, which is mainly impacted by population growth, influence water demand. Within the reviewed modeled CC adaptation studies, 11% incorporated only the potential LULC change, 7% incorporated only the future water demand change, 29% combined both aspects (i.e., future LULC and water demand changes), and 53% included none of them [Fig. 3(a)].
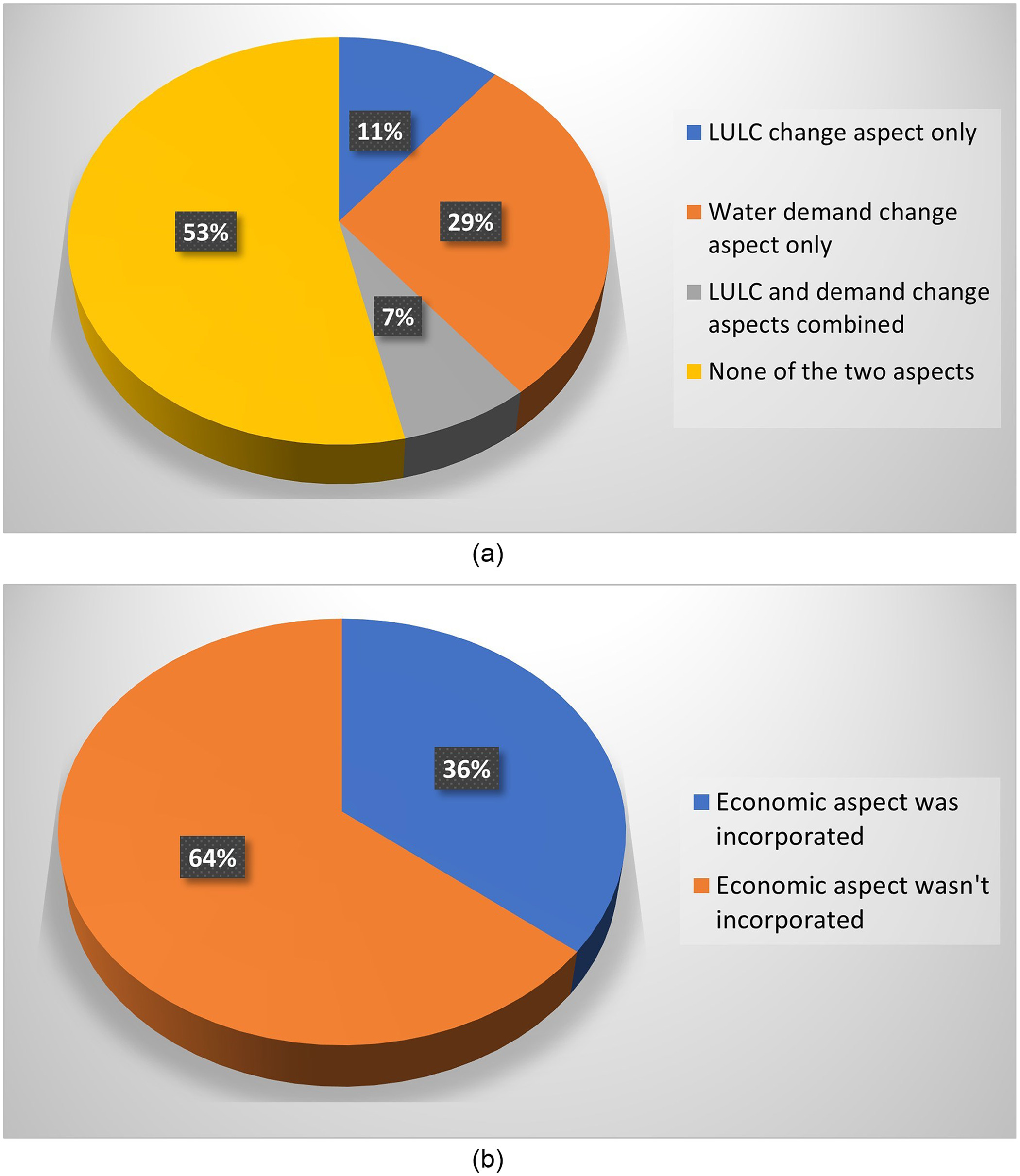
Selection of CC Adaptation Strategies
Before implementing CC adaptation strategies for a specific study area, the objectives and potential CC adaptation options should be identified. Then, these options should be evaluated using reliable aspects such as economic, environmental, and social aspects. The economic aspect, which is evaluated through cost-benefit analysis, is one of the main aspects used to compare the different options. The economic assessment is usually accompanied by many uncertainties, impacting decision-making (Hallegatte 2010). Despite its importance in evaluating the different CC adaptation options, the economic aspect was included in only about a third [Fig. 3(b)] of the reviewed articles (e.g., Olabanji et al. 2020; Manocha and Babovic 2018; Chen et al. 2017; Löwe et al. 2017; Escriva-Bou et al. 2017). And it was not included in most of the studies on CC nature-based solutions (Quagliolo et al. 2022). However, in recent studies, there is an increasing interest in incorporating the economic aspect into the evaluation, optimization, and selection phases of CC adaptation strategies.
The environmental aspect also can be used to evaluate the adaptation strategies, especially when considering gray structural strategies. It was incorporated in only a few of the reviewed articles, as also reported in Kourtis and Tsihrintzis’s (2021) study. It was monetized to compare pipe enlargement, LID measures, open urban drainage systems, and recreational basins as adaptation strategies (Zhou et al. 2013) and to optimize the reservoir operation curves (Medellín-Azuara et al. 2008). The social aspect is also used for evaluating CC adaptation options. It is assessed by measuring socioeconomic and demographic factors that affect the community’s capacity to cope with hazards (e.g., Kirshen et al. 2018).
Generally, comparing adaptation strategies is somewhat complicated. Therefore, it requires involving stakeholders and using multicriteria decision analysis (MCDA) to facilitate decision-making (Bojovic et al. 2018). For example, Bojovic et al. (2018) investigated the methodology of stakeholders’ e-participation in the MCDA of CC adaptation measures using effectiveness, efficiency, environmental performance, side effects, contribution to the resolution of conflicts, and performance under uncertainty as criteria. Where e-participation is a social activity facilitated by information and communication technologies (ICTs) that assures the interaction among citizens, public administration, and politicians, as critical stakeholders, in the decision-making process (Le Blanc 2020).
Bibliometric Analysis
Clusters Based on Bibliometric Analysis
BibExcel and Gephi software programs were used to cluster the data based on their co-citation network. Co-citation analysis is a science mapping technique that assumes articles frequently cited together in other documents investigate/discuss similar topics, which helps recognize a research area’s inner structure (Islam et al. 2021; Donthu et al. 2021). This technique was preferred for clustering the reviewed publications since it reveals the influential publications and uncovers the knowledge foundations. As a result, the clusters’ topics are identified based on influential publications (Donthu et al. 2021). Gephi created a co-citation network of nodes and edges (links), where the nodes represent the articles, and the links between the nodes represent the co-citation between the articles. It groups the nodes with the strongest co-citation connections within the same cluster. The clustering quality can be measured by the modularity index (Brandes et al. 2007; Islam et al. 2021). The index value is between –1 and 1, where a high index value means dense links between the nodes within clusters but weak links between the nodes in different clusters (Blondel et al. 2008). Gephi uses the Louvain algorithm, developed by Blondel et al. (2008), to maximize the clusters’ local modularity. Louvain randomly orders the nodes in the network, then removes and replaces each node in a different cluster until the modularity reaches its maximum (Blondel et al. 2008).
The co-citation analysis resulted in four clusters (see Fig. S1 ), where the number of publications in each cluster is illustrated in Table 2. To identify a cluster’s topic, we used the main keywords from three (randomly selected) articles with the highest Page Rank scores and considered the main contributions of the reviewed studies within the cluster. Page Rank is an algorithm developed by Brin and Page (1998) and used by Gephi to measure the importance of each node (i.e., publication). Giving a high importance score to publications based only on citation counting has many weaknesses (Ding et al. 2009), which have been lessened by the Page Rank scoring method (Islam et al. 2021). Instead of giving the same weight to all citations, the Page Rank algorithm gives higher importance scores to the articles cited by other highly cited ones (Islam et al. 2021).
Cluster topic | Papers count | Oldest/newest year | Publications with the highest Page Rank (Page Rank scores) | Keywords |
---|---|---|---|---|
1. CC organizational and institutional adaptation strategies to achieve capacity development, resilience, and sustainable water management | 54 | 2010/2022 | Azhoni et al. (2018) (0.025); Hurlimann and Wilson (2018) (0.021); Krysanova et al. (2010) (0.018) | Climate change; adaptation; adaptive capacity; adaptation to climate change; barriers; interorganizational networks; water management organizations; water; spatial planning; urban; sustainable urban water management; water management; river basin; questionnaire; driver; Barrier; Amudarya; Elbe; Guadiana; Nile Equatorial Lakes region; Orange; Rhine |
2. CC adaptation strategies for urban flood mitigation and stormwater management and decision-making process | 22 | 2010/2022 | Kim et al. (2017) (0.015); Fratini et al. (2012) (0.014); Eakin et al. (2022) (0.013) | Climate change adaptation; urban flooding; adaptation; decision-making; social justice complexity theory; multifunctionality; transdisciplinary |
3. CC adaptation strategies in water management to achieve water security | 22 | 2000/2022 | Lebel et al. (2010) (0.017); Bonelli et al. (2014) (0.017); Martins Pereira et al. (2009) (0.015) | Climate change; Adaptation; Water security; Water; Water governance; Monsoon Asia; Transboundary rivers; Maipo basin; Santiago; Brazil; Institutions; River; Sao Joao |
4. CC adaptation strategies to achieve robustness in water resources management systems through river modeling and reservoir reoperation | 27 | 2008/2022 | Rheinheimer et al. (2016) (0.016); Sterle et al. (2020) (0.016); Wilby (2020) (0.015) | Climate change; adaptation; water resources management; snow-fed river basins; risk assessment; modeling; reservoir reoperation; hydropower; environmental flows; collaborative modeling; climate stationarity; adaptive water management; risk assessment; field experiment |
Statistical Characteristics
Although publications on CC adaptation in water management started appearing in 2000, they significantly evolved during the last decade (Fig. 4), indicating an increased interest in this topic.
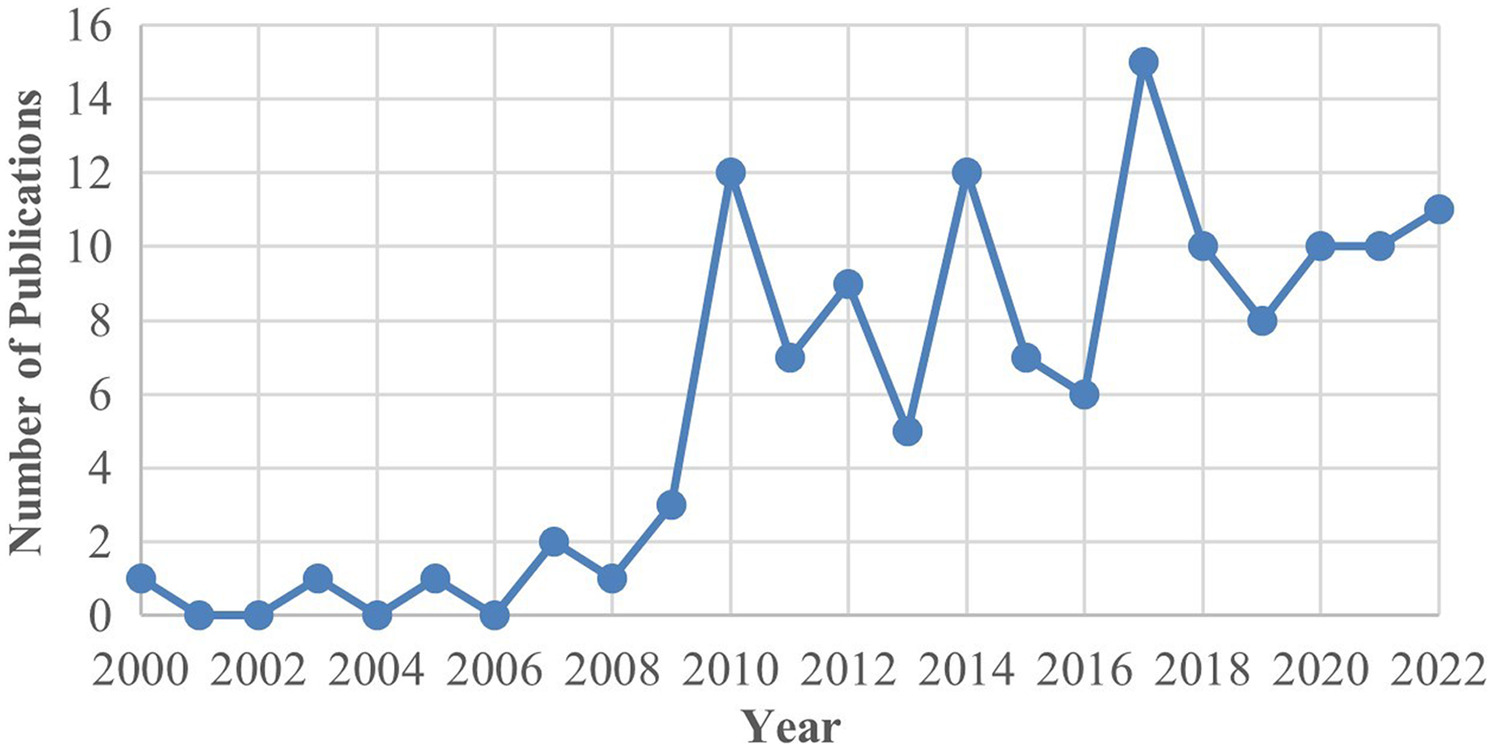
Identified Research Gaps and Suggested Future Studies
The following are research gaps identified based on the reviewed publications:
•
The WEAP model was commonly used for CC adaptation in water resources management systems due to its capability of integrating the primary system’s components (i.e., hydrologic processes, water resources allocation, water management, water infrastructure, and water demand). However, further investigations are required to reduce its long computational time and incorporate optimization routines to facilitate the calibration of its hydrologic model (Olabanji et al. 2020).
•
Although projections of water demand and LULC changes significantly impact the water management systems, they remain underincorporated in water management studies under CC. More than half (53%) did not include any of those two aspects; therefore, we highly recommend including them together in future studies. This recommendation is also supported by the Bonelli et al. (2014) findings.
•
Although they are crucial for evaluating the CC adaptation options, the economic, environmental, and social aspects remain underassessed individually and combined. Thus, they should be more commonly included in future studies, especially combined aspects, to ensure the sustainability of the selected strategies.
•
Updating the WYT definition as an adaptation strategy should be further investigated (Rheinheimer et al. 2016) using the approaches reported in the “Discussion” section of this review or developing new ones.
•
Bonelli et al. (2014) recommended including CC impacts on groundwater management in future research. Also, the literature lacks studies on CC adaptation in groundwater management, although groundwater is the main source for many people worldwide. Therefore, this topic is an urgent and important niche area of research.
•
The bibliometric analysis in this review is performed based on the methodology used in previous studies (e.g., Islam et al. 2021; Nasture et al. 2022; Chen et al. 2022), which did not include the academic tree impact that may result in research bias. However, its investigation is challenging because many researchers do not declare their academic relation. Therefore, we recommend considering the impact of the academic genealogy tree in future studies that focus on bibliometric analysis.
•
To reveal the influential publications and their research areas, we used the co-citation analysis technique for clustering in this review. However, if the objective is to identify a wide range of topics and allow niche and recent articles to gain visibility, Donthu et al. (2021) recommend bibliographic coupling, which clusters the articles based on the shared references.
Conclusions
This review comprehensively synthesizes the CC adaptation strategies and techniques in water management discussed in the English publications available on the Web of Science. It provides a typical procedure developed based on the reviewed articles to facilitate future relevant studies. This study categorizes the adaptation strategies discussed in the reviewed articles into strategies with and without modeling. The modeled strategies are classified into two groups: (1) water resources management strategies; and (2) storm drainage and flood mitigation ones.
For CC adaptation strategies in water resources management, adapting reservoir operation curves through optimization algorithms was the most used technique. It was preferred because it avoids/alleviates the cost of infrastructure construction or rehabilitation. However, water supply augmentation/conservation, demand management, and reuse strategies were also investigated. There was no agreement on one individual strategy to achieve the best performance in all the cases. Olabanji et al. (2020) recommended combining these strategies (i.e., water supply augmentation/conservation, demand management, and water reuse). Mirdashtvan et al. (2021) found that decreasing the population demand and water distribution network losses significantly improved adaptation to CC. Rheinheimer et al. (2016) reported that updating the WYT definition is effective for CC adaptation in water resources management systems and recommended them to be further investigated. WEAP is commonly used for modeling water resources management strategies. However, its long run time and inability to automatically calibrate the hydrologic model still need to be investigated (Olabanji et al. 2020).
Regarding CC adaptation in storm drainage and flood mitigation systems, LID measures were the most investigated. Their significant role in reducing flow runoff and flooding was evidenced by many studies (e.g., Binesh et al. 2019; Kirshen et al. 2018). However, other studies (e.g., Hopkins et al. 2020) reported that widely implementing LIDs did not prevent urbanization from altering streamflow magnitude and timing. Comparing LIDs to sewer enlargement (a gray strategy), Kourtis et al. (2021) found that the performance of each strategy was significantly sensitive to climate scenarios. When gray solutions are required, the environmental aspect should be included in evaluating the potential adaptation strategies. CC adaptation strategies in storm drainage and flood mitigation systems, in the reviewed studies, were commonly modeled using SWMMs.
A bibliometric analysis was performed in this review using co-citation analysis with the aid of BibExcel, Gephi, and VosViewer software packages. This analysis resulted in grouping the reviewed papers into four clusters, which are illustrated in Table 2. The research topic of each cluster was determined manually based on the keywords from the top three publications with the highest Page Rank scores. The analysis performed in the current review did not include the academic tree’s possible impact on the authors’ keywords selection. However, because of related challenges (e.g., identifying the academic tree while many researchers do not declare it), this point is recommended for future studies. The analysis also showed that CC adaptation in water management is still a relatively new topic that draws the attention of researchers.
Although the assessments of the economic, social, and environmental aspects are significant in reaching sustainable solutions (Kirshen et al. 2018), only a few of the reviewed publications included them to evaluate CC adaptation strategies. Some factors that should be considered to assess each aspect are the cost-benefit analysis for the economic aspect, the community’s socioeconomic and demographic factors for the social aspect, and the significance of improving water quality and preserving the ecosystem for the environmental aspect. MCDA and considering stakeholders’ input can be used to determine the scores of these qualitative aspects. This review also recommends that future studies examine CC adaptation in groundwater management, further investigate the bibliometric analysis performed here using different methods (e.g., clustering via bibliographic coupling), and more widely incorporate water demand and LULC changes. This comprehensive review provides up-to-date information available in the literature on CC adaptation strategies in water management, including research gaps and recommendations. It represents a good starting point for future research on CC adaptation in water management.
Declaration of Competing Interest
The authors declare that they have no known competing financial interests or personal relationships that could have appeared to influence the work reported in this paper.
Supplemental Materials
File (supplemental materials_jhyeff.heeng-6014 _elgendy.pdf)
- Download
- 491.06 KB
Data Availability Statement
Some or all data, models, or codes that support the findings of this study are available from the corresponding author upon reasonable request. BibExcel is available at https://homepage.univie.ac.at/juan.gorraiz/bibexcel/. Gephi is available at https://gephi.org/. VOSviewer is available at https://www.vosviewer.com/download.
Acknowledgments
The authors thank the anonymous reviewers for their valuable comments and suggestions. This research was supported by the Natural Science and Engineering Research Council (NSERC) of Canada, Grant NSERC Canadian FloodNet (NETGP-451456).
References
Anh, D. T., L. P. Hoang, M. D. Bui, and P. Rutschmann. 2019. “Modelling seasonal flows alteration in the Vietnamese Mekong Delta under upstream discharge changes, rainfall changes and sea level rise.” Int. J. River Basin Manage. 17 (4): 435–449. https://doi.org/10.1080/15715124.2018.1505735.
Azhoni, A., S. Jude, and I. Holman. 2018. “Adapting to climate change by water management organisations: Enablers and barriers.” J. Hydrol. 559 (Apr): 736–748. https://doi.org/10.1016/j.jhydrol.2018.02.047.
Baena-Ruiz, L., D. Pulido-Velazquez, A. J. Collados-Lara, A. Renau-Pruñonosa, I. Morell, J. Senent-Aparicio, and C. Llopis-Albert. 2020. “Summarizing the impacts of future potential global change scenarios on seawater intrusion at the aquifer scale.” Environ. Earth Sci. 79 (Mar): 1–13. https://doi.org/10.1007/s12665-020-8847-2.
Baláž, V., D. Dokupilová, and R. Filčák. 2021. “Participatory multi-criteria methods for adaptation to climate change.” Mitigation Adapt. Strategies Global Change 26 (4): 15. https://doi.org/10.1007/s11027-021-09955-4.
Barría, P., C. Chadwick, A. Ocampo-Melgar, M. Galleguillos, R. Garreaud, R. Díaz-Vasconcellos, D. Poblete, E. Rubio-Álvarez, and D. Poblete-Caballero. 2021. “Water management or megadrought: What caused the Chilean Aculeo Lake drying?” Reg. Environ. Change 21 (1): 1–15. https://doi.org/10.1007/s10113-021-01750-w.
Binesh, N., M. H. Niksokhan, A. Sarang, and W. Rauch. 2019. “Improving sustainability of urban drainage systems for climate change adaptation using best management practices: A case study of Tehran, Iran.” Hydrol. Sci. J. 64 (4): 381–404. https://doi.org/10.1080/02626667.2019.1585857.
Blondel, V. D., J. L. Guillaume, R. Lambiotte, and E. Lefebvre. 2008. “Fast unfolding of communities in large networks.” J. Stat. Mech: Theory Exp. 2008 (10): P10008. https://doi.org/10.1088/1742-5468/2008/10/P10008.
Bojovic, D., C. Giupponi, H. Klug, L. Morper-Busch, G. Cojocaru, and R. Schörghofer. 2018. “An online platform supporting the analysis of water adaptation measures in the Alps.” J. Environ. Plann. Manage. 61 (2): 214–229. https://doi.org/10.1080/09640568.2017.1301251.
Bonelli, S., S. Vicuña, F. J. Meza, J. Gironás, and J. Barton. 2014. “Incorporating climate change adaptation strategies in urban water supply planning: The case of central Chile.” J. Water Clim. Change 5 (3): 357–376. https://doi.org/10.2166/wcc.2014.037.
Brandes, U., D. Delling, M. Gaertler, R. Gorke, M. Hoefer, Z. Nikoloski, and D. Wagner. 2007. “On modularity clustering.” IEEE Trans. Knowl. Data Eng. 20 (2): 172–188. https://doi.org/10.1109/TKDE.2007.190689.
Brin, S., and L. Page. 1998. “The anatomy of a large-scale hypertextual web search engine.” Comput. Netw. ISDN Syst. 30 (1–7): 107–117. https://doi.org/10.1016/S0169-7552(98)00110-X.
Callaway, J. M., and J. W. Currie. 1985. Water resource systems and changes in climate and vegetation. DOE/ER-0236. Washington, DC: DOE.
Chang, L. H., C. T. Hunsaker, and J. D. Draves. 1992. “Recent research on effects of climate change on water resources 1.” JAWRA J. Am. Water Resour. Assoc. 28 (2): 273–286. https://doi.org/10.1111/j.1752-1688.1992.tb03993.x.
Chen, P. Y., C. P. Tung, and Y. H. Li. 2017. “Low impact development planning and adaptation decision-making under climate change for a community against pluvial flooding.” Water 9 (10): 756. https://doi.org/10.3390/w9100756.
Chen, Y., M. Lin, and D. Zhuang. 2022. “Wastewater treatment and emerging contaminants: Bibliometric analysis.” Chemosphere 297 (Jun): 133932. https://doi.org/10.1016/j.chemosphere.2022.133932.
CNCCP (China’s National Climate Change Programme). 2007. “Report of National Development and Reform Commission (NDRC).” Accessed July 20, 2015. https://ccchina.gov.cn/WebSite/CCChina/UpFile/File188.pdf.
Dettinger, M., J. Anderson, M. Anderson, L. R. Brown, D. Cayan, and E. Maurer. 2016. “Climate change and the Delta.” San Francisco Estuary Watershed Sci. 14 (3): 1–26. https://doi.org/10.15447/sfews.2016v14iss3art5.
Ding, Y., E. Yan, A. Frazho, and J. Caverlee. 2009. “PageRank for ranking authors in co-citation networks.” J. Am. Soc. Inf. Sci. Technol. 60 (11): 2229–2243. https://doi.org/10.1002/asi.21171.
Donthu, N., S. Kumar, D. Mukherjee, N. Pandey, and W. M. Lim. 2021. “How to conduct a bibliometric analysis: An overview and guidelines.” J. Bus. Res. 133 (Sep): 285–296. https://doi.org/10.1016/j.jbusres.2021.04.070.
Eakin, H. C., J. Parajuli, B. Hernandez Aguilar, and Y. Yogya. 2022. “Attending to the social-political dimensions of urban flooding in decision-support research: A synthesis of contemporary empirical cases.” Wiley Interdiscip. Rev. Clim. Change 13 (1): e743. https://doi.org/10.1002/wcc.743.
Ellegaard, O., and J. A. Wallin. 2015. “The bibliometric analysis of scholarly production: How great is the impact?” Scientometrics 105 (3): 1809–1831. https://doi.org/10.1007/s11192-015-1645-z.
EPA. 2016. “Climate change impacts by sector.” EPA. Accessed February 13, 2023. https://19january2017snapshot.epa.gov/climate-impacts/climate-change-impacts-sector_.html.
Escriva-Bou, A., M. Pulido-Velazquez, and D. Pulido-Velazquez. 2017. “Economic value of climate change adaptation strategies for water management in Spain’s Jucar Basin.” J. Water Resour. Plann. Manage. 143 (5): 04017005. https://doi.org/10.1061/(ASCE)WR.1943-5452.0000735.
Feng, G. L., and Y. P. Wu. 2016. “Signal of acceleration and physical mechanism of water cycle in Xinjiang, China.” PLoS One 11 (12): e0167387. https://doi.org/10.1371/journal.pone.0167387.
Fratini, C. F., M. Elle, M. B. Jensen, and P. S. Mikkelsen. 2012. “A conceptual framework for addressing complexity and unfolding transition dynamics when developing sustainable adaptation strategies in urban water management.” Water Sci. Technol. 66 (11): 2393–2401. https://doi.org/10.2166/wst.2012.442.
Fu, G., C. Zhang, J. W. Hall, and D. Butler. 2022. “Are sponge cities the solution to China’s growing urban flooding problems?” Wiley Interdiscip. Rev.: Water 10 (1): e1613. https://doi.org/10.1002/wat2.1613.
Füssel, H. M. 2007. “Adaptation planning for climate change: Concepts, assessment approaches, and key lessons.” Sustainability Sci. 2 (2): 265–275. https://doi.org/10.1007/s11625-007-0032-y.
Garnier, M., and I. Holman. 2019. “Critical review of adaptation measures to reduce the vulnerability of European drinking water resources to the pressures of climate change.” Environ. Manage. 64 (2): 138–153. https://doi.org/10.1007/s00267-019-01184-5.
Giuntoli, I., J. P. Vidal, C. Prudhomme, and D. M. Hannah. 2015. “Future hydrological extremes: The uncertainty from multiple global climate and global hydrological models.” Earth Syst. Dyn. 6 (1): 267–285. https://doi.org/10.5194/esd-6-267-2015.
Gutiérrez, J. M., et al. 2019. “An intercomparison of a large ensemble of statistical downscaling methods over Europe: Results from the value perfect predictor cross-validation experiment.” Int. J. Climatol. 39 (9): 3750–3785. https://doi.org/10.1002/joc.5462.
Hallegatte, S. 2010. “Uncertainties in the cost-benefit analysis of adaptation measures, and consequences for decision making.” In Climate: Global change and local adaptation, 169–192. Dordrecht, Netherlands: Springer.
Hernández-Hernández, M., J. Olcina, and Á. F. Morote. 2020. “Urban stormwater management, a tool for adapting to climate change: From risk to resource.” Water 12 (9): 2616. https://doi.org/10.3390/w12092616.
Heslehurst, N., H. Brown, A. Pemu, H. Coleman, and J. Rankin. 2018. “Perinatal health outcomes and care among asylum seekers and refugees: A systematic review of systematic reviews.” BMC Med. 16 (1): 1–25. https://doi.org/10.1186/s12916-018-1064-0.
Hopkins, K. G., A. S. Bhaskar, S. A. Woznicki, and R. M. Fanelli. 2020. “Changes in event-based streamflow magnitude and timing after suburban development with infiltration-based stormwater management.” Hydrol. Processes 34 (2): 387–403. https://doi.org/10.1002/hyp.13593.
Hurlimann, A., and E. Wilson. 2018. “Sustainable urban water management under a changing climate: The role of spatial planning.” Water 10 (5): 546. https://doi.org/10.3390/w10050546.
IPCC (Panel on Climate Change). 2007. “Climate change 2007.” In Synthesis report: Contribution of working groups I, II and III to the fourth assessment report of the intergovernmental panel on climate change, edited by R. K. Pachauri and A. Reisinger, 104. Geneva: IPCC.
IPCC (Panel on Climate Change). 2014. “Climate change 2014: Impacts, adaptation, and vulnerability. Part B: Regional aspects.” In Contribution of working group II to the fifth assessment report of the intergovernmental panel on climate change. Cambridge, UK: Cambridge University Press.
Islam, A., S. Hassini, and W. El-Dakhakhni. 2021. “A systematic bibliometric review of optimization and resilience within low impact development stormwater management practices.” J. Hydrol. 599 (Aug): 126457. https://doi.org/10.1016/j.jhydrol.2021.126457.
Jafroudi, M. 2020. “A legal obligation to adapt transboundary water agreements to climate change.” Water Policy 22 (5): 717–732. https://doi.org/10.2166/wp.2020.212.
Jaswal, A. K., A. Karandikar, M. Gujar, and S. Bhan. 2015. “Seasonal and annual rainfall trends in Himachal Pradesh during 1951–2005.” Mausam 66 (2): 247–264. https://doi.org/10.54302/mausam.v66i2.534.
Kahaduwa, A., and L. Rajapakse. 2022. “Review of climate change impacts on reservoir hydrology and long-term basin-wide water resources management.” Build. Res. Inf. 50 (5): 515–526. https://doi.org/10.1080/09613218.2021.1977908.
Khacheba, R., M. Cherfaoui, T. Hartani, and N. Drouiche. 2018. “The nexus approach to water-energy-food security: An option for adaptation to climate change in Algeria.” Desalin. Water Treat. 131 (Feb): 30–33. https://doi.org/10.5004/dwt.2018.22950.
Khaniya, B., M. B. Gunathilake, and U. Rathnayake. 2021. “Ecosystem-based adaptation for the impact of climate change and variation in the water management sector of Sri Lanka.” In Mathematical problems in engineering. London: Hindawi Limited.
Kim, Y., D. A. Eisenberg, E. N. Bondank, M. V. Chester, G. Mascaro, and B. S. Underwood. 2017. “Fail-safe and safe-to-fail adaptation: Decision-making for urban flooding under climate change.” Clim. Change 145 (3–4): 397–412. https://doi.org/10.1007/s10584-017-2090-1.
Kirshen, P., S. Aytur, J. Hecht, A. Walker, D. Burdick, S. Jones, N. Fennessey, R. Bourdeau, and L. Mather. 2018. “Integrated urban water management applied to adaptation to climate change.” Urban Clim. 24 (Jun): 247–263. https://doi.org/10.1016/j.uclim.2018.03.005.
Koch, H., M. Kaltofen, S. Kaden, and U. Grünewald. 2010. “Effects of global change and adaptation options for water resources management in the Czech part of the River Elbe basin (Vol. 340).” IAHS Publ. Accessed January 20, 2023. https://www.glowa-elbe.de.
Kourtis, I. M., V. Bellos, G. Kopsiaftis, B. Psiloglou, and V. A. Tsihrintzis. 2021. “Methodology for holistic assessment of grey-green flood mitigation measures for climate change adaptation in urban basins.” J. Hydrol. 603 (Dec): 126885. https://doi.org/10.1016/j.jhydrol.2021.126885.
Kourtis, I. M., and V. A. Tsihrintzis. 2021. “Adaptation of urban drainage networks to climate change: A review.” Sci. Total Environ. 771 (Jun): 145431. https://doi.org/10.1016/j.scitotenv.2021.145431.
Krysanova, V., et al. 2010. “Cross-comparison of climate change adaptation strategies across large river basins in Europe, Africa and Asia.” Water Resour. Manage. 24 (14): 4121–4160. https://doi.org/10.1007/s11269-010-9650-8.
Kundzewicz, Z. W., V. Krysanova, R. E. Benestad, Ø. Hov, M. Piniewski, and I. M. Otto. 2018. “Uncertainty in climate change impacts on water resources.” Environ. Sci. Policy 79 (Jan): 1–8. https://doi.org/10.1016/j.envsci.2017.10.008.
Lebel, L. 2013. “Local knowledge and adaptation to climate change in natural resource-based societies of the Asia-Pacific.” Mitigation Adapt. Strategies Global Change 18 (7): 1057–1076. https://doi.org/10.1007/s11027-012-9407-1.
Lebel, L., J. Xu, R. C. Bastakoti, and A. Lamba. 2010. “Pursuits of adaptiveness in the shared rivers of Monsoon Asia.” Int. Environ. Agreements-Polit. Law Econ. 10 (4): 355–375. https://doi.org/10.1007/s10784-010-9141-7.
Le Blanc, D. 2020. E-participation: A quick overview of recent qualitative trends, 163. New York: United Nations, Dept. of Economics and Social Affairs Working Papers.
Löwe, R., C. Urich, N. Domingo, O. Mark, A. Deletic, and K. Arnbjerg-Nielsen. 2017. “Assessment of urban pluvial flood risk and efficiency of adaptation options through simulations–A new generation of urban planning tools.” J. Hydrol. 550 (May): 355367. https://doi.org/10.1016/j.jhydrol.2017.05.009.
Ludwig, F., E. van Slobbe, and W. Cofino. 2014. “Climate change adaptation and integrated water resource management in the water sector.” J. Hydrol. 518 (Oct): 235–242. https://doi.org/10.1016/j.jhydrol.2013.08.010.
Manocha, N., and V. Babovic. 2018. “Real options, multi-objective optimization and the development of dynamically robust adaptive pathways.” Environ. Sci. Policy 90 (Dec): 11–18. https://doi.org/10.1016/j.envsci.2018.09.012.
Marchuk, G. I., V. P. Dymnikov, V. B. Zalesnyi, V. N. Lykosov, I. M. Bobyleva, V. I. Galin, and A. A. Fomenko. 1982. “Mathematical modeling of the general circulation of the atmosphere and ocean.” In Current problems in applied mathematics and mathematical modeling. A83-25238 09-46, 116–124. Novosibirsk, Russia: Izdatel’stvo Nauka.
Martins Pereira, L. F., S. Barreto, and J. Pittock. 2009. “Participatory river basin management in the Sao Joao River, Brazil: A basis for climate change adaptation?” Clim. Dev. 1 (3): 261–268. https://doi.org/10.3763/cdev.2009.0026.
Medellín-Azuara, J., J. J. Harou, M. A. Olivares, K. Madani, J. R. Lund, R. E. Howitt, S. K. Tanaka, M. W. Jenkins, and T. Zhu. 2008. “Adaptability and adaptations of California’s water supply system to dry climate warming.” Clim. Change 87 (Mar): 75–90. https://doi.org/10.1007/s10584-007-9355-z.
Mgquba, S. K., and S. Majozi. 2020. “Climate change and its impacts on hydro-politics in transboundary basins: A case study of the Orange-Senqu River basin.” J. Water Clim. Change 11 (1): 150–165. https://doi.org/10.2166/wcc.2018.166.
Mirdashtvan, M., A. Najafinejad, A. Malekian, and A. Sa’doddin. 2021. “Sustainable water supply and demand management in semi-arid regions: Optimizing water resources allocation based on RCPs scenarios.” Water Resour. Manage. 35 (15): 5307–5324. https://doi.org/10.1007/s11269-021-03004-0.
Momblanch, A., L. Beevers, P. Srinivasalu, A. Kulkarni, and I. P. Holman. 2020. “Enhancing production and flow of freshwater ecosystem services in a managed Himalayan river system under uncertain future climate.” Clim. Change 162 (2): 343–361. https://doi.org/10.1007/s10584-020-02795-2.
Nasture, A. M., E. I. Ionete, F. A. Lungu, S. I. Spiridon, and L. G. Patularu. 2022. “Water quality carbon nanotube-based sensors technological barriers and late research trends: A bibliometric analysis.” Chemosensors 10 (5): 161. https://doi.org/10.3390/chemosensors10050161.
Null, S. E., and J. H. Viers. 2013. “In bad waters: Water year classification in nonstationary climates.” Water Resour. Res. 49 (2): 1137–1148. https://doi.org/10.1002/wrcr.20097.
Olabanji, M. F., T. Ndarana, N. Davis, and E. Archer. 2020. “Climate change impact on water availability in the Olifants catchment (South Africa) with potential adaptation strategies.” Phys. Chem. Earth 120 (Dec): 102939. https://doi.org/10.1016/j.pce.2020.102939.
Onyutha, C., A. Asiimwe, B. Ayugi, H. Ngoma, V. Ongoma, and H. Tabari. 2021. “Observed and future precipitation and evapotranspiration in water management zones of Uganda: CMIP6 projections.” Atmosphere 12 (7): 887. https://doi.org/10.3390/atmos12070887.
Pandey, D. N., A. K. Gupta, and D. M. Anderson. 2003. “Rainwater harvesting as an adaptation to climate change.” In Current science, 46–59. Bangalore, India: Current Science Association.
Papadaskalopoulou, C., E. Katsou, K. Valta, K. Moustakas, D. Malamis, and M. Dodou. 2015. “Review and assessment of the adaptive capacity of the water sector in Cyprus against climate change impacts on water availability.” Resour. Conserv. Recycl. 105 (Mar): 95–112. https://doi.org/10.1016/j.resconrec.2015.10.017.
Papadimitriou, L., I. P. Holman, R. Dunford, and P. A. Harrison. 2019. “Trade-offs are unavoidable in multi-objective adaptation even in a post-Paris Agreement world.” Sci. Total Environ. 696 (Dec): 134027. https://doi.org/10.1016/j.scitotenv.2019.134027.
Quagliolo, C., P. Roebeling, R. Mendonça, A. Pezzoli, and E. Comino. 2022. “Integrating biophysical and economic assessment: Review of nature-based adaptation to urban flood extremes.” Urban Sci. 6 (3): 53. https://doi.org/10.3390/urbansci6030053.
Quandt, A., B. O’Shea, S. A. Oke, and O. O. Ololade. 2022. “Policy interventions to address water security impacted by climate change: Adaptation strategies of three case studies across different geographic regions.” Front. Water 4 (Oct): 935422. https://doi.org/10.3389/frwa.2022.935422.
Rankoana, S. A. 2020. “Climate change impacts on water resources in a rural community in Limpopo province, South Africa: A community-based adaptation to water insecurity.” Int. J. Clim. Change Strategies Manage. 12 (5): 587–598. https://doi.org/10.1108/IJCCSM-04-2020-0033.
Rehana, S., and P. P. Mujumdar. 2013. “Impacts of climate change on regional water resources.” J. Indian Inst. Sci. 93 (2): 227–242.
Rheinheimer, D. E., S. E. Null, and J. H. Viers. 2016. “Climate-adaptive water year typing for instream flow requirements in California’s Sierra Nevada.” J. Water Resour. Plann. Manage. 142 (11): 04016049. https://doi.org/10.1061/(ASCE)WR.1943-5452.0000693.
Rodrigues, M., and C. Antunes. 2021. “Best management practices for the transition to a water-sensitive city in the south of Portugal.” Sustainability 13 (5): 2983. https://doi.org/10.3390/su13052983.
Sampson, D. A., E. M. Cook, M. J. Davidson, N. B. Grimm, and D. M. Iwaniec. 2020. “Simulating alternative sustainable water futures.” Sustainability Sci. 15 (4): 1199–1210. https://doi.org/10.1007/s11625-020-00820-y.
Sant’Anna, C., A. Tilmant, and M. Pulido-Velazquez. 2022. “A hydrologically-driven approach to climate change adaptation for multipurpose multireservoir systems.” Clim. Risk Manage. 36 (Jan): 100427. https://doi.org/10.1016/j.crm.2022.100427.
Saraswat, C., P. Kumar, and B. K. Mishra. 2016. “Assessment of stormwater runoff management practices and governance under climate change and urbanization: An analysis of Bangkok, Hanoi and Tokyo.” Environ. Sci. Policy 64 (Oct): 101–117. https://doi.org/10.1016/j.envsci.2016.06.018.
Schuster, Z. T., K. W. Potter, and D. S. Liebl. 2012. “Assessing the effects of climate change on precipitation and flood damage in Wisconsin.” J. Hydrol. Eng. 17 (8): 888–894. https://doi.org/10.1061/(ASCE)HE.1943-5584.0000513.
Schwalm, C. R., S. Glendon, and P. B. Duffy. 2020. “RCP8.5 tracks cumulative emissions.” Proc. Natl. Acad. Sci. U.S.A. 117 (33): 19656–19657. https://doi.org/10.1073/pnas.2007117117.
Shaffril, H. A. M., A. A. Samah, and S. F. Samsuddin. 2021. “Guidelines for developing a systematic literature review for studies related to climate change adaptation.” Environ. Sci. Pollut. Res. 28 (18): 22265–22277. https://doi.org/10.1007/s11356-021-13178-0.
Shao, W., X. Su, J. Lu, J. Liu, Z. Yang, C. Mei, C. Liu, and J. Lu. 2021. “Urban resilience of Shenzhen city under climate change.” Atmosphere 12 (5): 537. https://doi.org/10.3390/atmos12050537.
Shrestha, S., M. S. Babel, and V. P. Pandey. 2014. Climate change and water resources. Boca Raton, FL: CRC Press.
Simonet, G., and S. Fatorić. 2016. “Does ‘adaptation to climate change’ mean resignation or opportunity?” Reg. Environ. Change 16 (3): 789–799. https://doi.org/10.1007/s10113-015-0792-3.
Singh, B. 1987. The impacts of -induced climate change on hydro-electric generation potential in the James Bay territory of Quebec. Vancouver, BC, Canada: Université de Montréal.
Singh, S., R. Kumar, A. Bhardwaj, L. Sam, M. Shekhar, A. Singh, R. Kumar, and A. Gupta. 2016. “Changing climate and glacio-hydrology in Indian Himalayan Region: A review.” Wiley Interdiscip. Rev. Clim. Change 7 (3): 393–410. https://doi.org/10.1002/wcc.393.
Singh, V. P., A. K. Mishra, H. Chowdhary, and C. P. Khedun. 2014. “Climate change and its impact on water resources.” In Modern water resources engineering, 525–569. Totowa, NJ: Humana Press. https://doi.org/10.1007/978-1-62703-595-8_11.
Stagge, J. H., and G. E. Moglen. 2014. “Evolutionary algorithm optimization of a multireservoir system with long lag times.” J. Hydrol. Eng. 19 (9): 05014011. https://doi.org/10.1061/(ASCE)HE.1943-5584.0000972.
Stagge, J. H., and G. E. Moglen. 2017. “Water resources adaptation to climate and demand change in the Potomac River.” J. Hydrol. Eng. 22 (11): 04017050. https://doi.org/10.1061/(ASCE)HE.1943-5584.0001579.
Steinschneider, S., and C. Brown. 2012. “Dynamic reservoir management with real-option risk hedging as a robust adaptation to nonstationary climate.” Water Resour. Res. 48 (5): 1–16. https://doi.org/10.1029/2011WR011540.
Sterle, K., L. Jose, S. Coors, L. Singletary, G. Pohll, and S. Rajagopal. 2020. “Collaboratively modeling reservoir reoperation to adapt to earlier snowmelt runoff.” J. Water Resour. Plann. Manage. 146 (1): 05019021. https://doi.org/10.1061/(ASCE)WR.1943-5452.0001136.
Timmerman, J., J. Matthews, S. Koeppel, D. Valensuela, and N. Vlaanderen. 2017. “Improving governance in transboundary cooperation in water and climate change adaptation.” Water Policy 19 (6): 1014–1029. https://doi.org/10.2166/wp.2017.156.
Titus, J. G., C. Y. Kuo, M. J. Gibbs, T. B. LaRoche, M. K. Webb, and J. O. Waddell. 1987. “Greenhouse effect, sea level rise, and coastal drainage systems.” J. Water Resour. Plann. Manage. 113 (2): 216–227. https://doi.org/10.1061/(ASCE)0733-9496(1987)113:2(216).
Upgupta, S., J. Sharma, M. Jayaraman, V. Kumar, and N. H. Ravindranath. 2015. “Climate change impact and vulnerability assessment of forests in the Indian Western Himalayan region: A case study of Himachal Pradesh, India.” Clim. Risk Manage. 10 (Jan): 63–76. https://doi.org/10.1016/j.crm.2015.08.002.
Urich, C., and W. Rauch. 2014. “Exploring critical pathways for urban water management to identify robust strategies under deep uncertainties.” Water Res. 66 (May): 374–389. https://doi.org/10.1016/j.watres.2014.08.020.
Wang, M., D. Q. Zhang, J. Su, A. P. Trzcinski, J. W. Dong, and S. K. Tan. 2017. “Future scenarios modeling of urban stormwater management response to impacts of climate change and urbanization.” Clean–Soil Air Water 45 (10): 1700111. https://doi.org/10.1002/clen.201700111.
Wilby, R. L. 2020. “Resilience viewed through the lens of climate change and water management.” Water 12 (9): 2510. https://doi.org/10.3390/w12092510.
Xiao-Jun, W., Z. Jian-Yun, S. Shahid, X. Xing-Hui, H. Rui-Min, and S. Man-Ting. 2014. “Catastrophe theory to assess water security and adaptation strategy in the context of environmental change.” Mitigation Adapt. Strategies Global Change 19 (4): 463–477. https://doi.org/10.1007/s11027-012-9443-x.
Xie, K., et al. 2022. “Intelligent scheduling of urban drainage systems: Effective local adaptation strategies for increased climate variability.” Water Resour. Manage. 37 (1): 91–111. https://doi.org/10.1007/s11269-022-03357-0.
Yang, T. H., and W. C. Liu. 2020. “A general overview of the risk-reduction strategies for floods and droughts.” Sustainability 12 (7): 1–20. https://doi.org/10.3390/su12072687.
Yang, Y. C. E., C. Brown, W. Yu, J. Wescoat, and C. Ringler. 2014. “Water governance and adaptation to climate change in the Indus River Basin.” J. Hydrol. 519 (Nov): 2527–2537. https://doi.org/10.1016/j.jhydrol.2014.08.055.
Zhang, X. 2015. “Conjunctive surface water and groundwater management under climate change.” Front. Environ. Sci. 3 (Sep): 59. https://doi.org/10.3389/fenvs.2015.00059.
Zhou, Q., T. E. Panduro, B. J. Thorsen, and K. Arnbjerg-Nielsen. 2013. “Adaption to extreme rainfall with open urban drainage system: An integrated hydrological cost-benefit analysis.” Environ. Manage. 51 (Mar): 586–601. https://doi.org/10.1007/s00267-012-0010-8.
Information & Authors
Information
Published In
Copyright
This work is made available under the terms of the Creative Commons Attribution 4.0 International license, https://creativecommons.org/licenses/by/4.0/.
History
Published online: Nov 9, 2023
Published in print: Feb 1, 2024
Discussion open until: Apr 9, 2024
Authors
Metrics & Citations
Metrics
Citations
Download citation
If you have the appropriate software installed, you can download article citation data to the citation manager of your choice. Simply select your manager software from the list below and click Download.